Rule-based shields embedded safe reinforcement learning approach for electric vehicle charging control
INTERNATIONAL JOURNAL OF ELECTRICAL POWER & ENERGY SYSTEMS(2024)
摘要
The optimal and secure electric vehicles charging control can be formulated as a large-scale partially-observable constrained Markov decision process (PO-CMDP) problem with high levels of security risks and uncertainties. Such a problem is very difficult to be handled by optimization-theory-based methods. Data-driven methods, especially reinforcement learning (RL), are suitable for handling uncertainties but are poor in ensuring safety, which is unacceptable in power systems. Motivated by the fact that humans could be supervised by an expert when learning a new skill involving risk, this paper proposes a safe RL framework that embeds rule-based local and global shields in the loop of RL for supervising the actions of agents. The proposed framework not only strictly guarantees local and global security in the training and execution phases, but also helps the agent to find a more near-optimal policy. The effectiveness and efficiency are demonstrated by comparison with multiple baseline methods in the IEEE-33 node system.
更多查看译文
关键词
Reinforcement learning,Safe reinforcement learning,Electric vehicle,Distribution system,Vehicle-to-grid
AI 理解论文
溯源树
样例
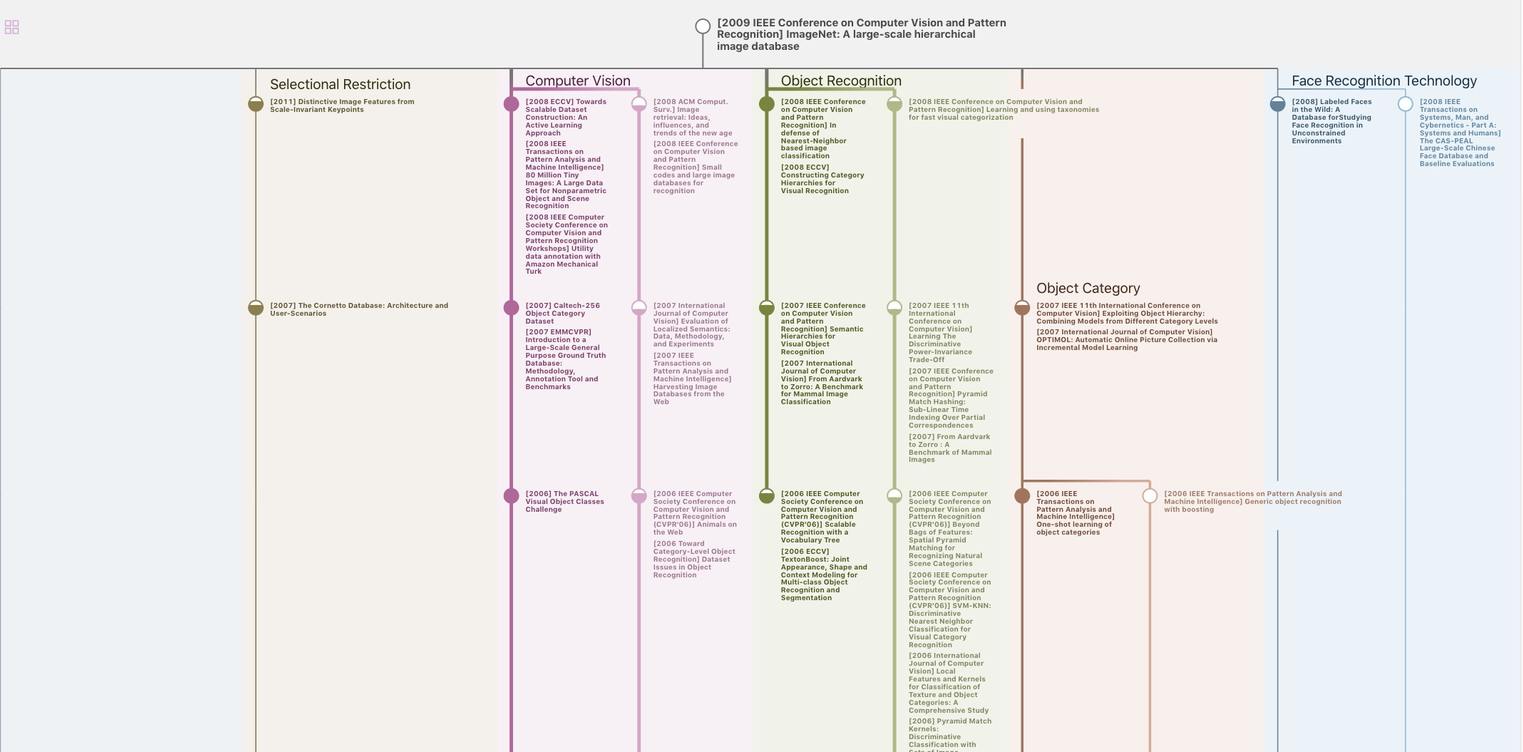
生成溯源树,研究论文发展脉络
Chat Paper
正在生成论文摘要