Time-varying polynomial grey prediction modeling with integral matching
KNOWLEDGE-BASED SYSTEMS(2024)
摘要
Owing to the variable characteristics of system structures, traditional grey prediction models with fixed parameters often struggle to accurately capture real -world dynamic changes. In this paper, we propose a novel time -varying polynomial grey model (TPGM) to address this issue. The TPGM leverages polynomial functions to approximate time -varying parameters, enabling it to capture trend variations effectively. Through integral matching, TPGM is reduced to an equivalent integro-differential equation (ITPGM) that allows direct modeling of the original time series. Then, the integral transformation and least -squares are employed to estimate model parameters, and a data -driven algorithm based on subset selection is designed to adaptively identify the optimal model structure. Extensive Monte Carlo simulations are conducted to evaluate model performance, and the results show that the ITPGM model has higher forecasting accuracy and robustness. Finally, two real -world cases of supply chain forecasting demonstrate that our model outperforms state-of-the-art forecasting methods.
更多查看译文
关键词
Grey prediction model,Time-varying parameter,Polynomial function,Integral matching,Structure identification
AI 理解论文
溯源树
样例
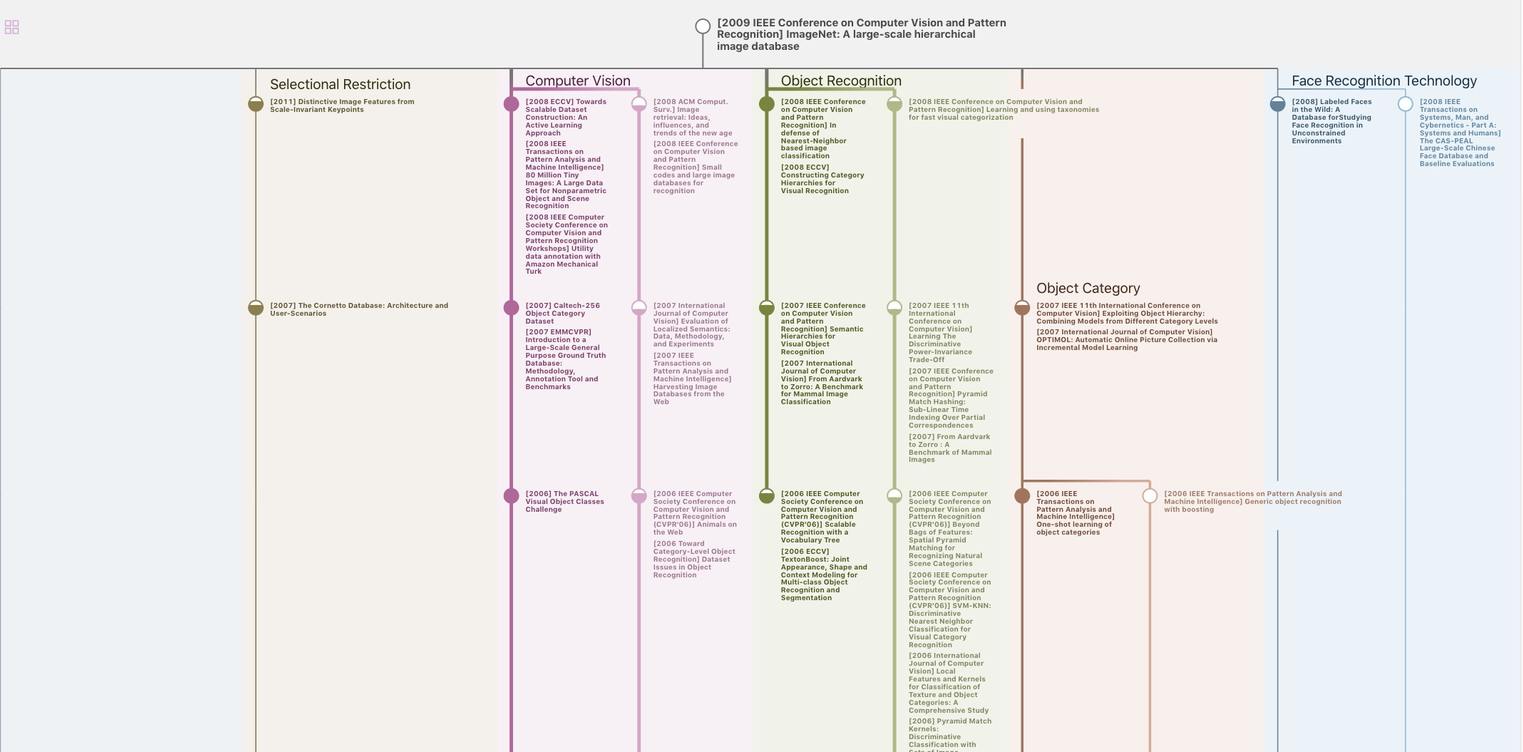
生成溯源树,研究论文发展脉络
Chat Paper
正在生成论文摘要