KeNet: Knowledge-enhanced Doc-Label Attention Network for Multi-label Text Classification.
IEEE International Conference on Acoustics, Speech, and Signal Processing(2024)
摘要
Multi-Label Text Classification (MLTC) is a fundamental task in the field ofNatural Language Processing (NLP) that involves the assignment of multiplelabels to a given text. MLTC has gained significant importance and has beenwidely applied in various domains such as topic recognition, recommendationsystems, sentiment analysis, and information retrieval. However, traditionalmachine learning and Deep neural network have not yet addressed certain issues,such as the fact that some documents are brief but have a large number oflabels and how to establish relationships between the labels. It is imperativeto additionally acknowledge that the significance of knowledge is substantiatedin the realm of MLTC. To address this issue, we provide a novel approach knownas Knowledge-enhanced Doc-Label Attention Network (KeNet). Specifically, wedesign an Attention Network that incorporates external knowledge, labelembedding, and a comprehensive attention mechanism. In contrast to conventionalmethods, we use comprehensive representation of documents, knowledge and labelsto predict all labels for each single text. Our approach has been validated bycomprehensive research conducted on three multi-label datasets. Experimentalresults demonstrate that our method outperforms state-of-the-art MLTC method.Additionally, a case study is undertaken to illustrate the practicalimplementation of KeNet.
更多查看译文
关键词
Multi-Label Text Classification,Natural Language Processing
AI 理解论文
溯源树
样例
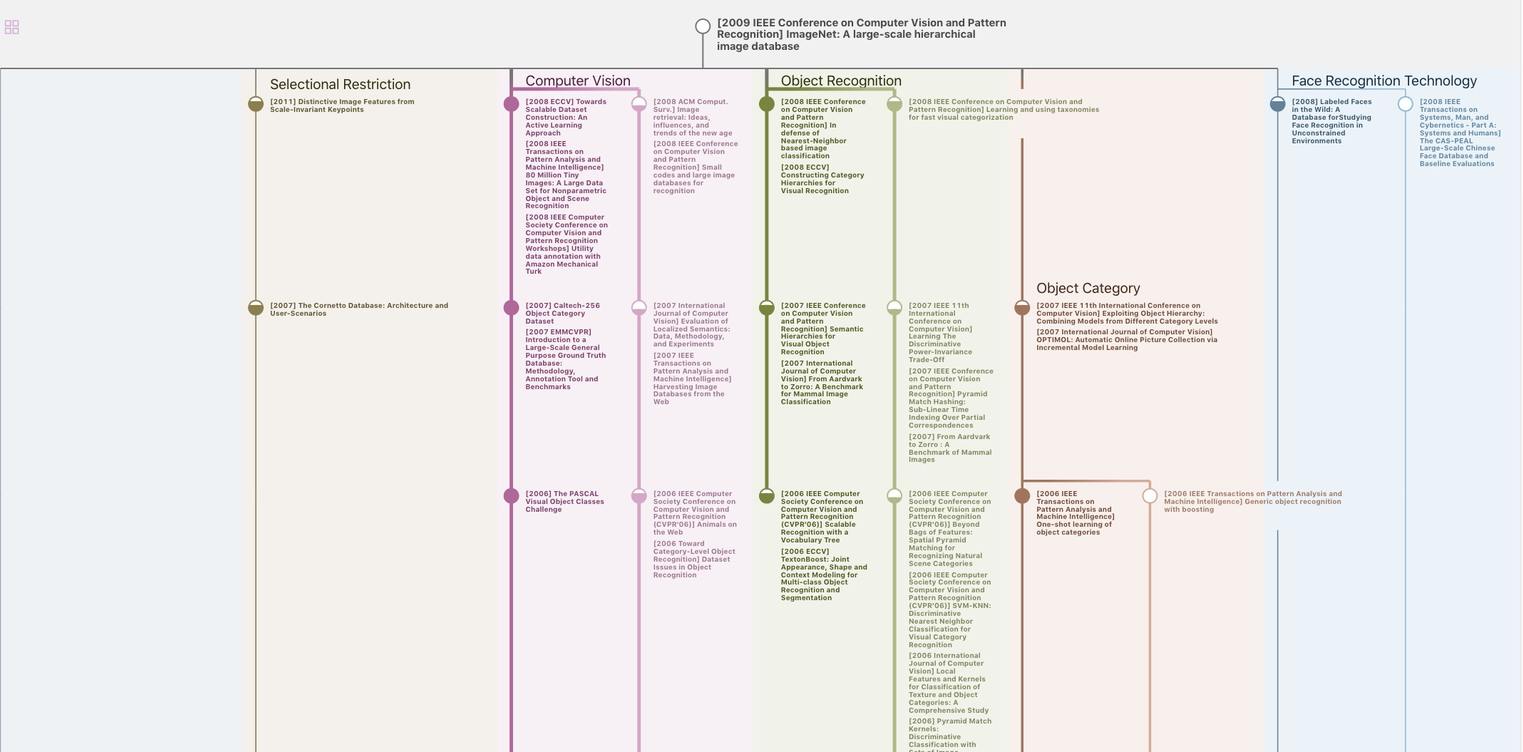
生成溯源树,研究论文发展脉络
Chat Paper
正在生成论文摘要