Unsigned Orthogonal Distance Fields: An Accurate Neural Implicit Representation for Diverse 3D Shapes
CVPR 2024(2024)
Abstract
Neural implicit representation of geometric shapes has witnessed considerable
advancements in recent years. However, common distance field based implicit
representations, specifically signed distance field (SDF) for watertight shapes
or unsigned distance field (UDF) for arbitrary shapes, routinely suffer from
degradation of reconstruction accuracy when converting to explicit surface
points and meshes. In this paper, we introduce a novel neural implicit
representation based on unsigned orthogonal distance fields (UODFs). In UODFs,
the minimal unsigned distance from any spatial point to the shape surface is
defined solely in one orthogonal direction, contrasting with the
multi-directional determination made by SDF and UDF. Consequently, every point
in the 3D UODFs can directly access its closest surface points along three
orthogonal directions. This distinctive feature leverages the accurate
reconstruction of surface points without interpolation errors. We verify the
effectiveness of UODFs through a range of reconstruction examples, extending
from simple watertight or non-watertight shapes to complex shapes that include
hollows, internal or assembling structures.
MoreTranslated text
AI Read Science
Must-Reading Tree
Example
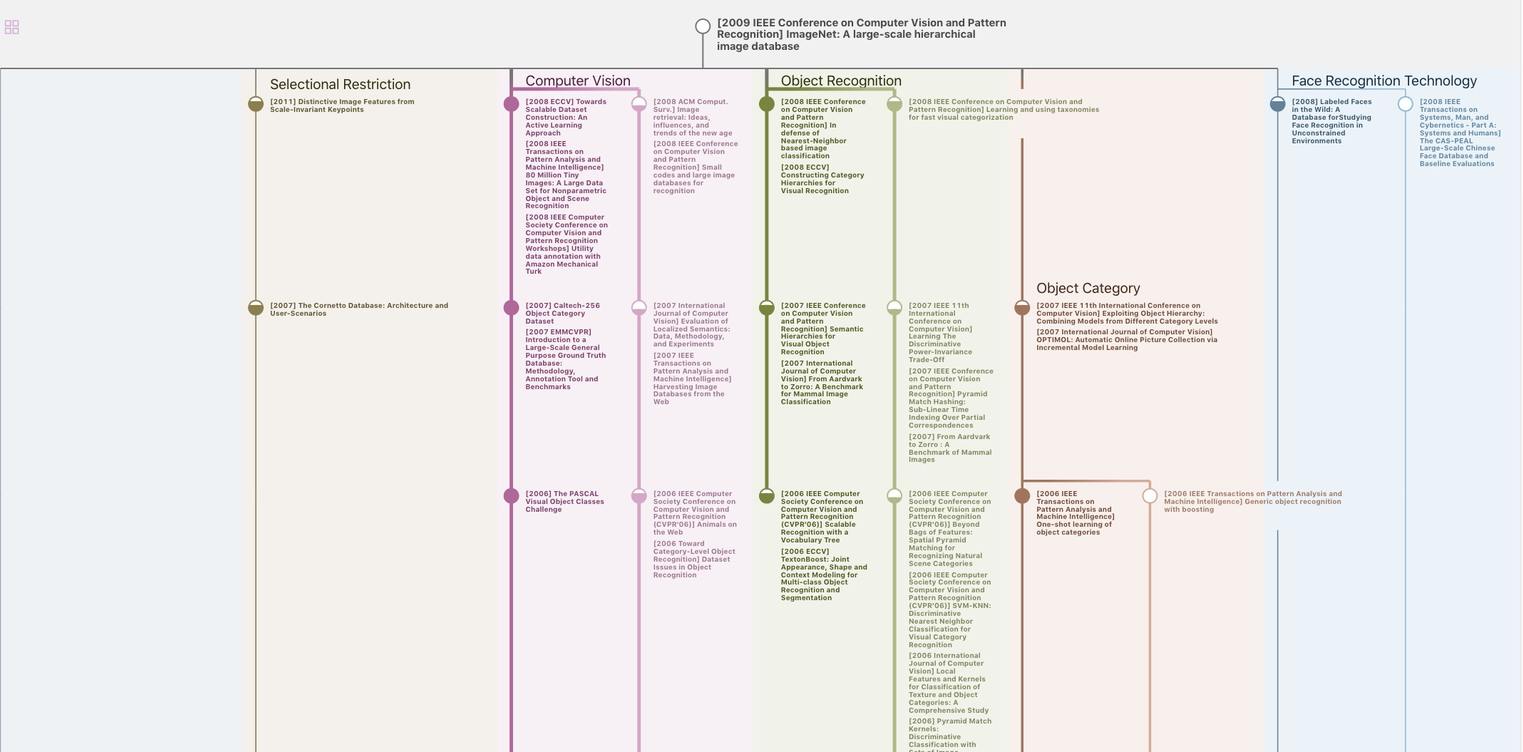
Generate MRT to find the research sequence of this paper
Chat Paper
Summary is being generated by the instructions you defined