Modality-Aware and Shift Mixer for Multi-modal Brain Tumor Segmentation
CoRR(2024)
摘要
Combining images from multi-modalities is beneficial to explore various
information in computer vision, especially in the medical domain. As an
essential part of clinical diagnosis, multi-modal brain tumor segmentation aims
to delineate the malignant entity involving multiple modalities. Although
existing methods have shown remarkable performance in the task, the information
exchange for cross-scale and high-level representations fusion in spatial and
modality are limited in these methods. In this paper, we present a novel
Modality Aware and Shift Mixer that integrates intra-modality and
inter-modality dependencies of multi-modal images for effective and robust
brain tumor segmentation. Specifically, we introduce a Modality-Aware module
according to neuroimaging studies for modeling the specific modality pair
relationships at low levels, and a Modality-Shift module with specific mosaic
patterns is developed to explore the complex relationships across modalities at
high levels via the self-attention. Experimentally, we outperform previous
state-of-the-art approaches on the public Brain Tumor Segmentation (BraTS 2021
segmentation) dataset. Further qualitative experiments demonstrate the efficacy
and robustness of MASM.
更多查看译文
AI 理解论文
溯源树
样例
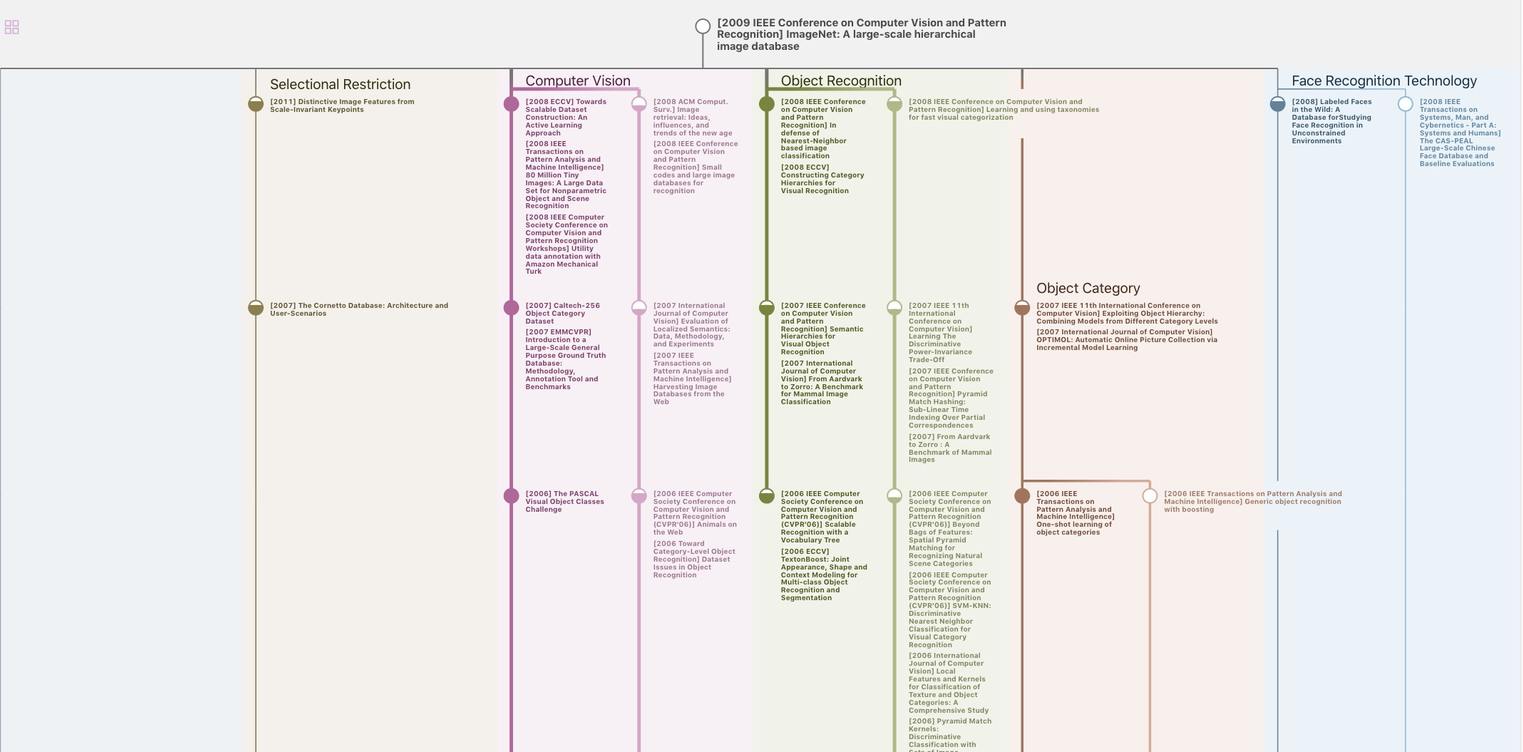
生成溯源树,研究论文发展脉络
Chat Paper
正在生成论文摘要