Predicting lodging severity in dry peas using UAS-mounted RGB, LIDAR, and multispectral sensors
Remote Sensing Applications: Society and Environment(2024)
摘要
This study investigated the feasibility of using data collected by sensors mounted onto unmanned aerial systems (UASs) and traditional classification methods (Maximum Likelihood, Mahalanobis Distance, Minimum Distance, and Adaptive Coherence Estimator) and four machine learning models (Random Forest [RF], Support Vector Machine [SVM], K-Nearest Neighbor [KNN], and Extreme Gradient Boosting [XGBOOST]) to predict the severity of dry pea lodging. Data were collected in two environments in North Dakota during the 2022 crop season, where lodging was visually estimated at the plot level using a lodging scale range of 0 to 9. Aerial images were collected using two UASs (DJI Matrice 200 and 300) during early, mid, and late stages of growth. Synthetic Minority Oversampling Technique combined with Edited Nearest Neighbors (SMOTE-ENN) was used to balance the imbalanced dataset. Different feature selection models (recursive feature elimination, lasso regression, principal component analysis, and correlation-based methods) were used and compared to identify the most important features in the dataset. Our study found that similar channels can be used interchangeably for analyzing lodging without compromising accuracy, simplifying data acquisition, and saving time and resources. The maximum plant height values obtained from LiDAR and RGB sensors showed a strong correlation with actual values. Recursive feature elimination was the most effective feature selection method for handling high-dimensional datasets. The XGBOOST model outperformed all traditional and machine learning classification models, with the lowest variation in precision values. RF showed the most pronounced overfitting among the machine learning models, while Mahalanobis distance showed the least overfitting among all traditional classification models. Overall, the study demonstrated the potential of UAS and machine learning models for predicting lodging severity in dry peas, with XGBOOST being the best-performing model.
更多查看译文
关键词
Lodging,Dry pea,Multidimensional dataset,Machine learning,UAS,High-throughput plant phenotyping,LIDAR
AI 理解论文
溯源树
样例
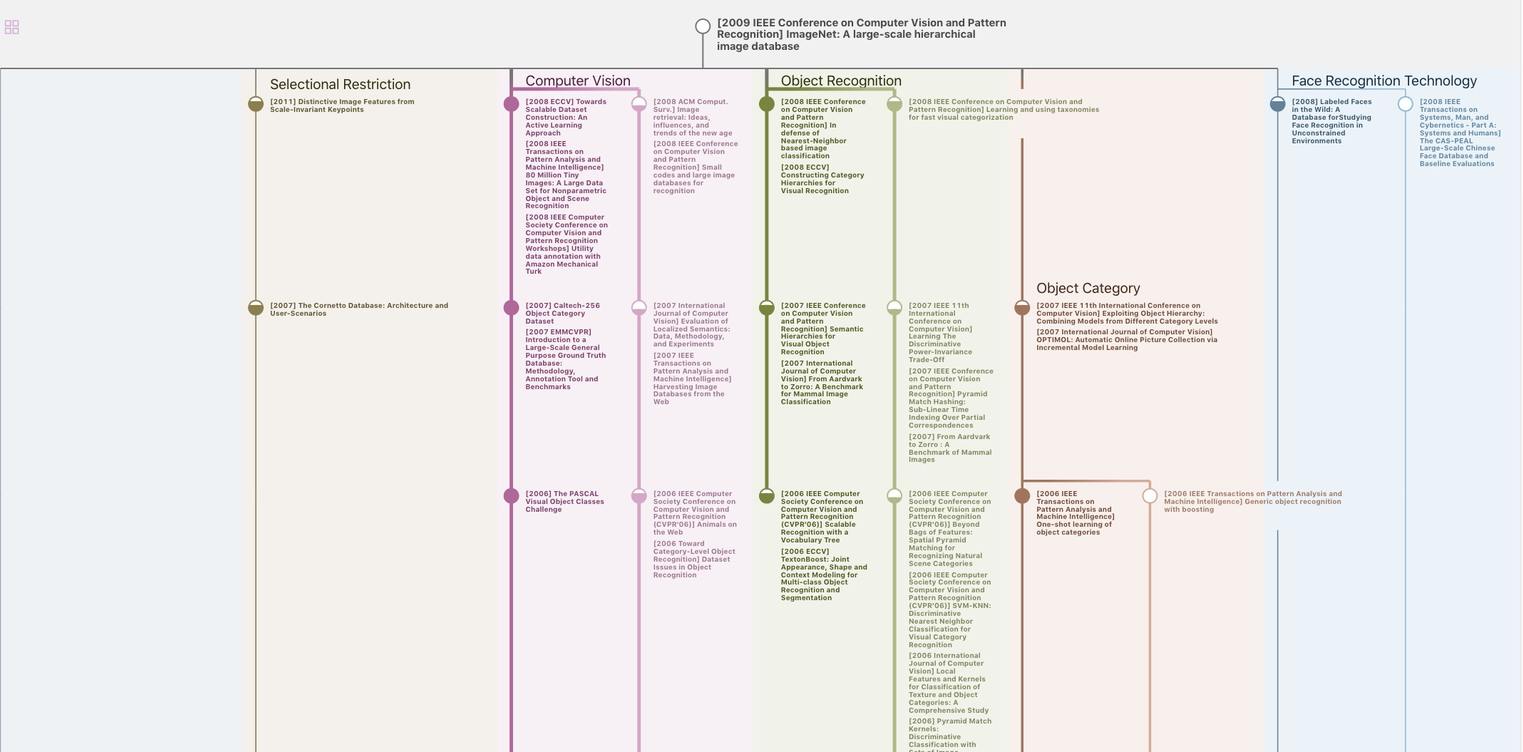
生成溯源树,研究论文发展脉络
Chat Paper
正在生成论文摘要