Multi-sensor cross-domain fault diagnosis method for leakage of ship pipeline valves
Ocean Engineering(2024)
摘要
The operating environments of ship pipeline valves are complicated. A single sensor may struggle to comprehensively characterize the valve fault features. Pressure variations in the pipeline network may result in changes of the feature distribution of the valve leakage acoustic emission (AE) signals, which makes it challenging for traditional data-driven detection models to achieve satisfactory generalization performance. Unfortunately, there has been limited attention in the current literature on multi-sensor cross-domain fault diagnosis for the ship pipeline valves. To address this research gap, this study aims to develop a novel multi-sensor cross-domain fault diagnosis method. Firstly, the time-frequency-multisqueezing transform (TFMST) is employed for time-frequency analysis of the valve leakage AE signals. By accurately describing harmonic and pulse components in the AE signals, the time-frequency domain features of the valve leakage can be extracted. Subsequently, a multi-sensor deep transfer learning model based on multi-channel fusion convolutional neural network (MCFCNN) is proposed, which utilizes the domain adaptation strategy to minimize the maximum mean discrepancy between source domain data and target domain data, significantly improving the robustness of the fault detection to variations of operating environments. Experimental testing has been carried out to evaluate the performance of the proposed method, and the results indicate effective valve leakage detection.
更多查看译文
关键词
Valve leakage,Acoustic emission,Sensor fusion,Deep transfer learning
AI 理解论文
溯源树
样例
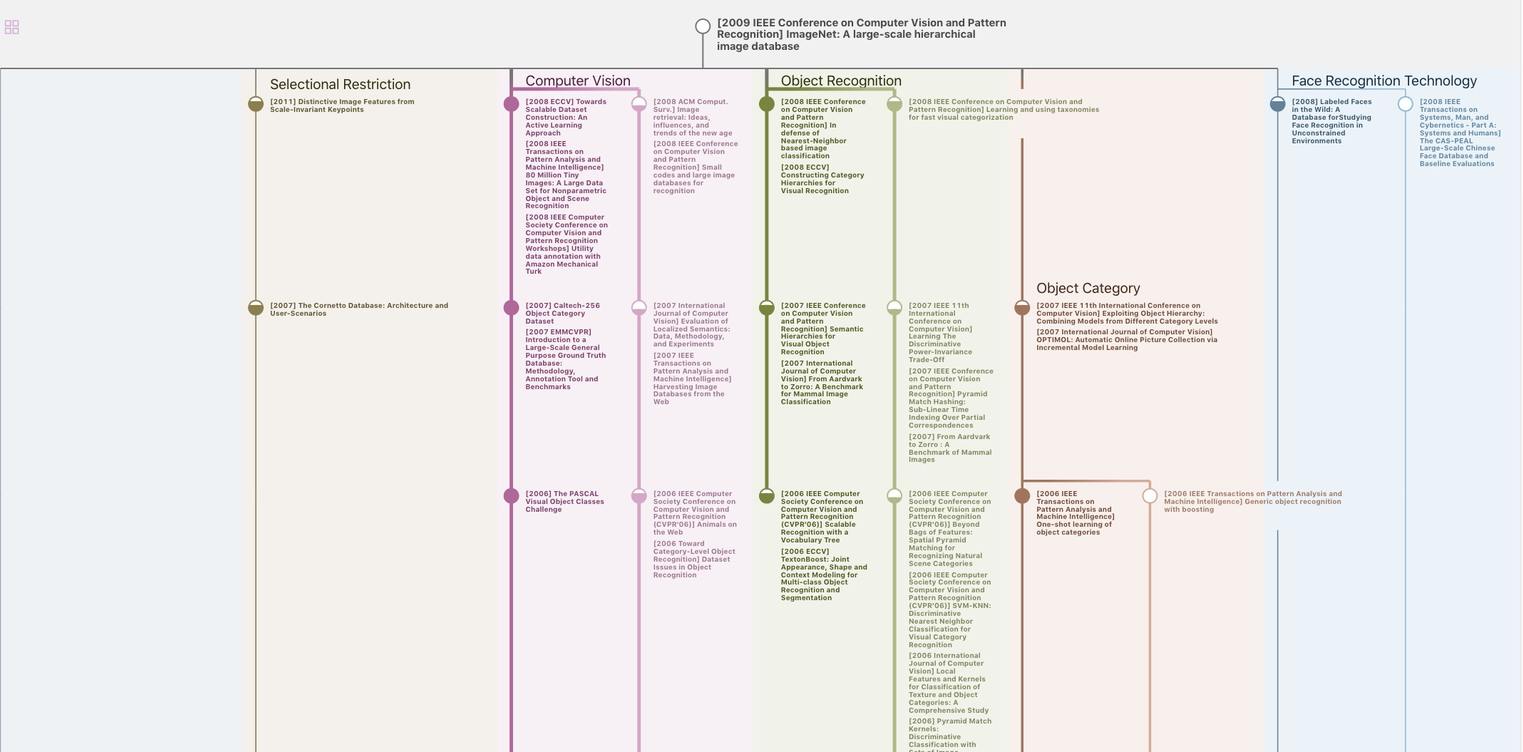
生成溯源树,研究论文发展脉络
Chat Paper
正在生成论文摘要