Physical model embedding-based generative adversarial networks for unsupervised fault detection of underwater thrusters
OCEAN ENGINEERING(2024)
摘要
This paper proposes a hybrid approach incorporating a physical model into generative adversarial networks for unsupervised fault detection of underwater thrusters. Specifically, a thruster physical model with unknown coefficients is constructed according to the thruster speed and the motion state of the vehicle, and this physical model is used to replace the neural network to build the generator. Interpretable features are then extracted from the thruster control signals by the constructed feature-extracted network, and these features are used as the input of the generator. Furthermore, two current values (the generated and the measured) are fed into the discriminator for adversarial learning. Finally, considering that the distribution of measured current values may deviate from the generated current values as the health of the thruster deteriorates, the output of the discriminator can be used to indicate the state of the thruster. The effectiveness of the proposed framework is demonstrated through two fault cases. Experimental results show that the proposed framework can accurately detect faults and describe fault development trends, outperforming the state -of -the -art methods.
更多查看译文
关键词
Underwater vehicle,Underwater thruster,Unsupervised fault diagnosis,Physical models,Generative adversarial network
AI 理解论文
溯源树
样例
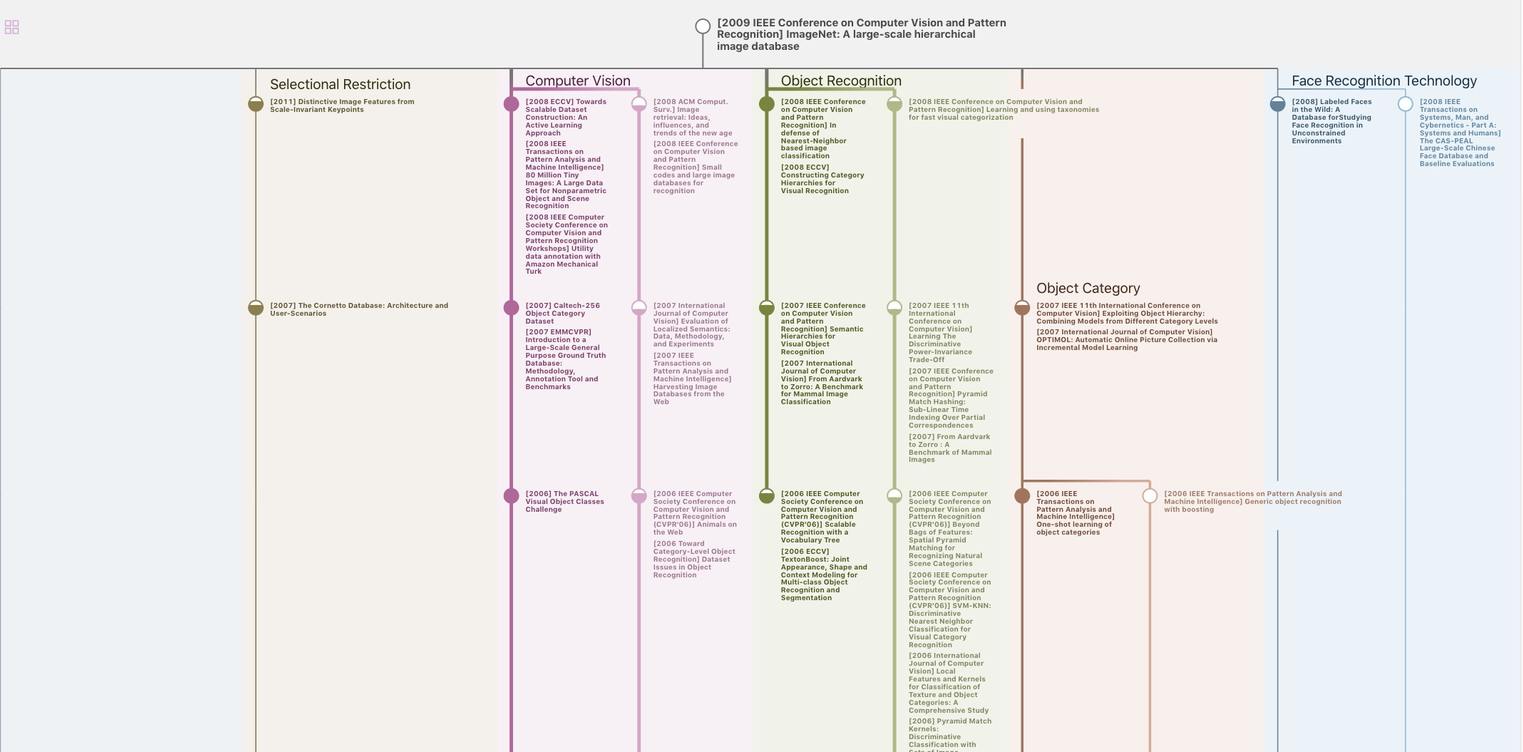
生成溯源树,研究论文发展脉络
Chat Paper
正在生成论文摘要