Tool condition monitoring in drilling processes using anomaly detection approaches based on control internal data
Procedia CIRP(2024)
摘要
The tool lifetime and the material removal rate significantly influence the production costs of a machined component. In order to maintain process reliability, tools are often changed too early in industrial practice, which is a waste of resources and results in increased tool costs and extended setup times. Tool condition monitoring systems based on machine learning have shown great potential to detect and predict tool wear, thereby reducing the risk of tool failure and optimising tool change intervals. Nevertheless, common supervised learning methods for tool wear detection require extensive data generation and high labeling effort. This paper presents two approaches with minimal to no labeling effort for predicting the end of the service life of drilling tools based on anomaly detection. Therefore, a classification approach to anomaly detection based on XGBoost and an unsupervised autoencoder architecture are evaluated on data acquired during machining experiments. In addition, the performance when applying the models of both approaches to other cutting parameters is investigated. Beyond a high classification performance on all datasets, the XGBoost approach can also be used as to derive an indicator for reaching the end of life, even when applied to other cutting parameters. For the autoencoder approach, it is shown that its reconstruction error correlates with the anomaly label and thus can also be used for anomaly detection.
更多查看译文
关键词
manufacturing,machining,process monitoring,tool condition monitoring,machine learning,anomaly detection,time series analysis,neural networks
AI 理解论文
溯源树
样例
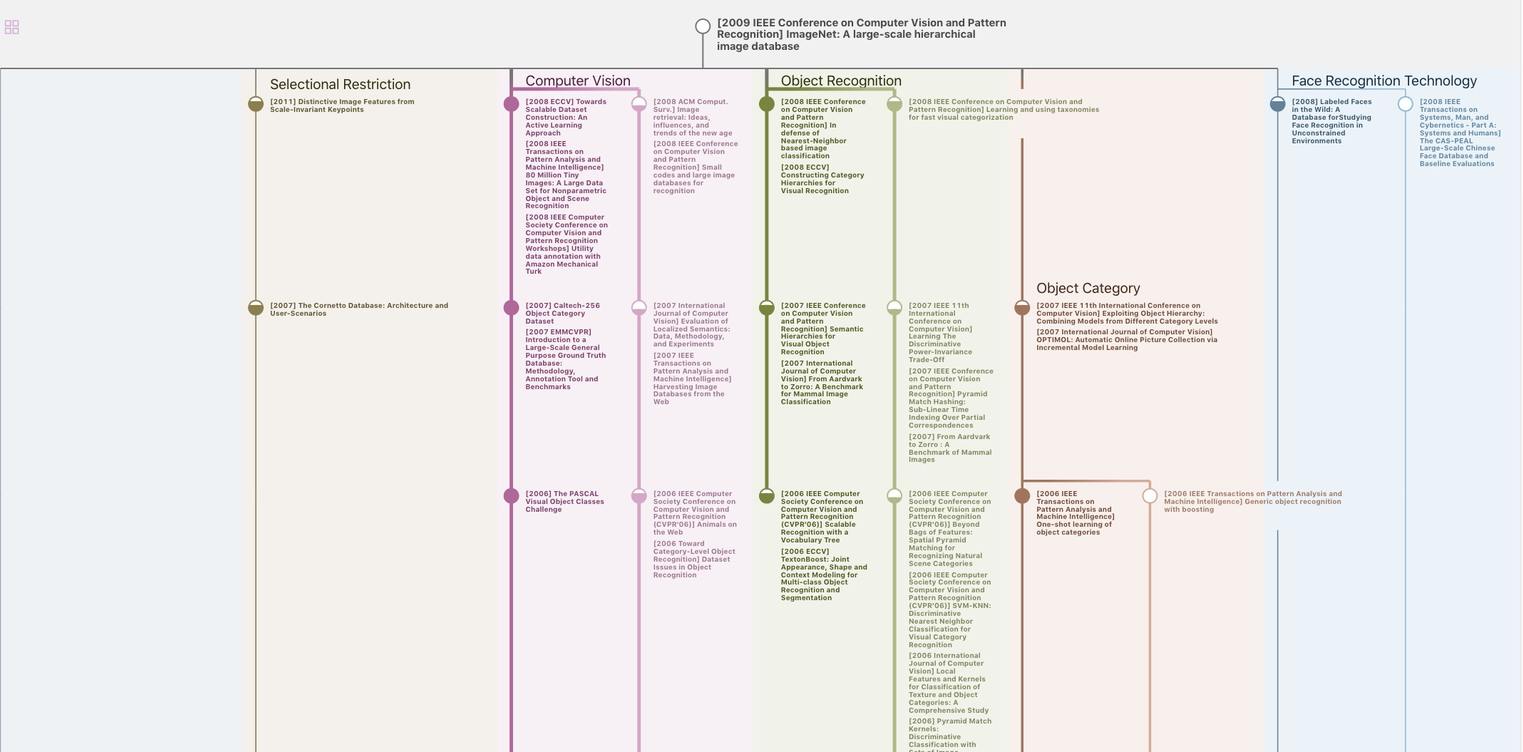
生成溯源树,研究论文发展脉络
Chat Paper
正在生成论文摘要