LUM-ViT: Learnable Under-sampling Mask Vision Transformer for Bandwidth Limited Optical Signal Acquisition
ICLR 2024(2024)
摘要
Bandwidth constraints during signal acquisition frequently impede real-time
detection applications. Hyperspectral data is a notable example, whose vast
volume compromises real-time hyperspectral detection. To tackle this hurdle, we
introduce a novel approach leveraging pre-acquisition modulation to reduce the
acquisition volume. This modulation process is governed by a deep learning
model, utilizing prior information. Central to our approach is LUM-ViT, a
Vision Transformer variant. Uniquely, LUM-ViT incorporates a learnable
under-sampling mask tailored for pre-acquisition modulation. To further
optimize for optical calculations, we propose a kernel-level weight
binarization technique and a three-stage fine-tuning strategy. Our evaluations
reveal that, by sampling a mere 10
maintains the accuracy loss within 1.8
The method sustains near-original accuracy when implemented on real-world
optical hardware, demonstrating its practicality. Code will be available at
https://github.com/MaxLLF/LUM-ViT.
更多查看译文
关键词
hyperspectral imaging,optical modulation,real-time detection,vision transformer,pre-acquisition modulation,learnable mask,weight binarization
AI 理解论文
溯源树
样例
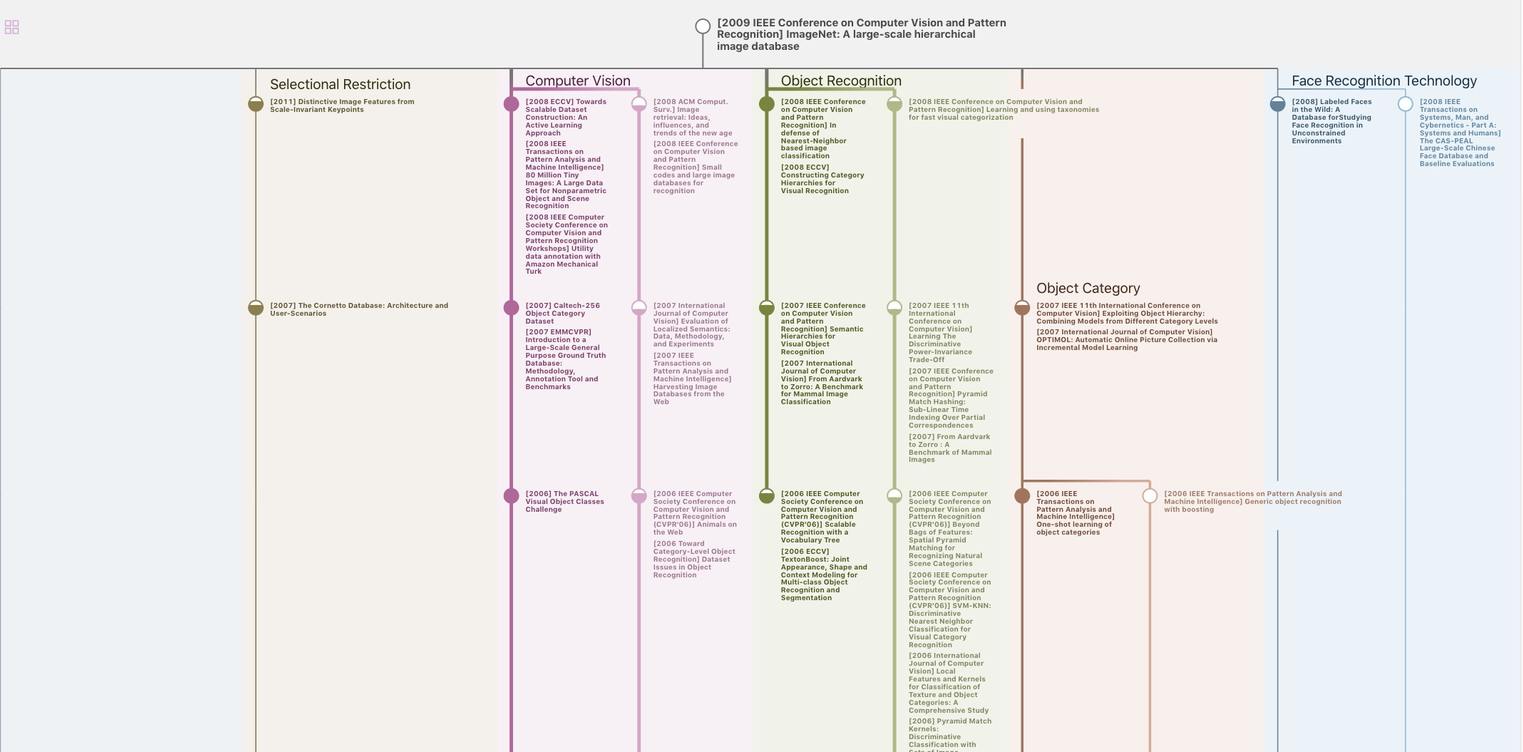
生成溯源树,研究论文发展脉络
Chat Paper
正在生成论文摘要