Aggregating Global and Local Representations via Hybrid Transformer for Video Deraining
IEEE Transactions on Circuits and Systems for Video Technology(2024)
摘要
Although video deraining technology has achieved great success in recent years, extracting spatiotemporal feature representations across the domains of spatial and temporal in successive frames, then performing spatial and temporal modeling, and restoring high-quality deraining videos with rich details are still challenging tasks. In this paper, we use the hybrid Transformer for the first attempt in video rain removal tasks, and propose a novel video deraining network based on hybrid transformer (VDN-HT) to aggregate global and local representations to accomplish video deraining. In the feature extraction process, we propose to use a U-shaped structure based on serial Transformer blocks to extract shallow local features, deep global features and global dependencies, and then adaptively aggregate them to obtain rainy video features with rain streaks of different directions and densities. In order to better model spatiotemporal relationships, the VDN-HT uses the Transformer’s long-range and relational modeling abilities to obtain the features of spatial and the correlations of temporal between continuous video frames to achieve multi-frame alignment. For ensuring the global-local consistency of the reconstructed frames, we design a global-local reconstruction module composed of Transformer and convolutional neural network (CNN) in parallel to aggregate global and local information to better reconstruct each frame. In addition, the proposed gating-based refinement module and color loss effectively retain the details and color information after removing rain streaks. Extensive experiments on NTURain, RainSynLight25 and RainSynHeavy25 datasets have shown that the VDN-HT can handle many types of rainy videos and perform better than previous methods.
更多查看译文
关键词
Video deraining,Hybrid transformer,Global and local representations,VDN-HT
AI 理解论文
溯源树
样例
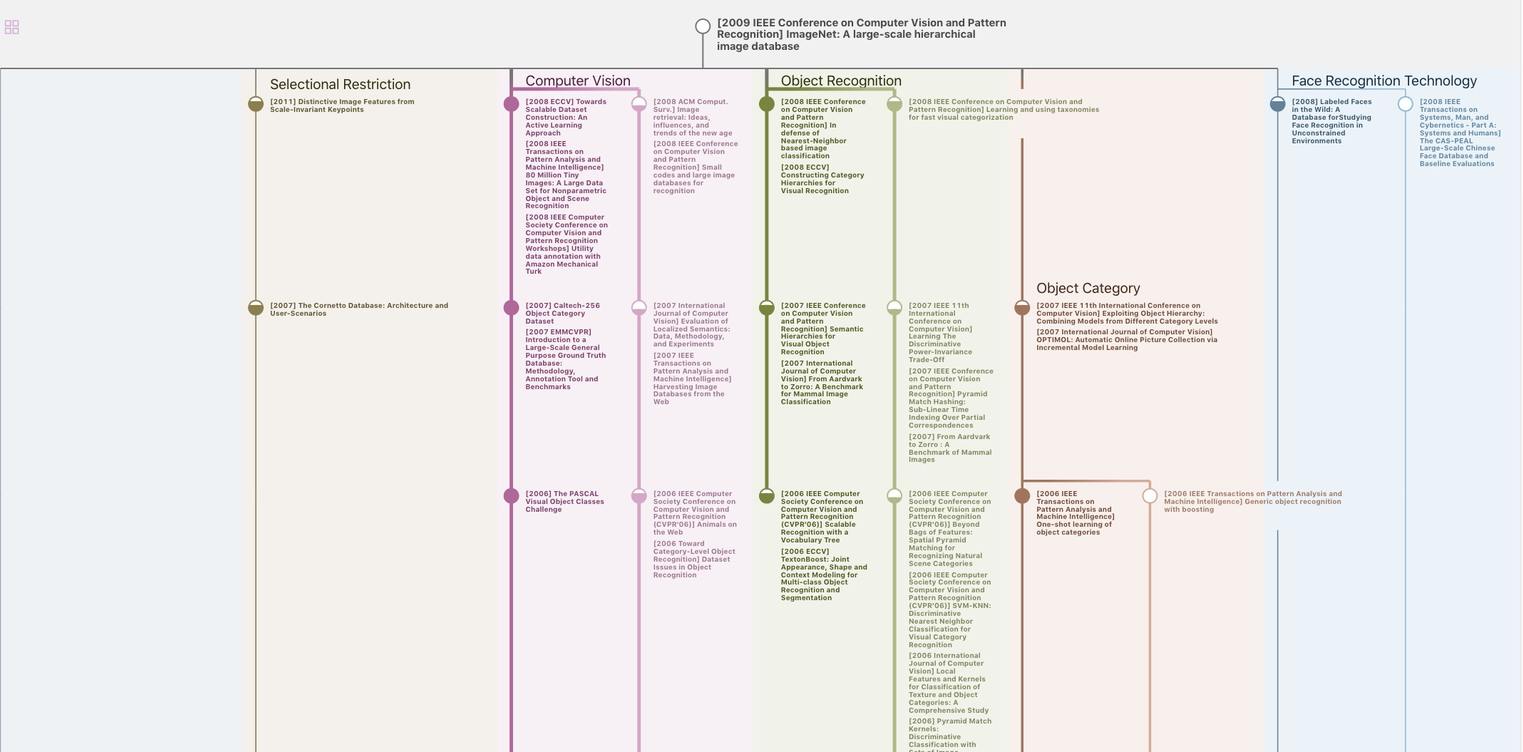
生成溯源树,研究论文发展脉络
Chat Paper
正在生成论文摘要