Hypergraph-based Truth Discovery for Sparse Data in Mobile Crowdsensing
ACM Transactions on Sensor Networks(2023)
摘要
Mobile crowdsensing leverages the power of a vast group of participants to collect sensory data, thus presenting an economical solution for data collection. However, due to the variability among participants, the quality of sensory data varies significantly, making it crucial to extract truthful information from sensory data of differing quality. Additionally, given the fixed time and monetary costs for the participants, they typically only perform a subset of tasks. As a result, the datasets collected in real-world scenarios are usually sparse. Current truth discovery methods struggle to adapt to datasets with varying sparsity, especially when dealing with sparse datasets. In this paper, we propose an adaptive Hypergraph-based EM truth discovery method, HGEM. The HGEM algorithm leverages the topological characteristics of hypergraphs to model sparse datasets, thereby improving its performance in evaluating the reliability of participants and the true value of the event to be observed. Experiments based on simulated and real-world scenarios demonstrate that HGEM consistently achieves higher predictive accuracy.
更多查看译文
关键词
mobile crowdsensing,truth discovery,hypergraph,sparse data
AI 理解论文
溯源树
样例
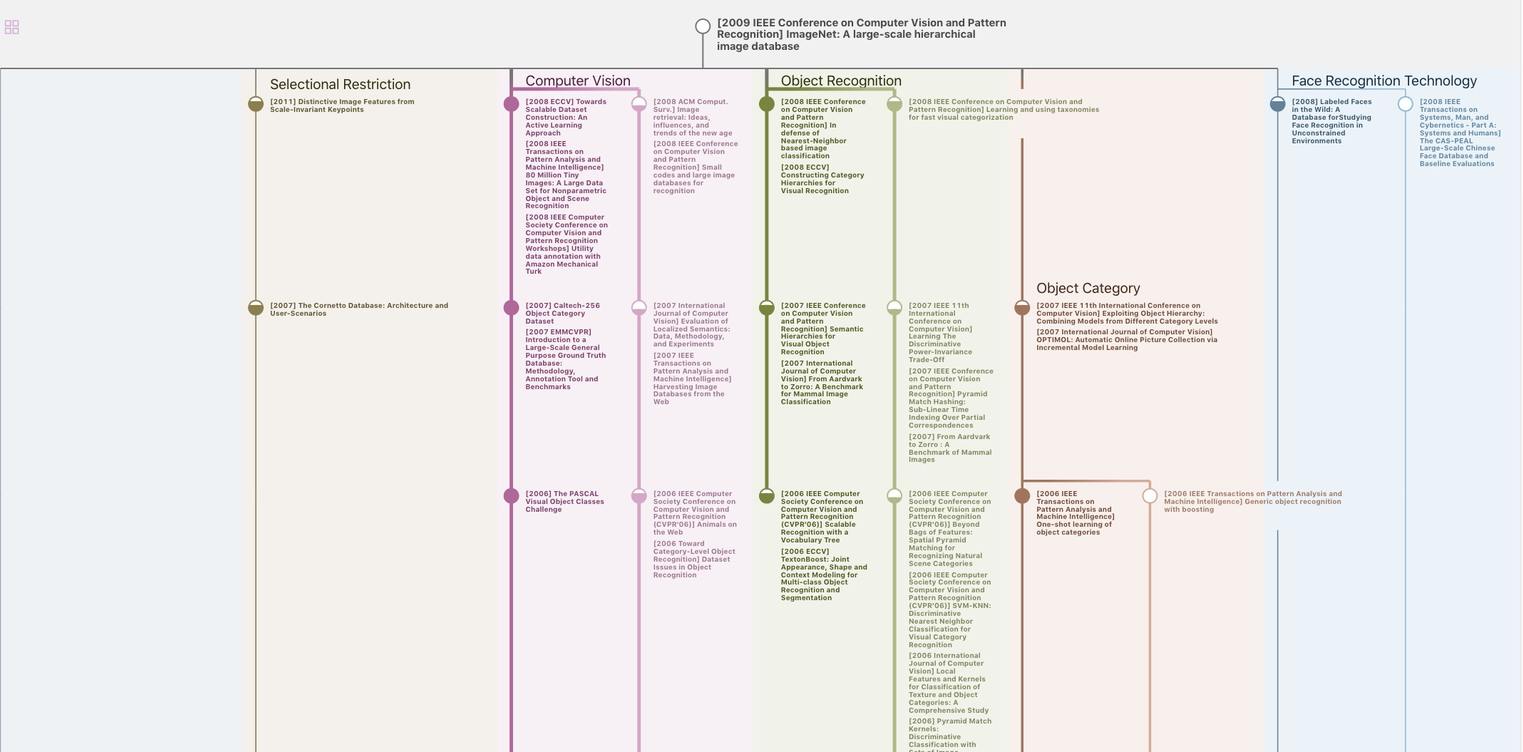
生成溯源树,研究论文发展脉络
Chat Paper
正在生成论文摘要