Regularized hidden Markov modeling with applications to wind speed predictions in offshore wind
MECHANICAL SYSTEMS AND SIGNAL PROCESSING(2024)
摘要
Offshore wind power is rapidly becoming an essential component of the transition to clean energy. As turbine design capacities continue to increase, there is a growing interest in monitoring both individual turbines and entire wind farms to ensure their performance while also reducing the levelized cost of energy. However, obtaining reliable and comprehensive data on these structures can be challenging, as it often requires costly and potentially dangerous installation procedures and significant computational resources. Therefore, it is critical to predict the needed information to properly assess the performance of offshore wind turbines when not available. To address these challenges, this paper introduces a modified Hidden Markov Model (HMM) framework -based strategy and a companion Python library, Hela. The proposed HMM-based framework incorporates a "smart" initialization strategy and regularization to overcome some of the limitations of applying HMMs to experimental cases. This Python library introduced in this paper is a highly flexible and customizable HMM library for training and inference. This work is all carried out within the use -drive context of offshore wind.
更多查看译文
关键词
Hidden Markov models,Wind speed,Offshore wind,Tykhonov Regularization,Cross-validation learning
AI 理解论文
溯源树
样例
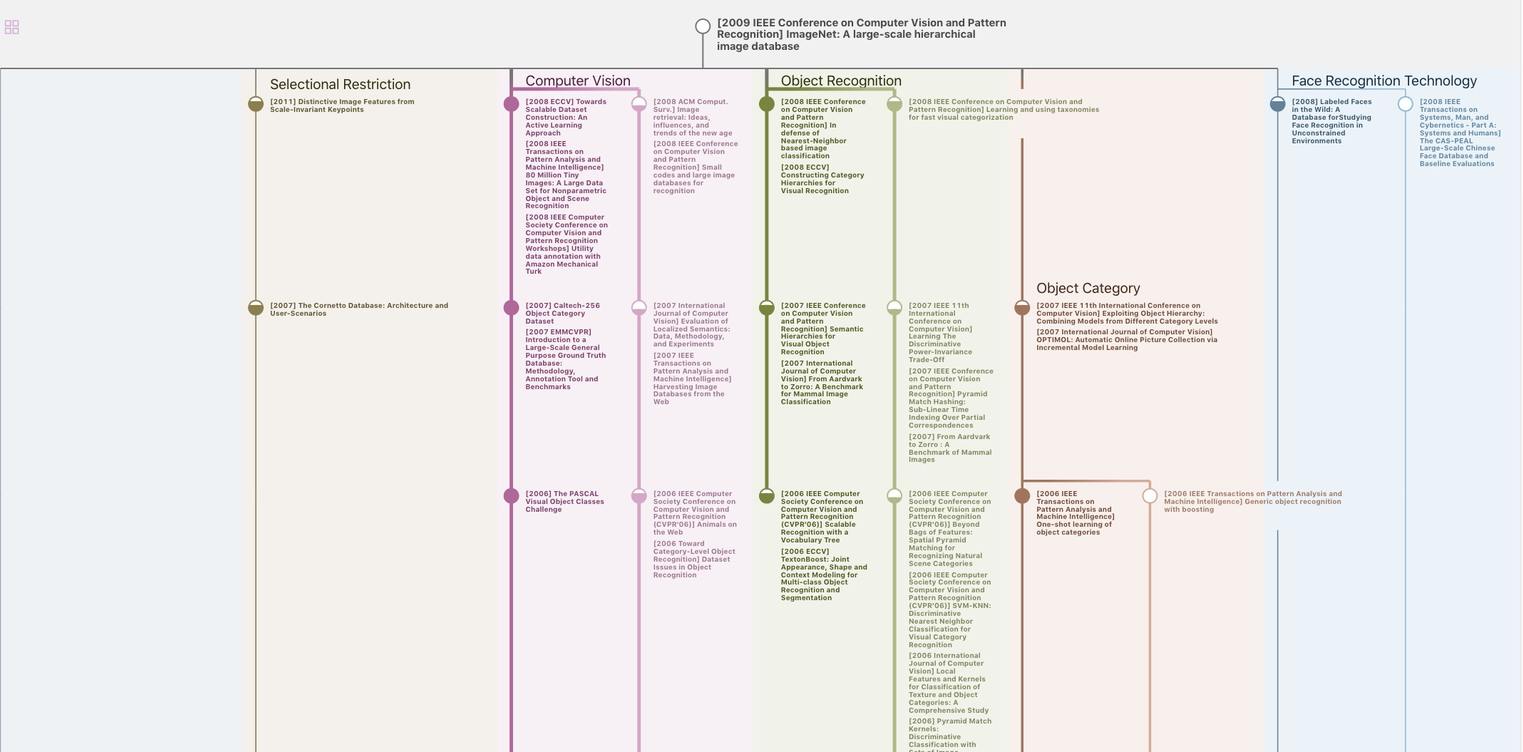
生成溯源树,研究论文发展脉络
Chat Paper
正在生成论文摘要