Multi-granularity Cross Transformer Network for person re-identification
PATTERN RECOGNITION(2024)
摘要
Person re -identification (Re -ID) aims to retrieve the same person in the gallery. Transformers have been introduced to the Re -ID task due to their excellent ability to model long-range dependency. However, due to the properties of the global attention mechanism, they are less effective in capturing the discriminative local semantics of pedestrians compared to convolutional operations. To address this issue, we present a Multi -granularity Cross Transformer Network (MCTN) that progressively learns salient features of different local structures in a global context. Specifically, we first utilize a Multi -granularity Convolutional Layer (MCL) to investigate salient pedestrian features at various granularities. On this basis, we propose a Pyramidal Cross Transformer learning layer (PCT), which contains a pyramidal division of pedestrian image feature maps, differentiated feature extraction of different parts of pedestrians, and cross attention to exploring the local- global relationship of the feature map. It allows effective mining of local information in the global structure from a coarse -to -fine perspective. Furthermore, to enhance the interaction between low-level detailed features and high-level semantic features, a Hierarchical Aggregation Strategy (HAS) is introduced to fuse features learned by cross attention learning at different stages. Pedestrian features learned in shallow layers will serve as global priors for semantics learning in deep layers. We evaluate our method on four large-scale Re -ID datasets, and the experimental results reveal that the proposed method outperforms the state-of-the-art methods.
更多查看译文
关键词
Person re-identification,Cross transformer,Multi-granularity
AI 理解论文
溯源树
样例
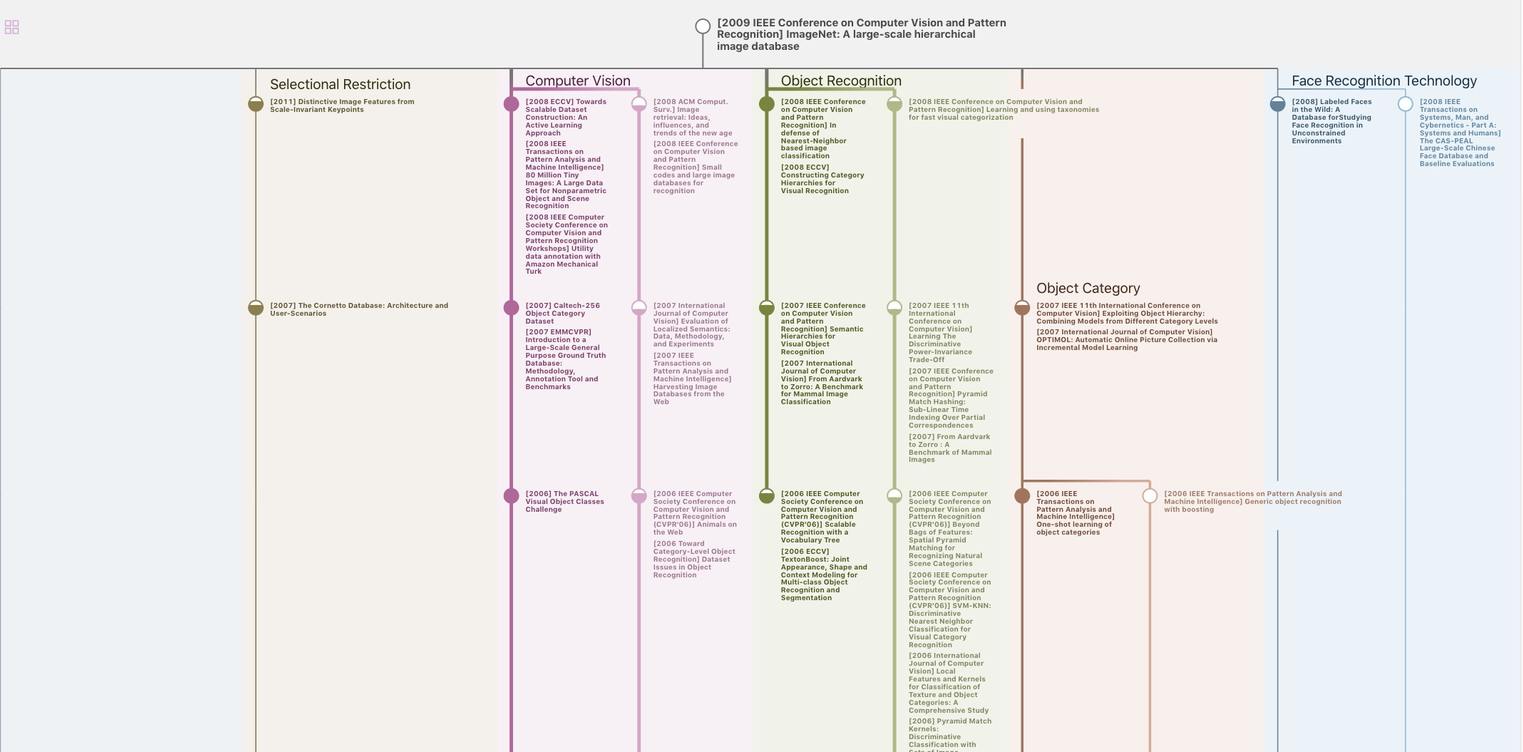
生成溯源树,研究论文发展脉络
Chat Paper
正在生成论文摘要