Chemical composition based machine learning model to predict defect formation in additive manufacturing
MATERIALIA(2024)
摘要
With a goal of exploiting additive manufacturing to improve the manufacturing of existing reactor materials, we developed a chemical composition-based machine learning model to predict the printability of any given alloy in laser powder bed fusion (L-PBF) using experimental data from peer-reviewed literature. We defined printability as the ability to avoid defects like cracking, balling, porosity, and lack of fusion, that are caused by thermal stresses (during solidification or liquation), molten pool disintegration into disconnected small beads or lack of heat input respectively. Our models predict the tendency of balling defect formation and porosity percentage for a given composition, under a given set of processing conditions. To predict the likelihood of balling defect, three models: a random forest classifier, a gradient boost regressor and a neural network were trained on a dataset containing both single and multi principal element alloys. The neural network model showed the highest accuracy of 92.3 % in predicting the balling defect formation. A random forest regressor, gradient boost regressor and neural network were trained and tested on a dataset of various alloys to predict porosity. The random forest regressor showed the best predictions with an R2 score of 0.97. The models also revealed the relative importance of the input descriptors on defect-formation tendency. Of particular significance was the identification of carbon as an important element in determining the occurrence of balling and percent porosity in alloys like steel, as well as being moderately important to the percentage porosity in other alloys as well as steel. Manganese was also identified as a key descriptor for the percentage of porosity in steel and other alloys. Manganese's low thermal conductivity and consistent presence in the dataset is the likely cause for its contribution. Carbon's role is attributable to its relatively high specific heat and high melting temperature. Our model serves as a swift, chemistry-based tool to design experiments and find modified compositions better suited for additive manufacturing.
更多查看译文
关键词
Additive manufacturing,Chemical composition,Laser powder bed fusion,Printability,Machine learning,Defects
AI 理解论文
溯源树
样例
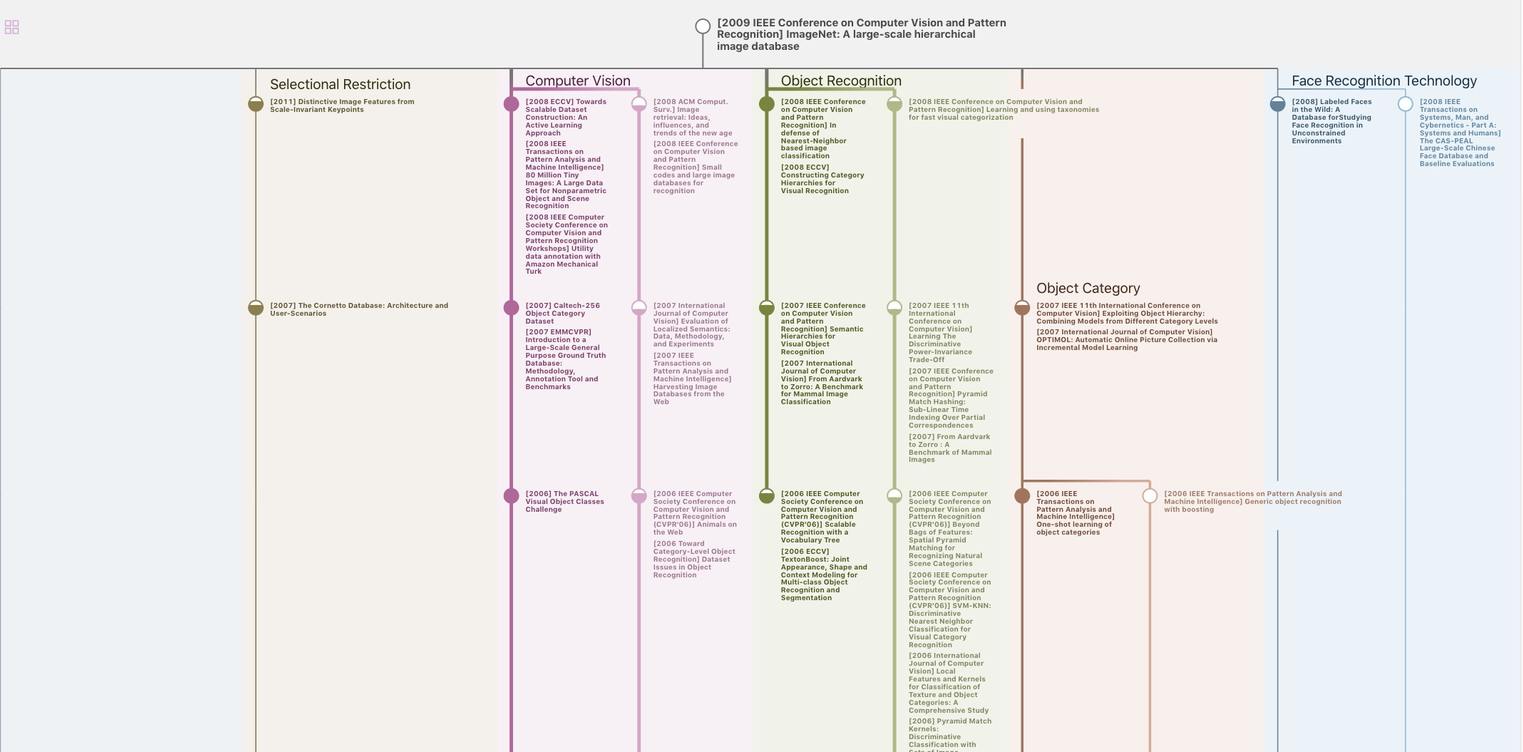
生成溯源树,研究论文发展脉络
Chat Paper
正在生成论文摘要