Few-shot Learning for Heterogeneous Information Networks
ACM Transactions on Information Systems(2022)
摘要
Heterogeneous information networks (HINs) are a key resource in many domain-specific retrieval and recommendation scenarios, and in conversational environments. Current approaches to mining graph data often rely on abundant supervised information. However, supervised signals for graph learning tend to be scarce for a new task and only a handful of labeled nodes may be available. Meta-learning mechanisms are able to harness prior knowledge that can be adapted to new tasks. In this paper, we design a meta-learning framework, called META-HIN , for few-shot learning problems on HINs. To the best of our knowledge, we are among the first to design a unified framework to realize the few-shot learning of HINs and facilitate different downstream tasks across different domains of graphs. Unlike most previous models, which focus on a single task on a single graph, META-HIN is able to deal with different tasks (node classification, link prediction, and anomaly detection are used as examples) across multiple graphs. Subgraphs are sampled to build the support and query set. Before being processed by the meta-learning module, subgraphs are modeled via a structure module to capture structural features. Then, a heterogeneous GNN module is used as the base model to express the features of subgraphs. We also design a GAN-based contrastive learning module that is able to exploit unsupervised information of the subgraphs. In our experiments, we fuse several datasets from multiple domains to verify META-HIN ’s broad applicability in a multiple-graph scenario. META-HIN consistently and significantly outperforms state-of-the-art alternatives on every task and across all datasets that we consider.
更多查看译文
关键词
Heterogeneous information network,Few-shot learning,Meta-learning,Graph mining
AI 理解论文
溯源树
样例
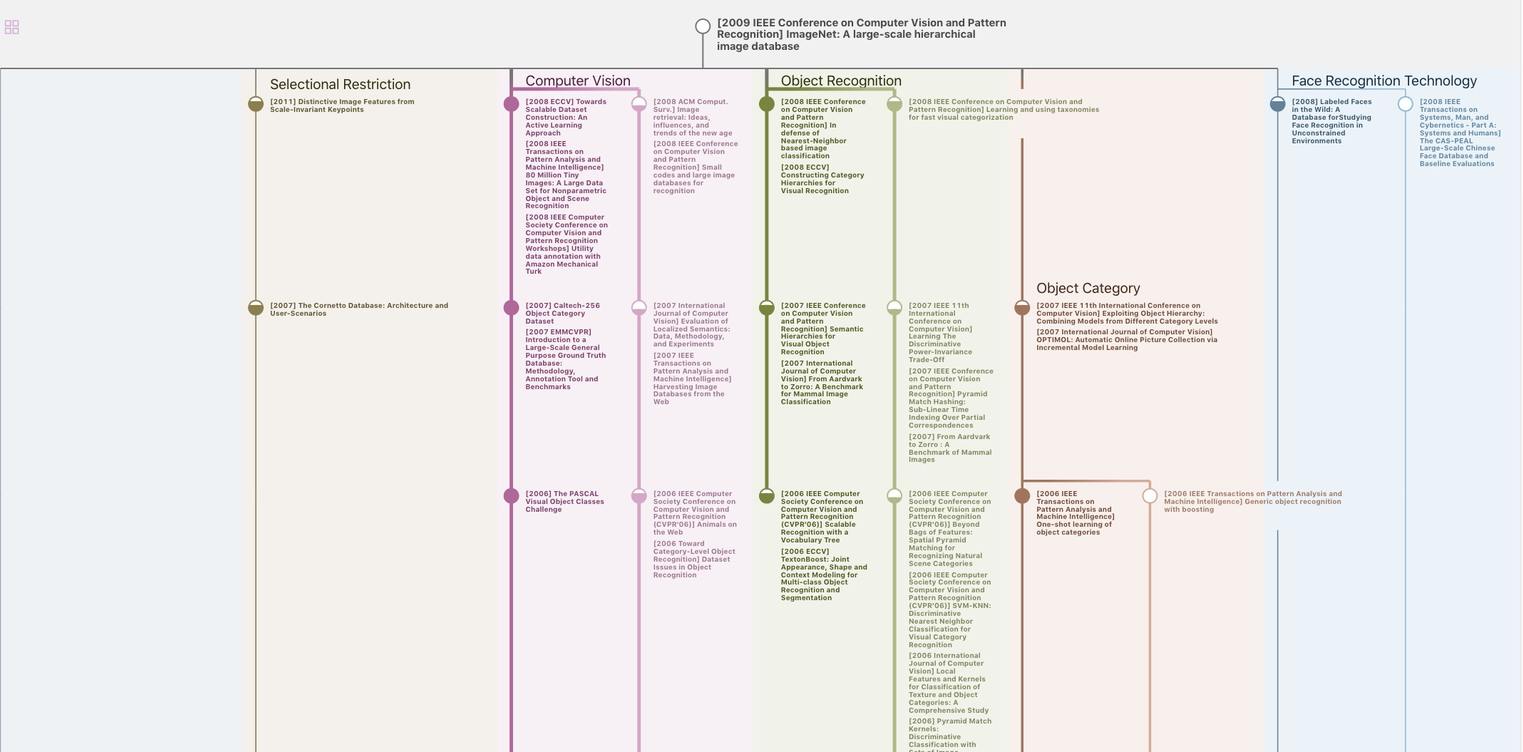
生成溯源树,研究论文发展脉络
Chat Paper
正在生成论文摘要