VTG-GPT: Tuning-Free Zero-Shot Video Temporal Grounding with GPT
APPLIED SCIENCES-BASEL(2024)
摘要
Video temporal grounding (VTG) aims to locate specific temporal segments from an untrimmed video based on a linguistic query. Most existing VTG models are trained on extensive annotated video-text pairs, a process that not only introduces human biases from the queries but also incurs significant computational costs. To tackle these challenges, we propose VTG-GPT, a GPT-based method for zero-shot VTG without training or fine-tuning. To reduce prejudice in the original query, we employ Baichuan2 to generate debiased queries. To lessen redundant information in videos, we apply MiniGPT-v2 to transform visual content into more precise captions. Finally, we devise the proposal generator and post-processing to produce accurate segments from debiased queries and image captions. Extensive experiments demonstrate that VTG-GPT significantly outperforms SOTA methods in zero-shot settings and surpasses unsupervised approaches. More notably, it achieves competitive performance comparable to supervised methods. The code is available on GitHub.
更多查看译文
关键词
video temporal grounding,generative pre-trained transformer,tuning-free strategy,query debiasing
AI 理解论文
溯源树
样例
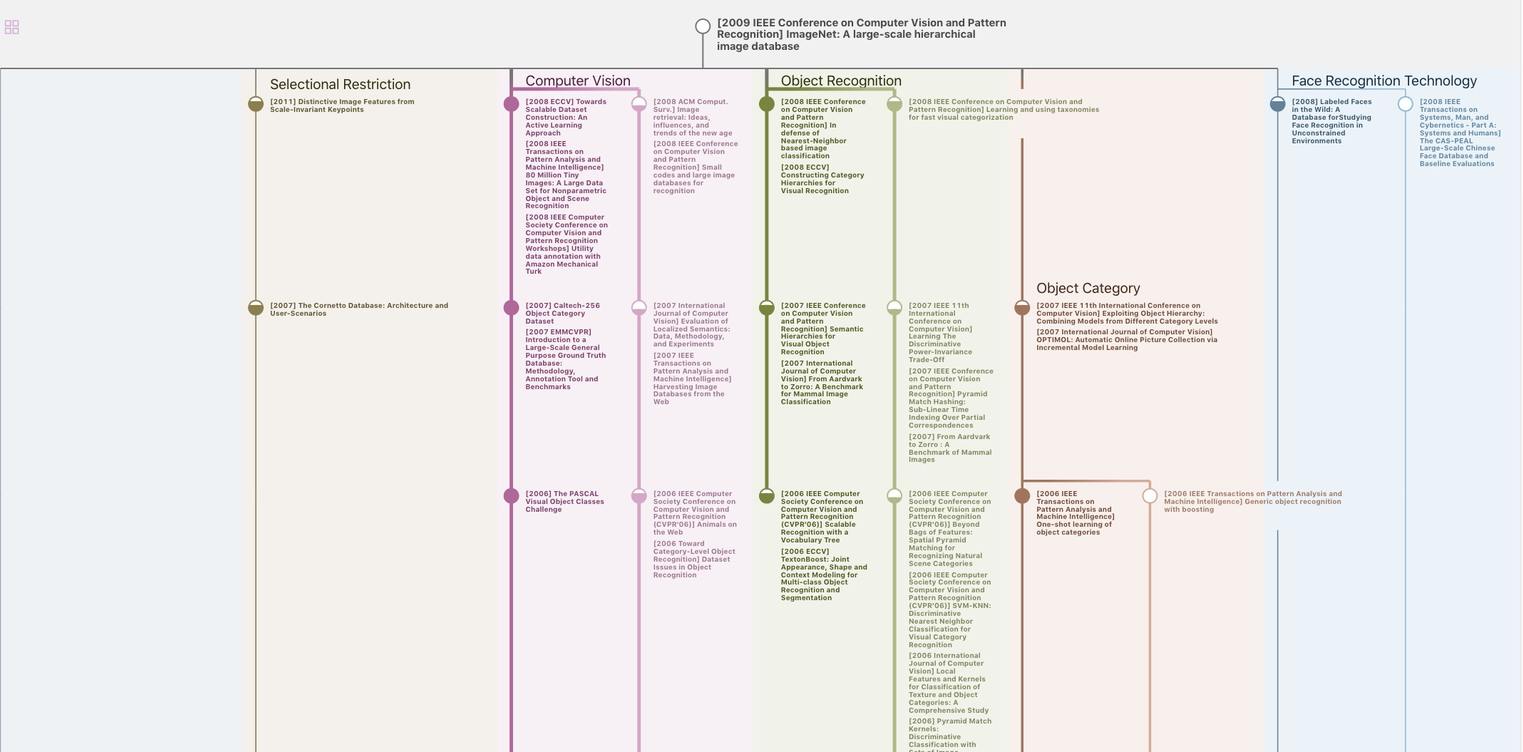
生成溯源树,研究论文发展脉络
Chat Paper
正在生成论文摘要