Exploring sludge yield patterns through interpretable machine learning models in China's municipal wastewater treatment plants
Resources, Conservation and Recycling(2024)
摘要
Sludge management remains a challenge for municipal wastewater treatment plants (MWWTPs). In this study, we use machine learning models to predict sludge yield and employ interpretable methods to highlight the driving factors. We analyze over 27,000 data entries of monthly plant-level operational details to predict the sludge yield for 177 MWWTPs in 11 cities throughout China. Evaluated by multiple statistical indicators including Coefficient of Determination (R2), Mean Absolute Error (MAE), Normalized Mean Absolute Error (NMAE), Mean Square Error (MSE) and Root Mean Square Error (RMSE), the machine learning model's performance proves superior to empirical estimation. Interpretative analysis reveals that pollutant removal quantities exert a more substantial influence on sludge yield than influent pollutant concentrations. The sludge yield becomes increasingly sensitive to wastewater quality when effluent discharge standards rise. The integration of interpretable machine learning models expands the research scope to a more holistic perspective, catalyzing interdisciplinary collaboration and novel insights.
更多查看译文
关键词
Municipal wastewater treatment plants (MWWTPs),Sludge yield,Machine learning model,Interpretative analysis
AI 理解论文
溯源树
样例
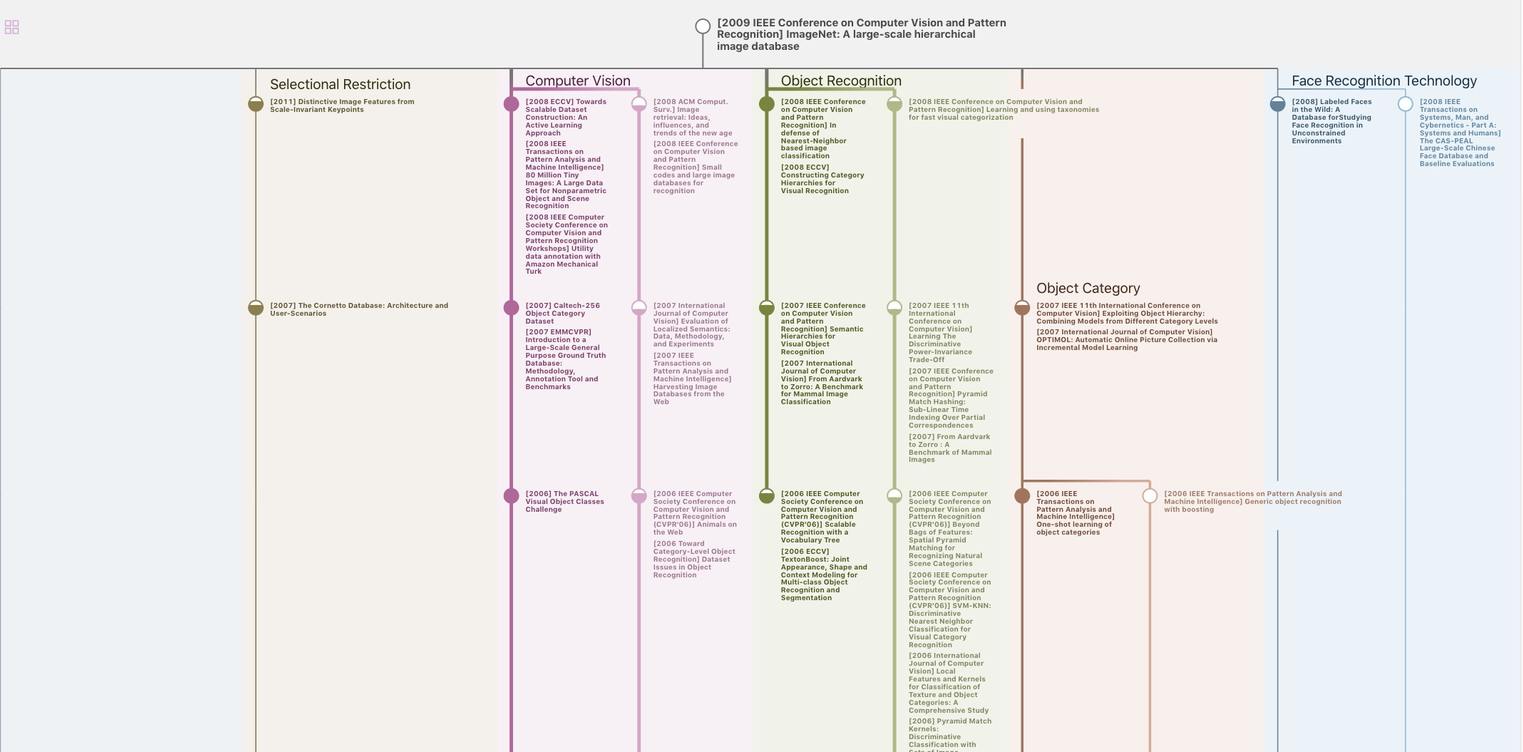
生成溯源树,研究论文发展脉络
Chat Paper
正在生成论文摘要