Enhancing photovoltaic module fault diagnosis: Leveraging unmanned aerial vehicles and autoencoders in machine learning
SUSTAINABLE ENERGY TECHNOLOGIES AND ASSESSMENTS(2024)
摘要
Photovoltaic (PV) modules play a pivotal role in renewable energy systems, underscoring the critical need for their fault diagnosis to ensure sustained energy production. This study introduces a novel approach that combines the power of deep neural networks and machine learning for comprehensive PV module fault diagnosis. Specifically, a fusion methodology that incorporates autoencoders (a deep neural network architecture) and support vector machines (SVM) (a machine learning algorithm) is proposed in the present study. To generate high-quality image datasets for training, unmanned aerial vehicles (UAVs) equipped with RGB cameras were employed to capture detailed images of PV modules. Burn marks, snail trails, discoloration, delamination, glass breakage and good panel were the conditions considered in the study. The experimental results demonstrate remarkable accuracy of 98.57% in diagnosing faults, marking a significant advancement in enhancing the reliability and performance of PV modules. This research contributes to the sustainability and efficiency of renewable energy systems, underlining its importance in the quest for a cleaner, greener future.
更多查看译文
关键词
Photovoltaic modules,Support vector machines,Autoencoders,Unmanned aerial vehicles
AI 理解论文
溯源树
样例
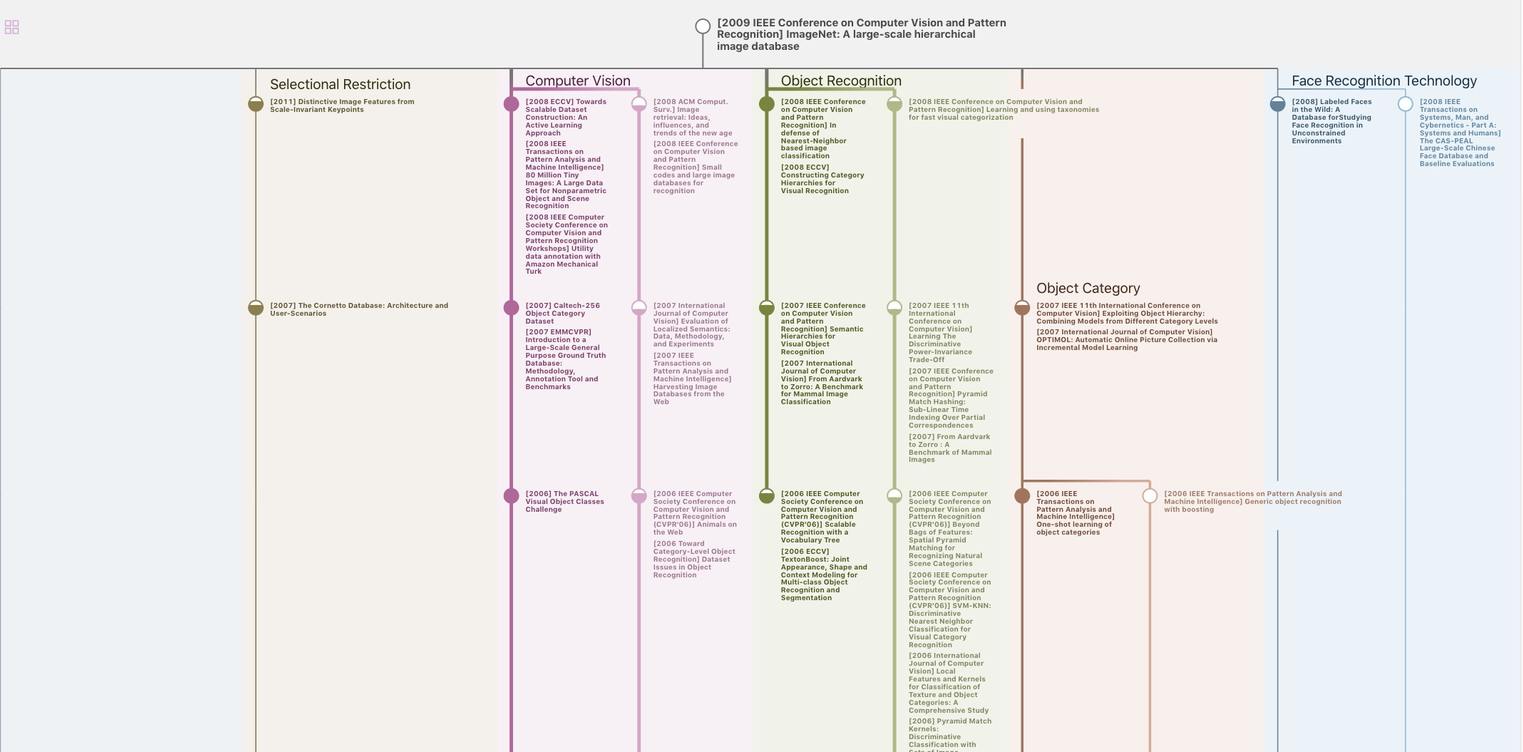
生成溯源树,研究论文发展脉络
Chat Paper
正在生成论文摘要