Feasibility of the application of deep learning-reconstructed ultra-fast respiratory-triggered T2-weighted imaging at 3 T in liver imaging
MAGNETIC RESONANCE IMAGING(2024)
摘要
Objective. The evaluate the feasibility of a novel deep learning-reconstructed ultra-fast respiratory-triggered T2WI sequence (DL-RT-T2WI) In liver imaging, compared with respiratory-triggered Arms-T2WI (Arms-RT-T2WI) and respiratory-triggered FSE-T2WI (FSE-RT-T2WI) sequences. Methods. 71 patients with liver lesions underwent 3-T MRI and were prospectively enrolled. Two readers independently analyzed images acquired with DL-RT-T2WI, Arms-RT-T2WI, and FSE-RT-T2WI. The qualitative evaluation indicators, including overall image quality (OIQ), sharpness, noise, artifacts, lesion detectability (LC), lesion characterization (LD), cardiacmotion-related signal loss (CSL), and diagnostic confidence (DC), were evaluated in two readers, and further statistically compared using paired Wilcoxon rank-sum test among three sequences. Results. 176 lesions were detected in DL-RT-T2W and Arms-RT-T2WI, and 175 were detected in FSE-RT-T2WI. The acquisition time of DL-RT-T2WI was improved by 4.8-7.9 folds compared to the other two sequences. The OIQ was scored highest for DL-RT-T2WI (R1, 4.61 +/- 0.52 and R2, 4.62 +/- 0.49), was significantly superior to Arms-RT-T2WI (R1, 4.30 +/- 0.66 and R2, 4.34 +/- 0.69) and FSE-RT-T2WI (R1, 3.65 +/- 1.08 and R2, 3.75 +/- 1.01). Artifacts and sharpness scored highest for DL-RT-T2WI, followed by Arms-RT-T2WI, and were lowest for FSE-RT-T2WI in both two readers. Noise and CSL for DL-RT-T2WI scored similar to Arms-RT-T2WI (P > 0.05) and were significantly superior to FSE-RT-T2WI (P < 0.001). Both LD and LC for DL-RT-T2WI were significantly superior to Arms-RT-T2WI and FSE-RT-T2WI in two readers (P < 0.001). DC for DL-RT-T2WI scored best, significantly superior to Arms-RT-T2WI (P < 0.010) and FSE-RT-T2WI (P < 0.001). Conclusions. The novel ultra-fast DL-RT-T2WI is feasible for liver imaging and lesion characterization and diagnosis, not only offers a significant improvement in acquisition time but also outperforms Arms-RT-T2WI and FSE-RT-T2WI concerning image quality and DC.
更多查看译文
关键词
Deep learning,Respiratory-triggered,Liver,T2-weighted imaging
AI 理解论文
溯源树
样例
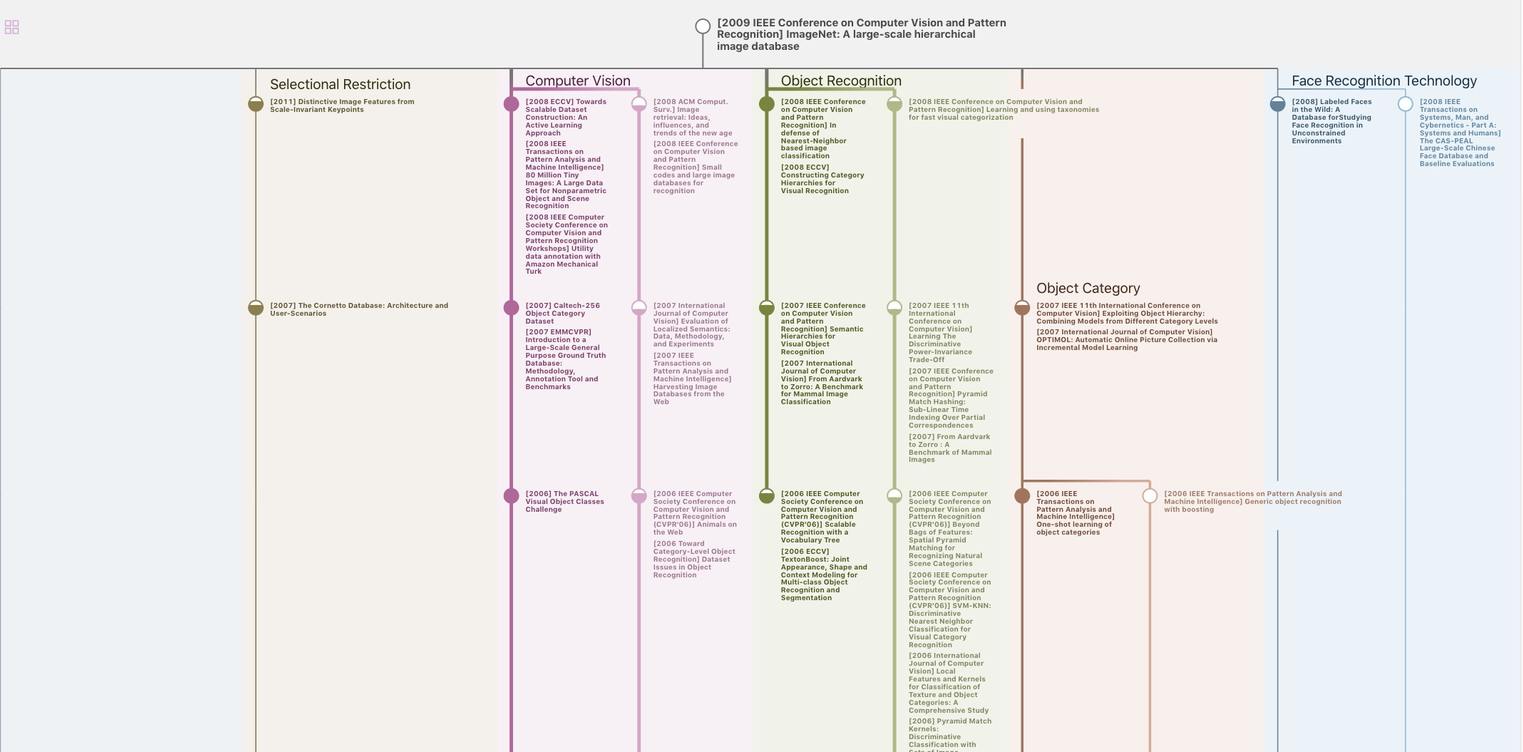
生成溯源树,研究论文发展脉络
Chat Paper
正在生成论文摘要