Improving phase change heat transfer in an enclosure filled by uniform and heterogenous metal foam layers: A neural network design approach
JOURNAL OF ENERGY STORAGE(2024)
摘要
Phase change materials (PCMs) inherently store and release large amounts of energy during phase transitions. In this research, the potential of two metal foam (MF) layers in enhancing the thermal energy storage unit's heat transfer was probed, with one layer having distinct attributes at an anisotropic angle, omega Utilizing the finite element method to understand the system dynamics, model accuracy was affirmed through rigorous checks. The impact of the heterogeneous parameter (0 < Kn < 0.3), heterogeneous angle (0 < omega < 90 degrees), and porosity 0.9 < epsilon < 0.975 was addressed on the melting process. To circumvent the high simulation costs, an artificial neural network (ANN) was trained on 7838 data points. Noteworthy findings indicate that a slight 7.5 % increase in porosity can reduce the melting time by 66 %. Moreover, the 0 degrees anisotropic angle emerged as the most efficient in heat transfer due to its superior thermal properties. The incorporation of ANN analytics was a pivotal shift, bypassing the traditionally high computational demands of phase change heat transfer studies. Once fully trained, the ANN adeptly demonstrated melting volume fraction (MVF) nuances under varied conditions. Further, optimal melting efficiencies were pinpointed at the omega = 0 degrees angle, with a specific porosity zone, epsilon similar to 0.925, showing minimal MVF and the benefits of a higher porosity (epsilon = 0.94) becoming evident at t = 3000 s. Ultimately, this investigation harmoniously integrates traditional analytical tools with ANN technology, offering profound insights into PCM heat transfer dynamics and laying the groundwork for future energy-efficient thermal storage solutions.
更多查看译文
关键词
Heterogeneous metal foam,Neural networks,Phase change material (PCM),Latent heat thermal energy storage (LHTES),Melting process
AI 理解论文
溯源树
样例
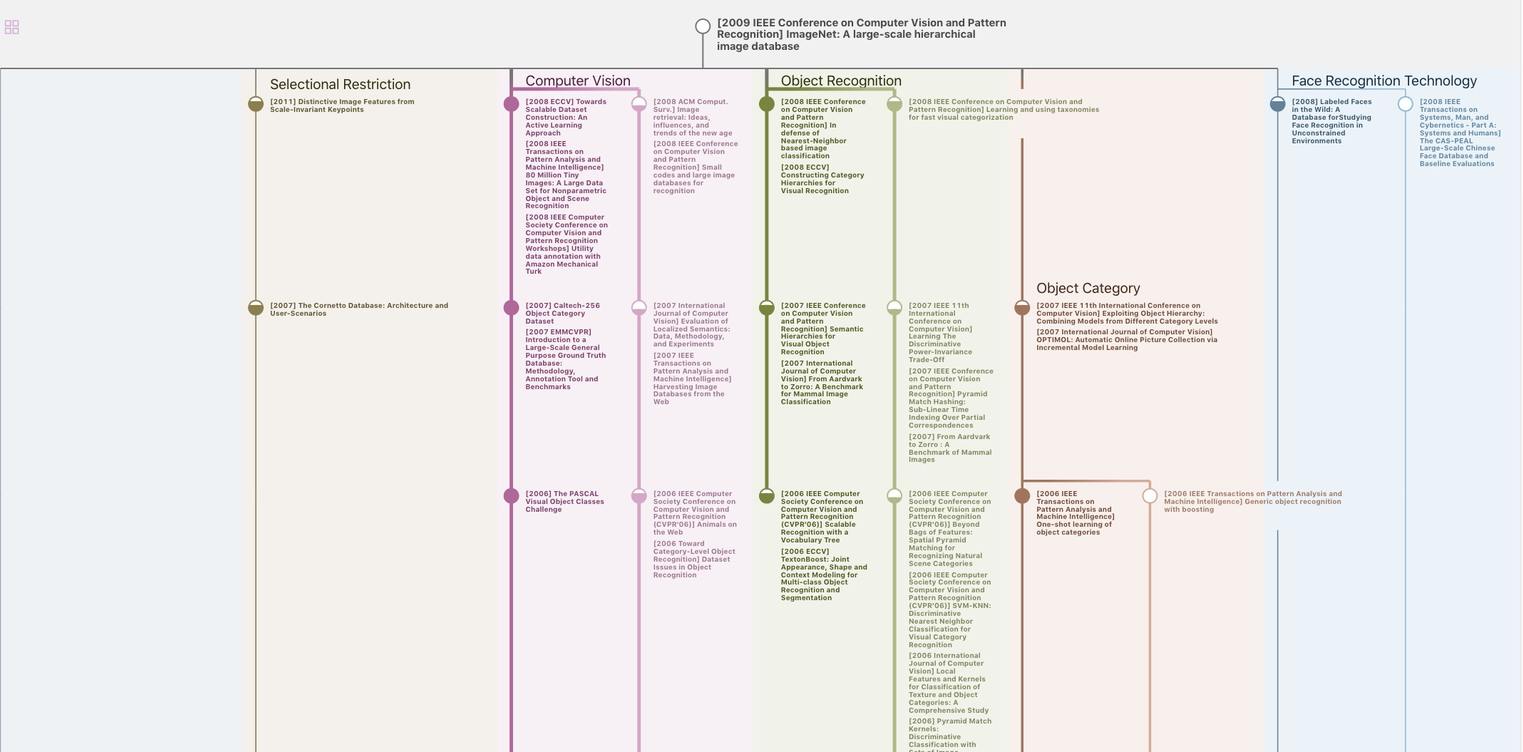
生成溯源树,研究论文发展脉络
Chat Paper
正在生成论文摘要