Precise chlorine determination in geological materials using LIBS coupled with stable learning for Mars explorations
SPECTROCHIMICA ACTA PART B-ATOMIC SPECTROSCOPY(2024)
摘要
Chlorine plays an important role in Mars explorations by offering insights into the planet's ancient geological processes, such as hydrothermal and volcanic activities. In-situ detections of chlorine on Mars have been reported by using various instruments onboard landers or rovers, and in particular laser-induced breakdown spectroscopy (LIBS). The detection with LIBS is limited by the faintness of the chlorine lines, and affected by matrix effect as well as various chemical speciation of chlorine in a geological sample. Alternatively, emissions from CaCl molecules enable a more sensitive detection of chlorine, but precise quantification is still elusive due to the dependence on calcium as cation in the molecule formation. Beyond the univariate approach using atomic or molecular emissions, multivariate regressions based on machine learning have been proven able to offer an effective correction of matrix effect. A preliminary attempt highlighted the challenge of applying this method to chlorine detection, stemming from the prevalence in a LIBS spectrum of the lines emitted by the cations detached from chlorides, which are alkali or alkaline earth metals with emission lines much more intense than those of chlorine. These intense lines introduce confounding variables in the model training, which compromises the performance of the trained model, and especially, leads to unstable predictive accuracies across different types of samples. In this work with an experiment in a laboratory-simulated Mars environment, we developed a method based on stable learning to improve the robustness and stability of a machine learning-based multivariate model. This was achieved by mitigating the impact of confounding variables via an elaborated feature selection guided by prior physical knowledge, and implementing a sample weighting procedure to decorrelate the features in the model training phase. Alongside a training sample set comprising various matrices and distinct chemical forms of chlorine-bearing compounds, two independent sample sets were designed to test the trained models. The first test set comprises samples that are similar to the training ones in terms of both matrix and chlorine-bearing compounds. Conversely, the second test set contains samples that differ from the training ones in both the matrix and the chemical speciation of chlorine. The optimized stable learning regression model exhibits a limit of detection (LOD) of 0.16 wt% and achieves root mean square errors of prediction (RMSEP) of 0.57 wt% and 0.59 wt% for the two test sets, respectively.
更多查看译文
关键词
LIBS,Chlorine quantification,Simulated martian environment,Feature engineering,Prior physical knowledge,Confounding variables,Stable learning
AI 理解论文
溯源树
样例
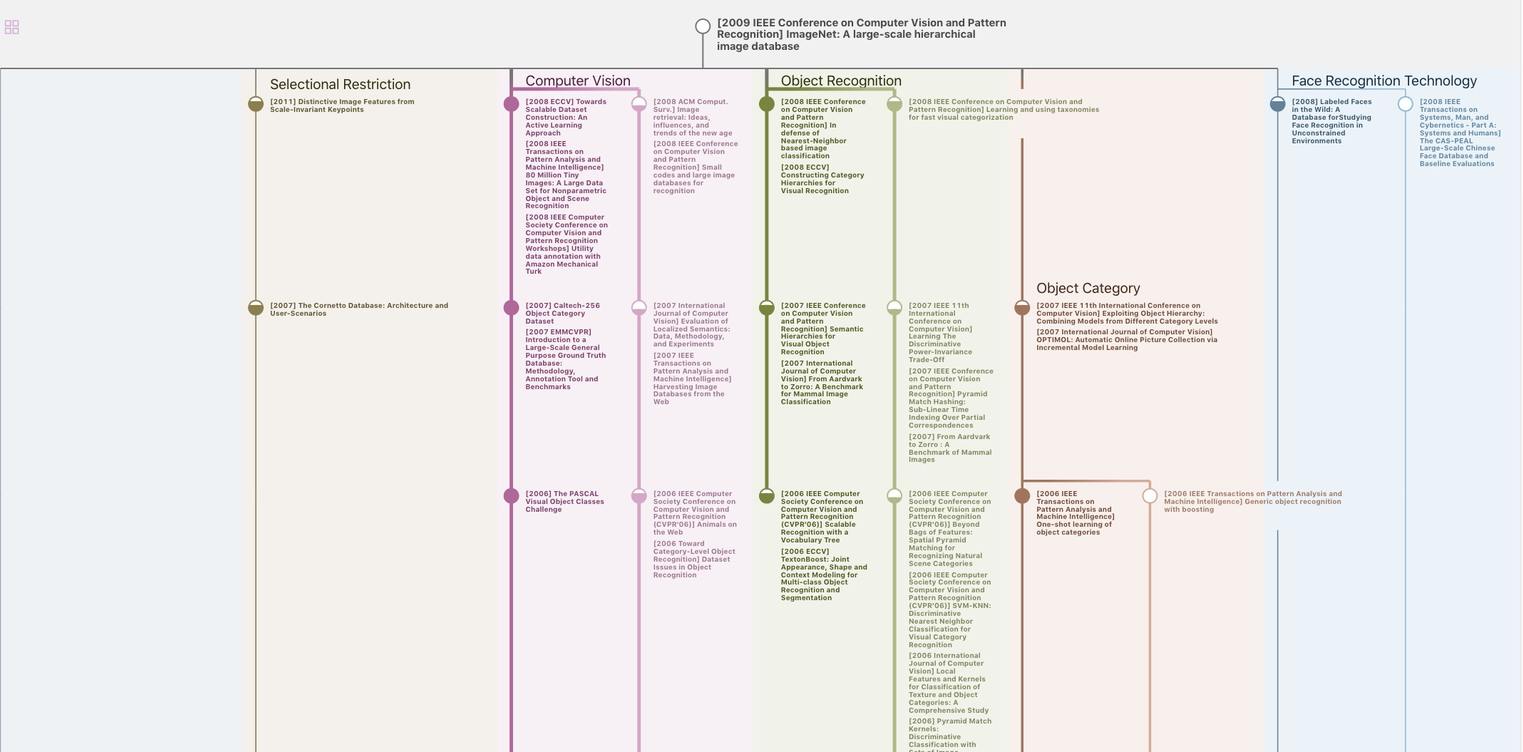
生成溯源树,研究论文发展脉络
Chat Paper
正在生成论文摘要