Domain adaptation for structural health monitoring via physics-informed and self-attention-enhanced generative adversarial learning
MECHANICAL SYSTEMS AND SIGNAL PROCESSING(2024)
摘要
Health monitoring technologies, empowered by sensor-driven information and model updating, play an important role in assessing the status of civil structures and detecting anomalies. However, significant domain discrepancies exist in the distribution of physical parameters between numerical structural models and their real-world counterparts. As a result, many health monitoring theories struggle to be effective in practice, even if they perform perfectly in the simulated data. To bridge the domain discrepancies, this paper proposes an unsupervised domain adaptation approach based on an adapted cycle-consistent generative adversarial network (CycleGAN) that incorporates physical constraints and a self-attention mechanism. The approach focuses on the mutual transformation of the multi-channel time series obtained from numerical models and actual structures. Specifically, the physical constraints are derived from the governing equation of linear dynamic systems, while the self-attention mechanism is achieved by adding transformer structures to both the generator and discriminator. Through free-vibration experiments on a steel beam and a large-scale steel bridge model, the physical constraints and transformer structures have proven beneficial for improving the learning capability and training stability of the GAN model. Furthermore, the proposed approach is not only verified as effective in transforming acceleration responses between the test structures and their corresponding finite element models in both time and frequency domains but has also been shown to reproduce mode shapes accurately.
更多查看译文
关键词
Structural health monitoring,Domain adaptation,Self -attention,Physical constraint,Generative adversarial learning
AI 理解论文
溯源树
样例
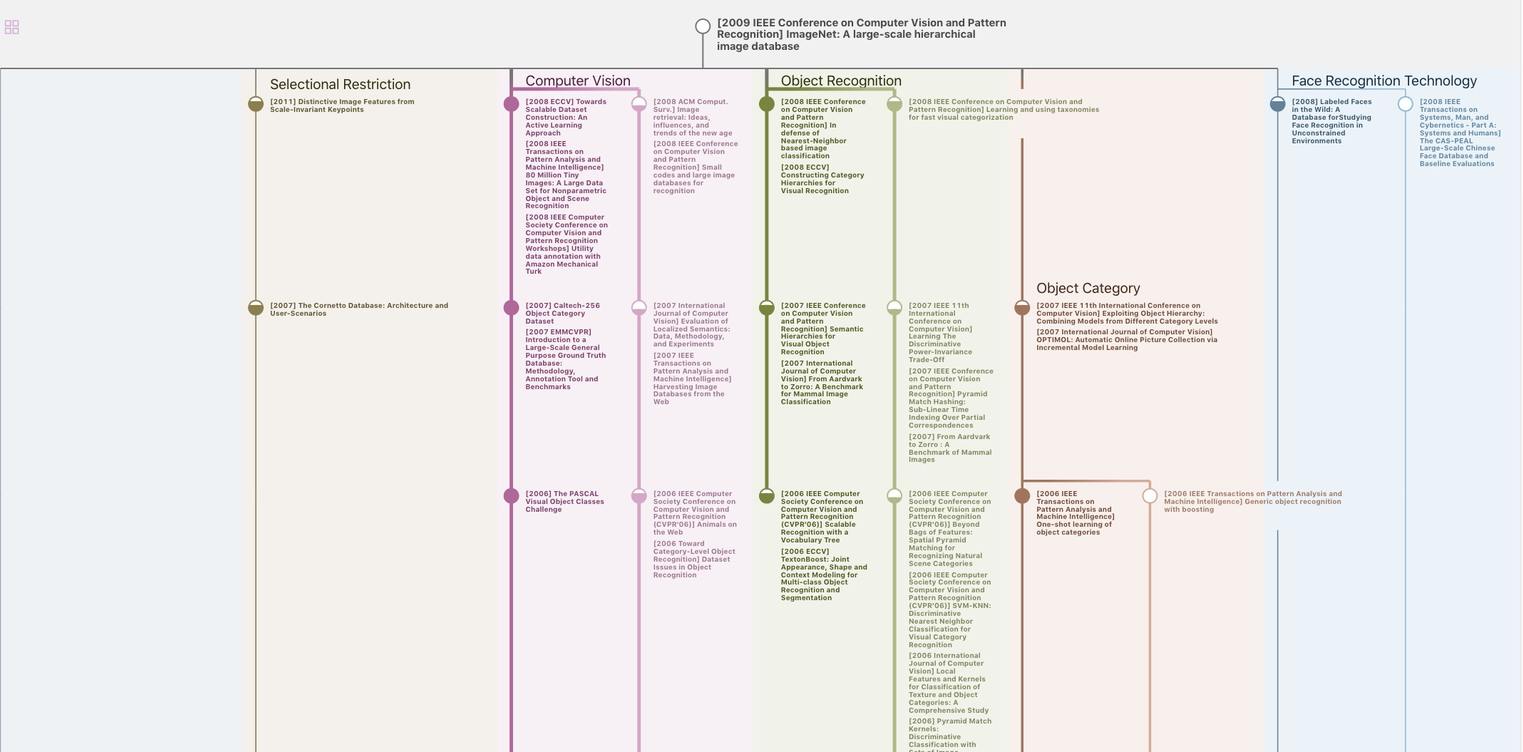
生成溯源树,研究论文发展脉络
Chat Paper
正在生成论文摘要