The coagulation-precipitation turbidity prediction model for precision drug delivery system based on deep learning and machine vision
JOURNAL OF ENVIRONMENTAL CHEMICAL ENGINEERING(2024)
摘要
The process effect of coagulation and precipitation is an important factor affecting the quality and economic cost of wastewater treatment, but it is very difficult to automatically control the dosage due to its nonlinear and large lag characteristics. Model prediction method will be the main direction to deal with nonlinearity and lag. In the process of coagulation and precipitation, the morphological characteristics of the floc reflect the quality of the flocculation, which has a great influence on the effluent quality. However, many modeling studies only use the water quality parameters of the previous moment to predict the effluent quality of the current moment, without considering the continuity of the flocculation precipitation process and the information represented by the floc image. The purpose of this paper is to solve the problem of precise dosing during coagulation and precipitation, save dosing cost. In this study, a self-built continuous coagulation and precipitation experimental system based on machine vision was used to conduct experiments and collect data, a self-written image processing program was used to quantitatively analyze the flocculation effect. LSTM model and Transformer model were used for training. The results showed that the deep learning model using time series data and flocs feature parameters had higher prediction accuracy, and both models achieved more accurate prediction (R-2>0.95). The results of this study provide a new direction for the model predictive control of coagulation-precipitation process and precise dosing.
更多查看译文
关键词
Coagulation precipitation,Deep learning,Neural network,Image processing,Turbidity prediction
AI 理解论文
溯源树
样例
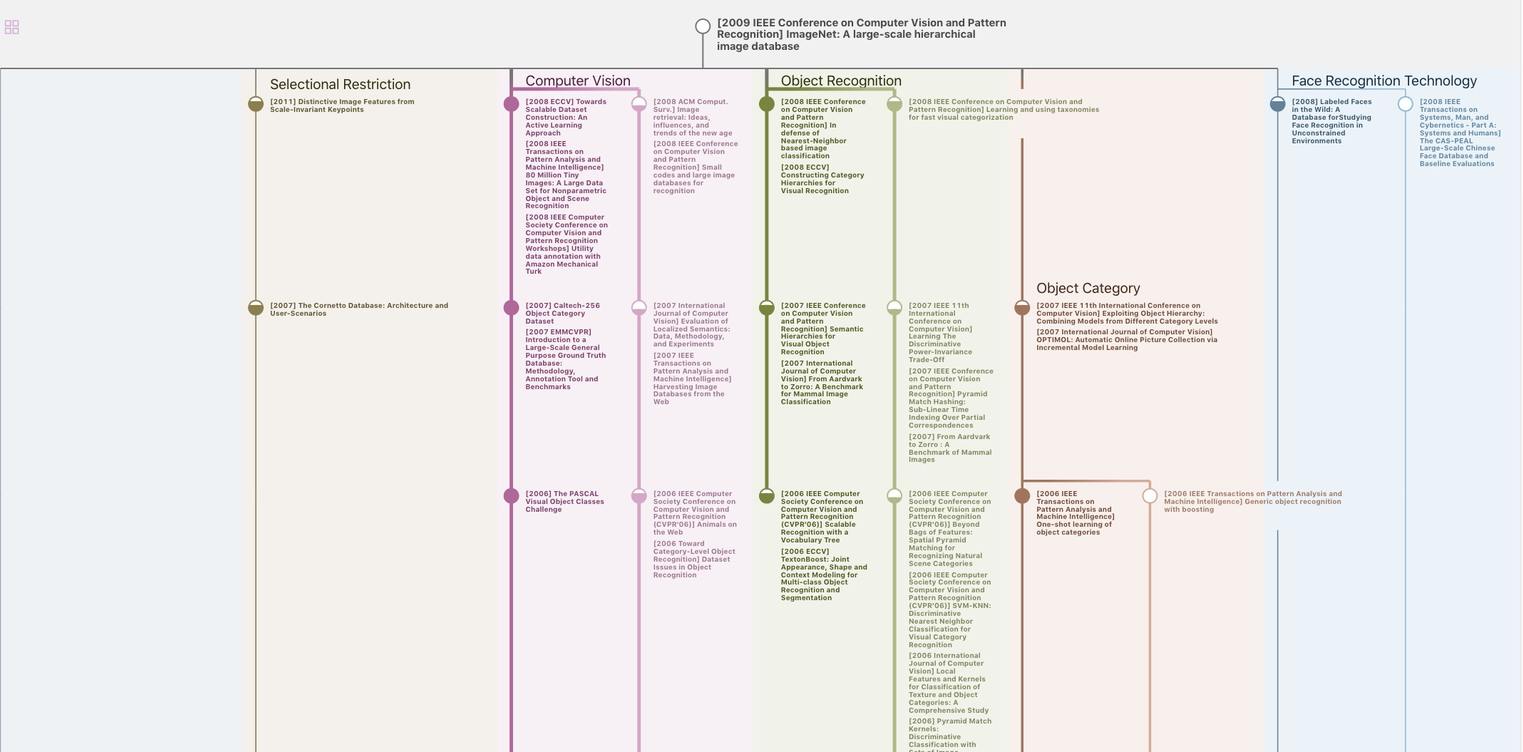
生成溯源树,研究论文发展脉络
Chat Paper
正在生成论文摘要