Abnormal data detection and recovery of sensors network based on spatiotemporal deep learning methodology
Measurement(2024)
摘要
This paper proposes a novel deep-learning-enabled framework for abnormal data detection and recovery considering spatial-temporal correlation among sensors. The wavelet scattering transform is adopted to replace the first layer of one-dimension convolutional neural network classification model. The multiple typical sensor failure modes of sensor are identified including bias, drifting, gain, precision degradation, and three complete faults (constant; constant + noise; noise). The bidirectional long-short time memory (BLSTM) network is developed to recover abnormal data through positive and negative propagation. The BLSTM cell involving forget, input, and output gate is utilized to adaptively transmit valuable information of long-term data. Different sensor configurations are conducted to select the optimal input sensors of BLSTM model. The proposed models are then validated by three engineering cases. The results show that the failure mode diagnosis and recovery accuracy are over 90 %. Sensor selection has a large impact on the data reconstruction, and selecting sensors with higher correlation can achieve a higher accuracy. In addition, the data recovery accuracy of the proposed BLSTM model surpasses that of traditional long-short time memory models.
更多查看译文
关键词
Sensor failure mode,Spatiotemporal correlation,WST-1DCNN,BLSTM,Data recovery
AI 理解论文
溯源树
样例
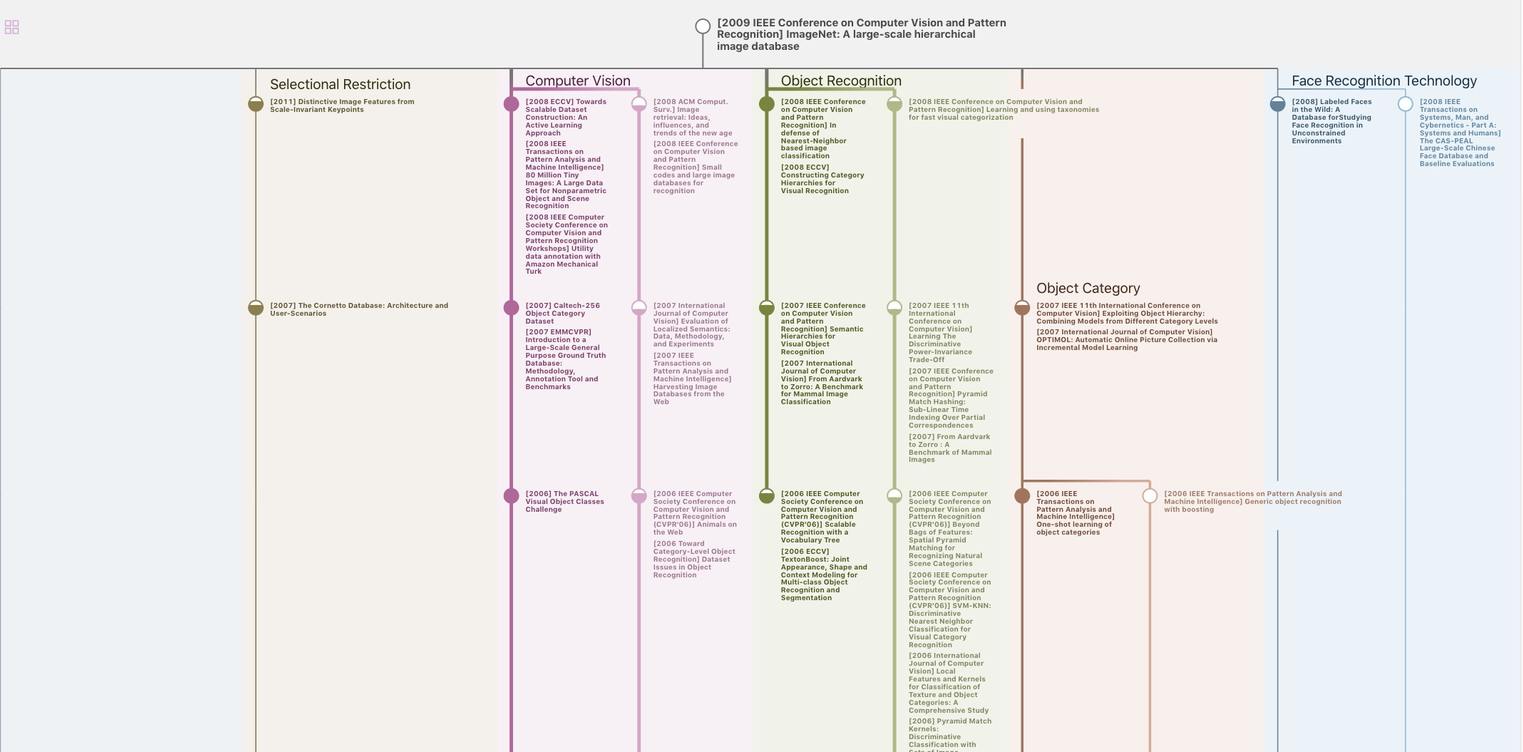
生成溯源树,研究论文发展脉络
Chat Paper
正在生成论文摘要