Deep learning in spectral analysis: Modeling and imaging
TRAC-TRENDS IN ANALYTICAL CHEMISTRY(2024)
摘要
Deep learning (DL) is powerful to find patterns or hidden information from data using neural networks. With the growth of data and computing capabilities, DL has rapidly advanced and shown great power in scientific research. In recent years, DL methods have been widely explored in spectral analysis. This review provides an overview of the advancements in DL techniques and highlights their recent applications in spectral analysis. Specifically, efforts in addressing the challenge of insufficient sample in DL methods, including transfer learning, data augmentation, and adversarial networks, are introduced. Furthermore, methods for improving model interpretability and data understanding are summarized. The review also covers efforts to adapt DL approaches for spectral analysis. In future studies, besides exploring more DL methods, it is more important to improve DL methods by integrating spectral expertise. In addition, the combination of representation learning and interpretability methods may constitute a promising direction.
更多查看译文
关键词
Deep learning,Neural network,Spectral modeling,Spectral imaging analysis
AI 理解论文
溯源树
样例
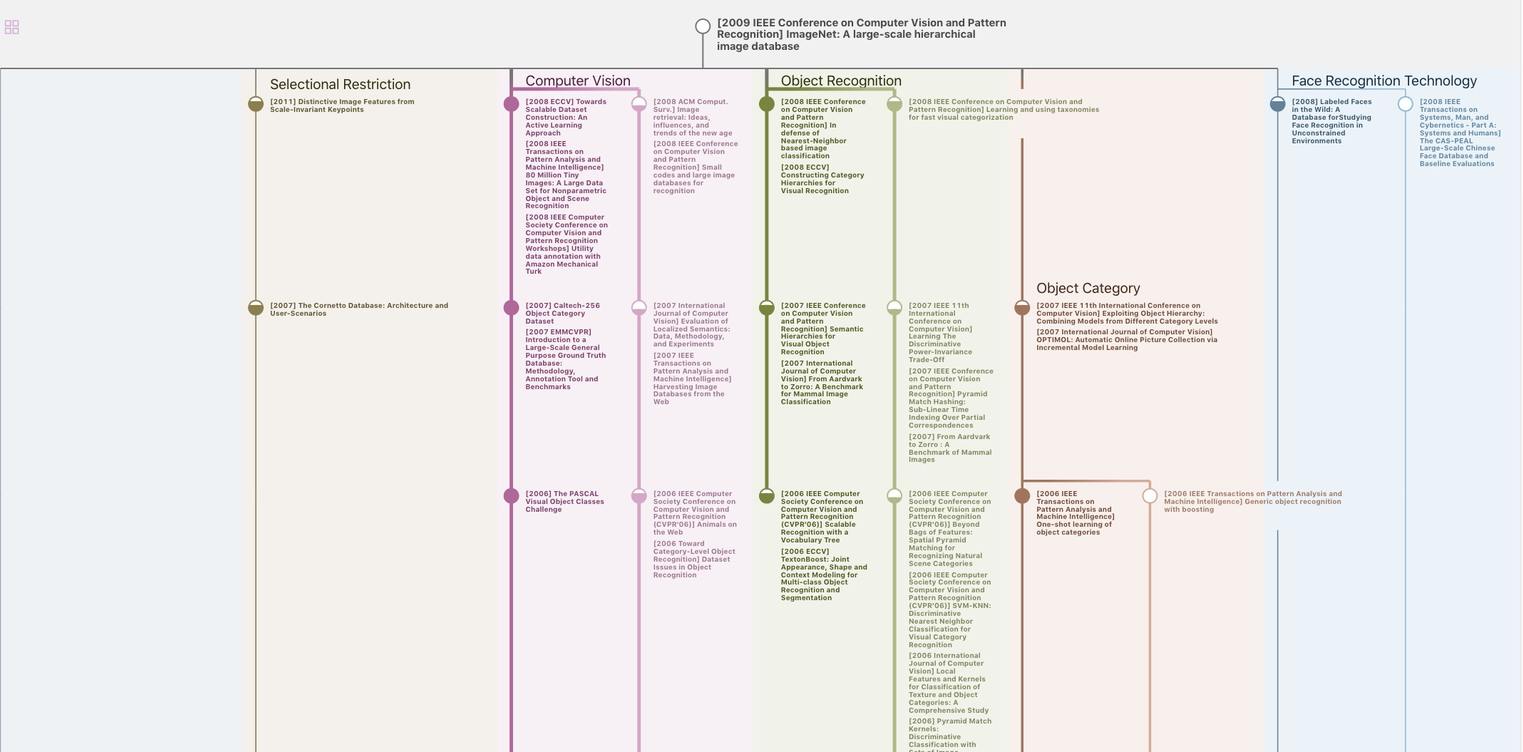
生成溯源树,研究论文发展脉络
Chat Paper
正在生成论文摘要