COVID-19 detection in lung CT slices using Brownian-butterfly-algorithm optimized lightweight deep features
HELIYON(2024)
摘要
Several deep-learning assisted disease assessment schemes (DAS) have been proposed to enhance accurate detection of COVID-19, a critical medical emergency, through the analysis of clinical data. Lung imaging, particularly from CT scans, plays a pivotal role in identifying and assessing the severity of COVID-19 infections. Existing automated methods leveraging deep learning contribute significantly to reducing the diagnostic burden associated with this process. This research aims in developing a simple DAS for COVID-19 detection using the pre-trained lightweight deep learning methods (LDMs) applied to lung CT slices. The use of LDMs contributes to a less complex yet highly accurate detection system. The key stages of the developed DAS include image collection and initial processing using Shannon's thresholding, deep-feature mining supported by LDMs, feature optimization utilizing the Brownian Butterfly Algorithm (BBA), and binary classification through three-fold cross-validation. The performance evaluation of the proposed scheme involves assessing individual, fused, and ensemble features. The investigation reveals that the developed DAS achieves a detection accuracy of 93.80% with individual features, 96% accuracy with fused features, and an impressive 99.10% accuracy with ensemble features. These outcomes affirm the effectiveness of the proposed scheme in significantly enhancing COVID-19 detection accuracy in the chosen lung CT database.
更多查看译文
关键词
COVID-19,Shannon ' s,MobileNet,Butterfly algorithm,Classification
AI 理解论文
溯源树
样例
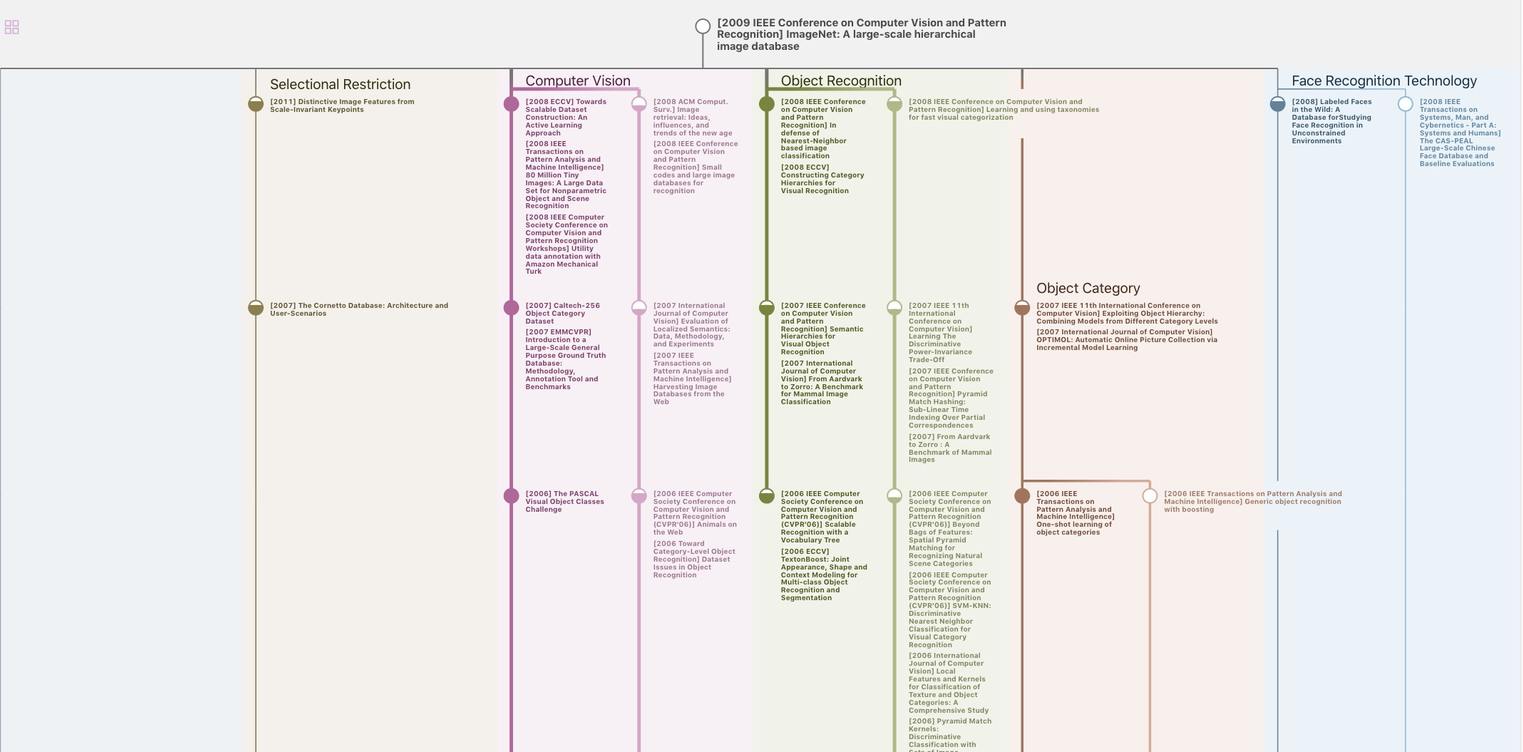
生成溯源树,研究论文发展脉络
Chat Paper
正在生成论文摘要