Harnessing Intra-group Variations Via a Population-Level Context for Pathology Detection
arxiv(2024)
摘要
Realizing sufficient separability between the distributions of healthy and
pathological samples is a critical obstacle for pathology detection
convolutional models. Moreover, these models exhibit a bias for contrast-based
images, with diminished performance on texture-based medical images. This study
introduces the notion of a population-level context for pathology detection and
employs a graph theoretic approach to model and incorporate it into the latent
code of an autoencoder via a refinement module we term PopuSense. PopuSense
seeks to capture additional intra-group variations inherent in biomedical data
that a local or global context of the convolutional model might miss or smooth
out. Experiments on contrast-based and texture-based images, with minimal
adaptation, encounter the existing preference for intensity-based input.
Nevertheless, PopuSense demonstrates improved separability in contrast-based
images, presenting an additional avenue for refining representations learned by
a model.
更多查看译文
AI 理解论文
溯源树
样例
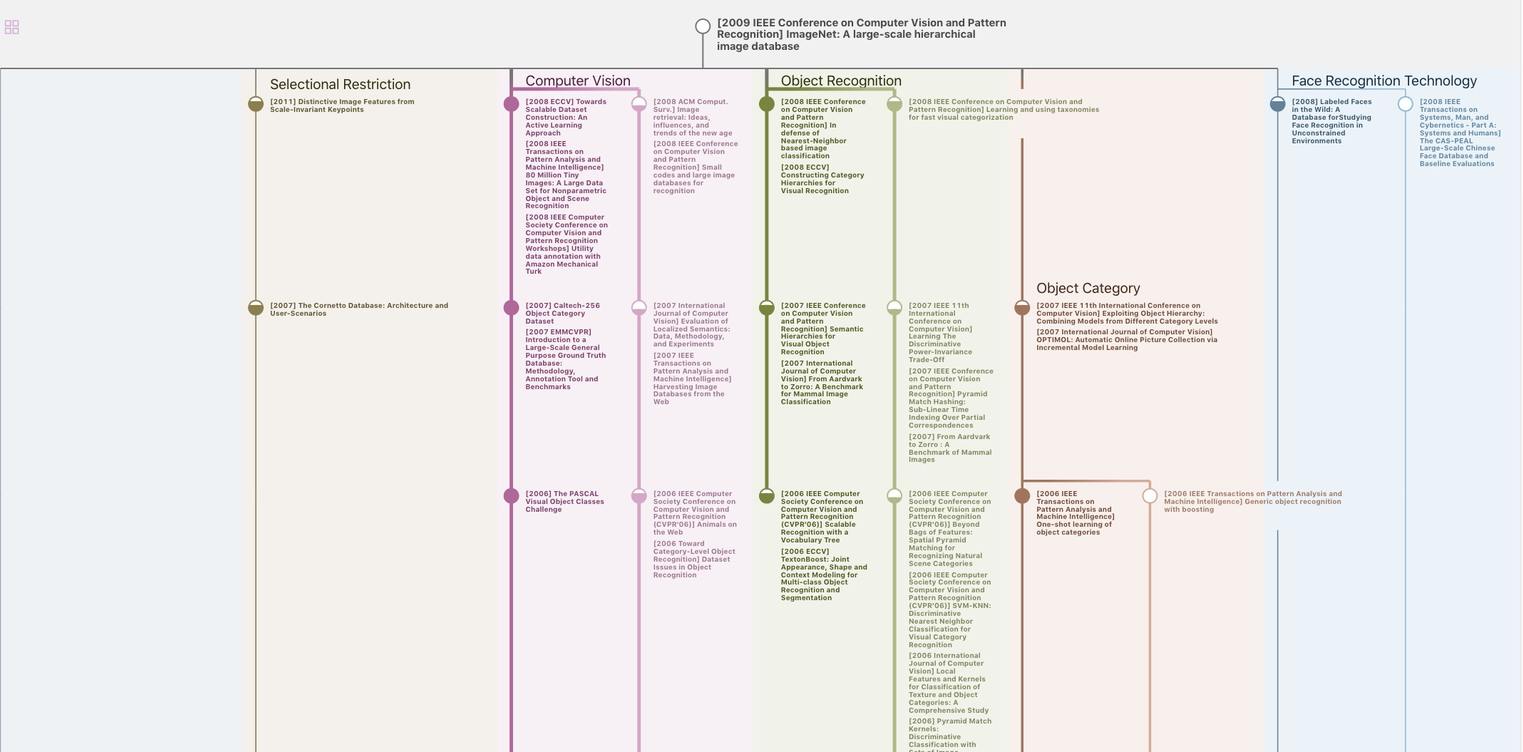
生成溯源树,研究论文发展脉络
Chat Paper
正在生成论文摘要