Accelerating fourth-generation machine learning potentials by quasi-linear scaling particle mesh charge equilibration
arxiv(2024)
摘要
Machine learning potentials (MLP) have revolutionized the field of atomistic
simulations by describing the atomic interactions with the accuracy of
electronic structure methods at a small fraction of the costs. Most current
MLPs construct the energy of a system as a sum of atomic energies, which depend
on information about the atomic environments provided in form of predefined or
learnable feature vectors. If, in addition, non-local phenomena like long-range
charge transfer are important, fourth-generation MLPs need to be used, which
include a charge equilibration (Qeq) step to take the global structure of the
system into account. This Qeq can significantly increase the computational cost
and thus can become the computational bottleneck for large systems. In this
paper we present a highly efficient formulation of Qeq that does not require
the explicit computation of the Coulomb matrix elements resulting in a
quasi-linearly scaling method. Moreover, our approach also allows for the
efficient calculation of energy derivatives, which explicitly consider the
global structure-dependence of the atomic charges as obtained from Qeq. Due to
its generality, the method is not restricted to MLPs but can also be applied
within a variety of other force fields.
更多查看译文
AI 理解论文
溯源树
样例
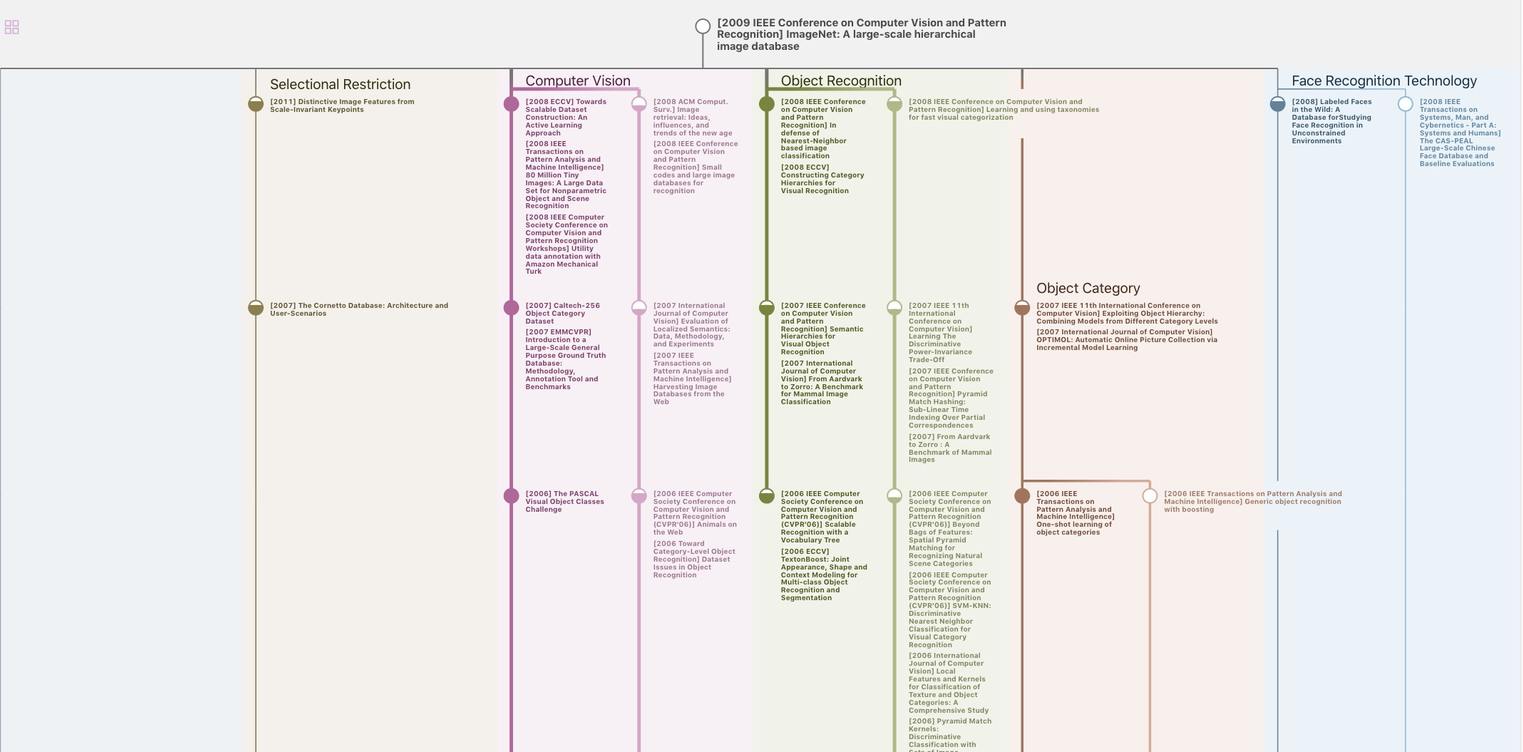
生成溯源树,研究论文发展脉络
Chat Paper
正在生成论文摘要