Machine learning techniques to predict atmospheric black carbon in a tropical coastal environment
REMOTE SENSING APPLICATIONS-SOCIETY AND ENVIRONMENT(2024)
摘要
Black Carbon (BC) is a crucial atmospheric pollutant with significant impacts on both human health and climate change. Therefore, continuous measurement of this pollutant is vital to comprehend its complexities and variations in the future. However, acquiring BC data is challenging due to the limited availability of ground-based measurements. In this study, machine-learningbased models are used to overcome such challenges and predict their variability. The study has three major objectives such as; i) emphasis on long-term monitoring of BC mass concentration using ground-based measurements for determining the trend, ii) developing a machine learning model to estimate and predict BC concentration, and iii) substituting observational data with reanalysis data (MERRA2) in the same model to address the concerns faced by sparse ground-based observations. Consequently, the performance of three machine learning (ML) methods: ARIMA, Random Forest, and XGBoost were assessed. XGBoost surpassed the other two ML algorithms, delivering the best R2 value of 0.763 (R = 0.862) with RMSE = 0.941 mu gm-3, MAE = 0.052 mu gm-3, and MAPE = 4.3% in the shortest amount of time. Later, the XGBoost model was fitted with several meteorological parameters, where temperature and wind speed were identified as key factors affecting BC concentration. The modified model was able to capture nearly 79% of observations with reduced error values. This demonstrated that taking meteorological conditions into account helped enhance BC estimation. When applied to MERRA2 data, the XGBoost model performed exceptionally well, with R2 = 0.879 (R = 0.937), RMSE = 0.455 mu gm-3, MAE = 0.109 mu gm-3, and MAPE = 5%. The analysis revealed a reasonable degree of agreement between observed and anticipated values highlighting the potential of ML modeling for BC variability simulations.
更多查看译文
关键词
Machine learning (ML),Black carbon,MERRA2,Meteorological parameters
AI 理解论文
溯源树
样例
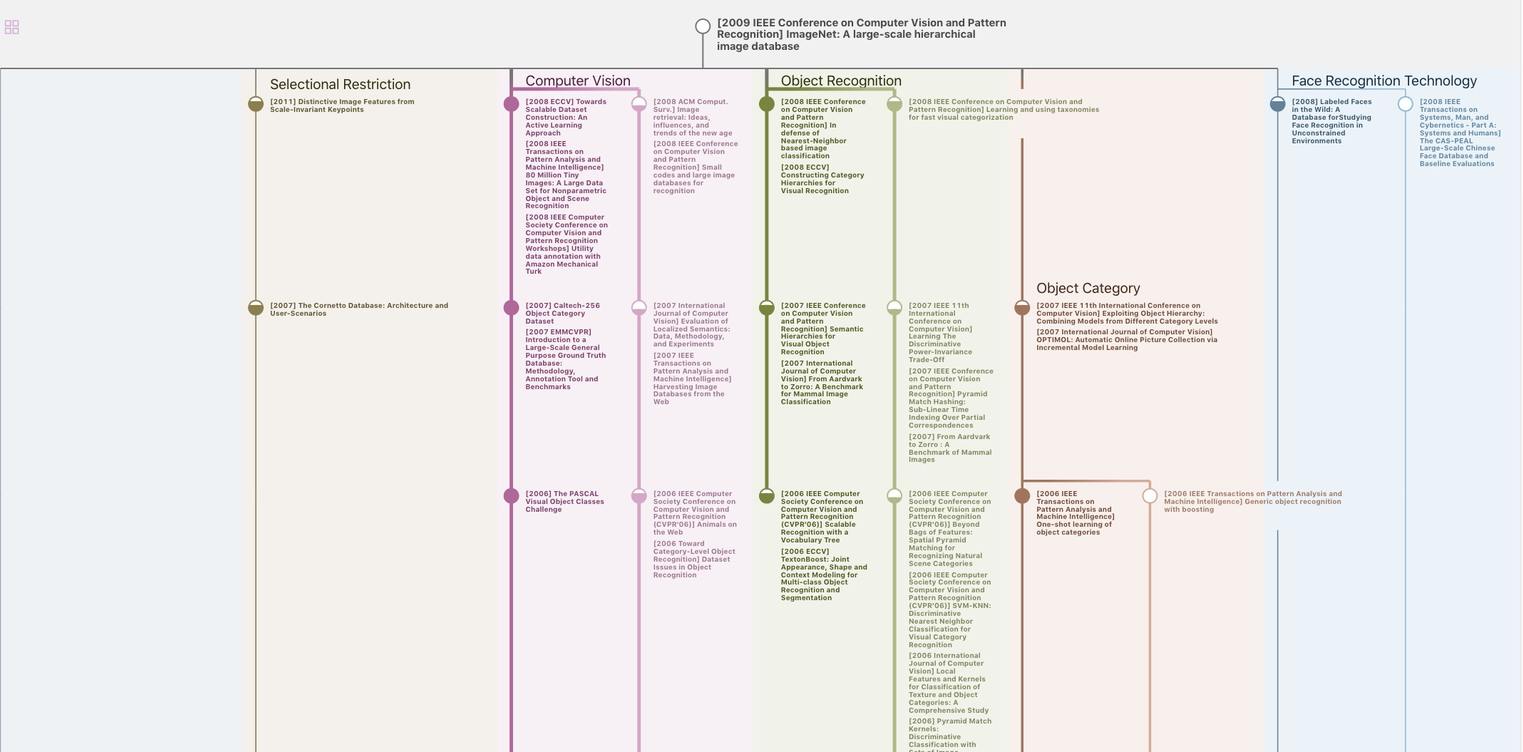
生成溯源树,研究论文发展脉络
Chat Paper
正在生成论文摘要