Optimizing CO2 capture in pressure swing adsorption units: A deep neural network approach with optimality evaluation and operating maps for decision-making
SEPARATION AND PURIFICATION TECHNOLOGY(2024)
摘要
This study presents a methodology for surrogate optimization of cyclic adsorption processes, focusing on enhancing pressure swing adsorption units for carbon dioxide (CO2) capture. We developed and implemented a multiple -input, single -output (MISO) framework comprising two deep neural network (DNN) models, predicting key process performance indicators. These models were then integrated into an optimization framework, leveraging particle swarm optimization (PSO) and statistical analysis to generate a comprehensive Pareto front representation. This approach delineated feasible operational regions (FORs) and highlighted the spectrum of optimal decision -making scenarios. A key aspect of our methodology was the evaluation of optimization effectiveness. This was accomplished by testing decision variables derived from the Pareto front against a phenomenological model, affirming the surrogate model's reliability. Subsequently, the study delved into analyzing the feasible operational domains of these decision variables. A detailed correlation map was constructed to elucidate the interplay between these variables, thereby uncovering the most impactful factors influencing process behavior. The study offers a practical, insightful operational map that aids operators in pinpointing the optimal process location and prioritizing specific operational goals.
更多查看译文
关键词
Pressure swing adsorption,Multiobjective optimization,Surrogate model,Feasible operation region,Optimally
AI 理解论文
溯源树
样例
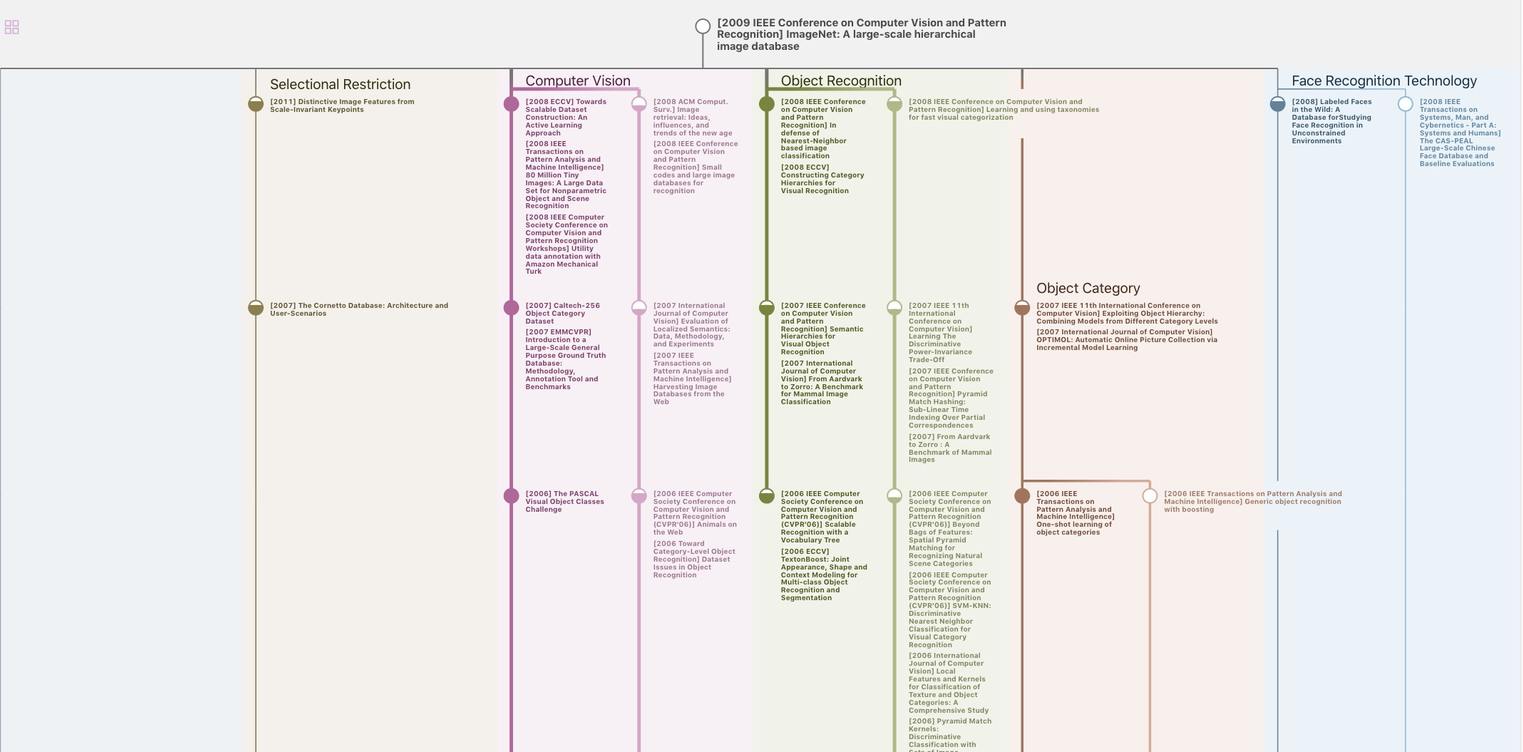
生成溯源树,研究论文发展脉络
Chat Paper
正在生成论文摘要