Sample diversity selection strategy based on label distribution morphology for active label distribution learning
PATTERN RECOGNITION(2024)
摘要
Labeling a sample in label distribution learning is highly expensive because it involves several labels at the same time and also requires assigning an exact value as the significance of each label. Therefore, active learning, which lowers the labeling cost by actively querying the labels of the most useful data, becomes especially critical for label distribution learning. Most of the known active query algorithms are for multilabel learning, and applying them directly to label distribution learning will lose some key supervisory information and thus fail to yield satisfactory experimental results. In this paper, we propose a sample diversity selection strategy based on the label distribution morphology, which can select diverse samples with different distribution morphologies from a large number of unlabeled samples for active querying. First, we use the feature space to construct a dissimilarity matrix that describes the pairwise dissimilarity among the unlabeled samples in order to pick a subset of samples that are representative of the unlabeled dataset. Second, using the information about the label distribution morphologies provided by the predicted labels of the unlabeled samples, we design a diversity loss score for each unlabeled sample. This score reflects the degree of difference between the sample and the labeled training sample. Finally, we use a convex optimization method to select valuable samples that are diverse from the labeled samples and represent the distribution of the unlabeled samples. The results of the comparison experiments demonstrate the effectiveness of our approach.
更多查看译文
关键词
Label distribution learning,Active learning,Representativeness,Diversity,Label distribution morphology
AI 理解论文
溯源树
样例
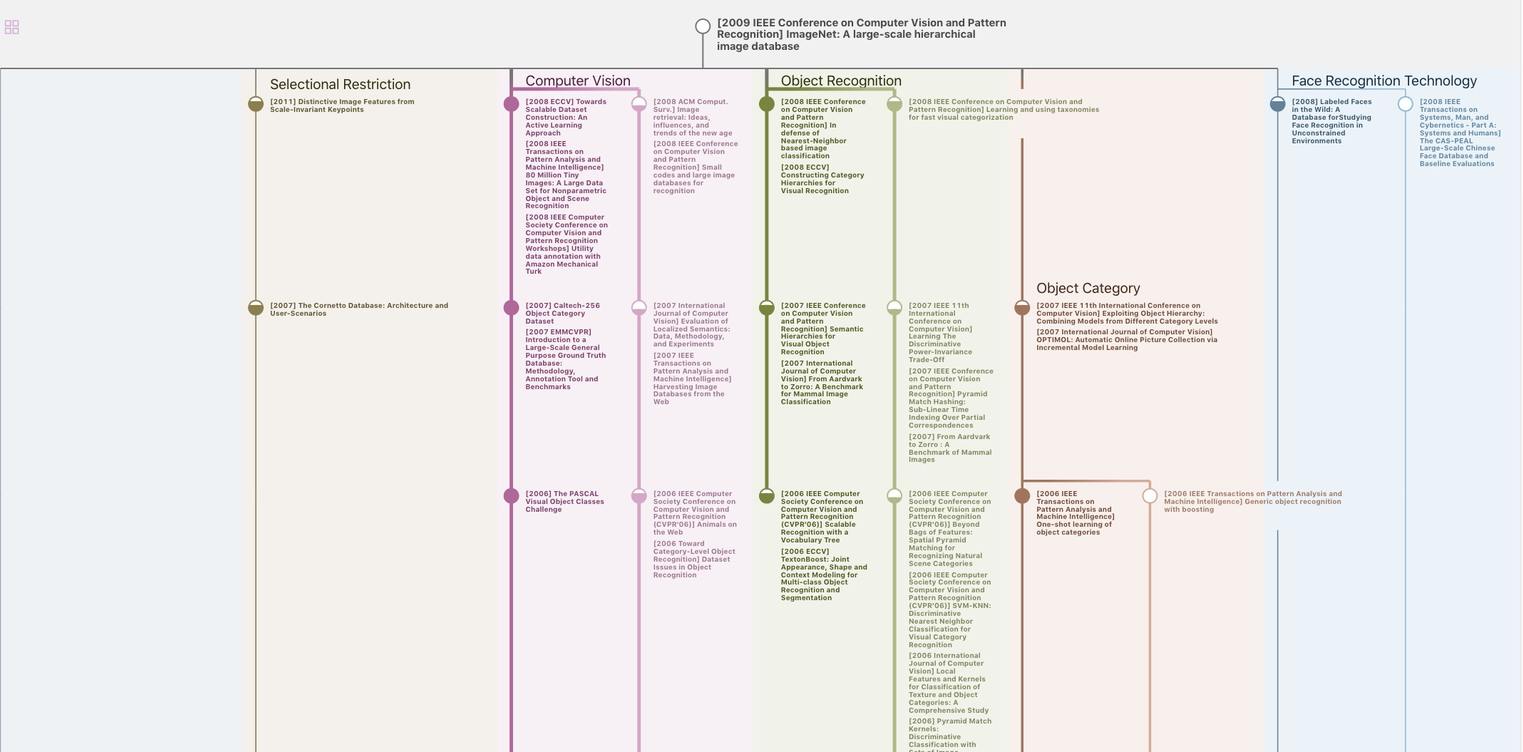
生成溯源树,研究论文发展脉络
Chat Paper
正在生成论文摘要