End-edge-cloud collaborative learning-aided prediction for high-speed train operation using LSTM
TRANSPORTATION RESEARCH PART C-EMERGING TECHNOLOGIES(2024)
摘要
This paper aims to incorporate the throttle handle level prediction in high speed train(HST) operation prediction problem to enable the prediction of HST drivers' activities, in which the key instructions available to HST driver are difficult to determine. Specifically, we consider an end -edge -cloud orchestration system to capture the real-time responses for driver state changes. By adding edge computing nodes, the real-time performance of data collection, transmission, and processing is improved. Our ultimate goal is to guide and regulate train drivers' activities in the same way, regardless of uncertain factors affecting HST dynamic or kinematic performance. We formulate the problem as a physical -based and data -driven deep learning -aided prediction model and solve it using a novel long short-term memory (LSTM) deep neural network which combines: (i) an off-line approximate training model to learn the time series data in the cloud layer, and (ii) an online prediction process to determine driving strategies in the realtime windows, more in general expressed as driving skill level constraints. To evaluate the performance of our approach, some case studies using the real -world railway infrastructure and HST data have been conducted. The results show that the proposed models produce higher prediction accuracy for both speed and throttle handle level prediction tasks. Compared to the conventional HST operation prediction problem, which considers speed sequences only without throttle handle level consideration, this study finds that jointly modeling speed and throttle handle level actually improves the next operation prediction performance itself, potentially because throttle handle level observations capture the information on HST control dynamics, which may affect operators' driving choices.
更多查看译文
关键词
High speed train operation,Speed prediction,Throttle handle level prediction,End-edge-cloud collaborative learning,LSTM
AI 理解论文
溯源树
样例
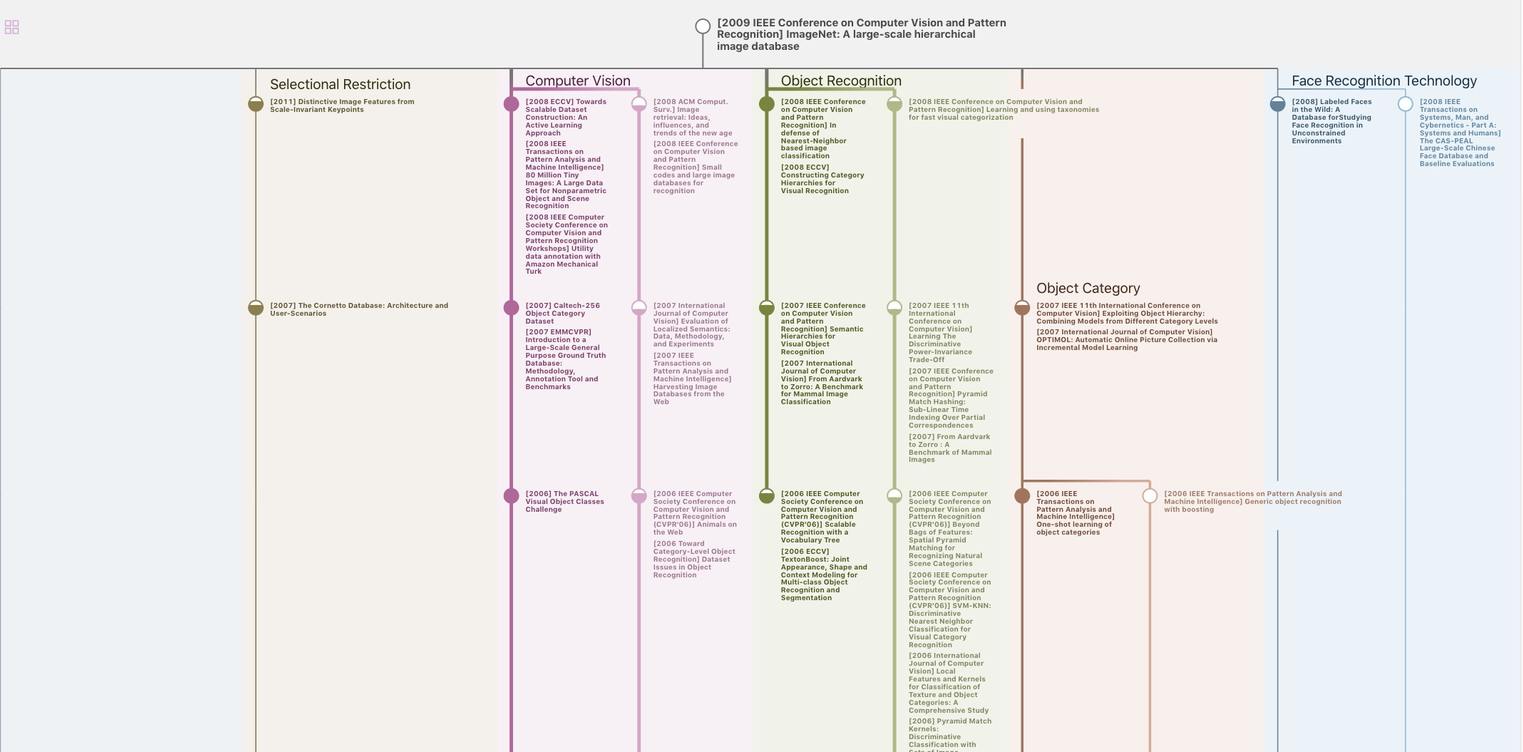
生成溯源树,研究论文发展脉络
Chat Paper
正在生成论文摘要