COMMIT: Certifying Robustness of Multi-Sensor Fusion Systems against Semantic Attacks
CoRR(2024)
Abstract
Multi-sensor fusion systems (MSFs) play a vital role as the perception module
in modern autonomous vehicles (AVs). Therefore, ensuring their robustness
against common and realistic adversarial semantic transformations, such as
rotation and shifting in the physical world, is crucial for the safety of AVs.
While empirical evidence suggests that MSFs exhibit improved robustness
compared to single-modal models, they are still vulnerable to adversarial
semantic transformations. Despite the proposal of empirical defenses, several
works show that these defenses can be attacked again by new adaptive attacks.
So far, there is no certified defense proposed for MSFs. In this work, we
propose the first robustness certification framework COMMIT certify robustness
of multi-sensor fusion systems against semantic attacks. In particular, we
propose a practical anisotropic noise mechanism that leverages randomized
smoothing with multi-modal data and performs a grid-based splitting method to
characterize complex semantic transformations. We also propose efficient
algorithms to compute the certification in terms of object detection accuracy
and IoU for large-scale MSF models. Empirically, we evaluate the efficacy of
COMMIT in different settings and provide a comprehensive benchmark of certified
robustness for different MSF models using the CARLA simulation platform. We
show that the certification for MSF models is at most 48.39
of single-modal models, which validates the advantages of MSF models. We
believe our certification framework and benchmark will contribute an important
step towards certifiably robust AVs in practice.
MoreTranslated text
AI Read Science
Must-Reading Tree
Example
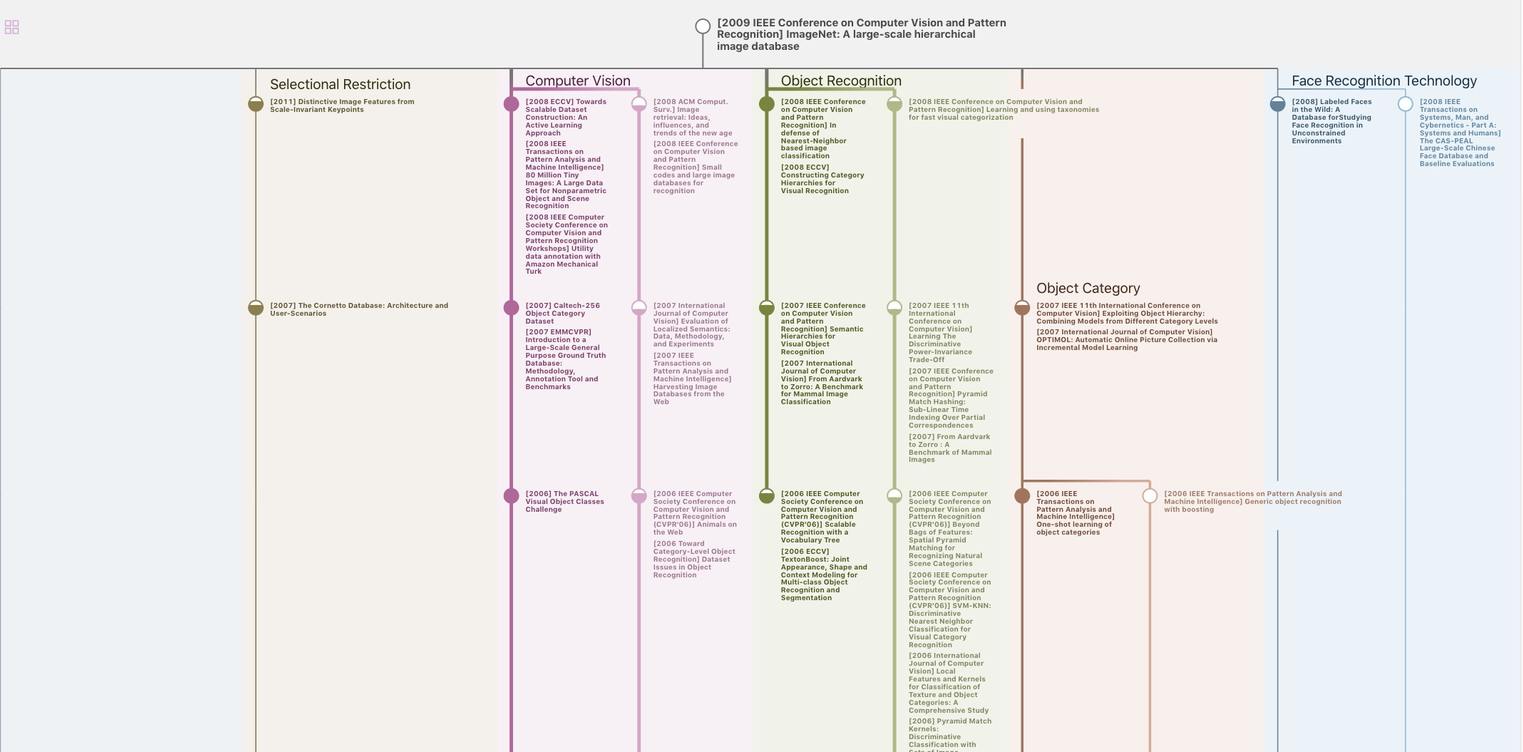
Generate MRT to find the research sequence of this paper
Chat Paper
Summary is being generated by the instructions you defined