Exploring Hierarchical Information in Hyperbolic Space for Self-Supervised Image Hashing.
IEEE transactions on image processing : a publication of the IEEE Signal Processing Society(2024)
摘要
In real-world datasets, visually related images often form clusters, and these clusters can be further grouped into larger categories with more general semantics. These inherent hierarchical structures can help capture the underlying distribution of data, making it easier to learn robust hash codes that lead to better retrieval performance. However, existing methods fail to make use of this hierarchical information, which in turn prevents the accurate preservation of relationships between data points in the learned hash codes, resulting in suboptimal performance. In this paper, our focus is on applying visual hierarchical information to self-supervised hash learning and addressing three key challenges, including the construction, embedding, and exploitation of visual hierarchies. We propose a new self-supervised hashing method named Hierarchical Hyperbolic Contrastive Hashing (HHCH), making breakthroughs in three aspects. First, we propose to embed continuous hash codes into hyperbolic space for accurate semantic expression since embedding hierarchies in the hyperbolic space generates less distortion than in the hyper-sphere or Euclidean space. Second, we update the K-Means algorithm to make it run in the hyperbolic space. The proposed hierarchical hyperbolic K-Means algorithm can achieve the adaptive construction of hierarchical semantic structures. Last but not least, to exploit the hierarchical semantic structures in hyperbolic space, we propose the hierarchical contrastive learning algorithm, including hierarchical instance-wise and hierarchical prototype-wise contrastive learning. Extensive experiments on four benchmark datasets demonstrate that the proposed method outperforms state-of-the-art self-supervised hashing methods. Our codes are released at https://github.com/HUST-IDSM-AI/HHCH.git.
更多查看译文
关键词
Self-supervised hashing,contrastive learning,hyperbolic embedding,hierarchical semantic structure,K-Means
AI 理解论文
溯源树
样例
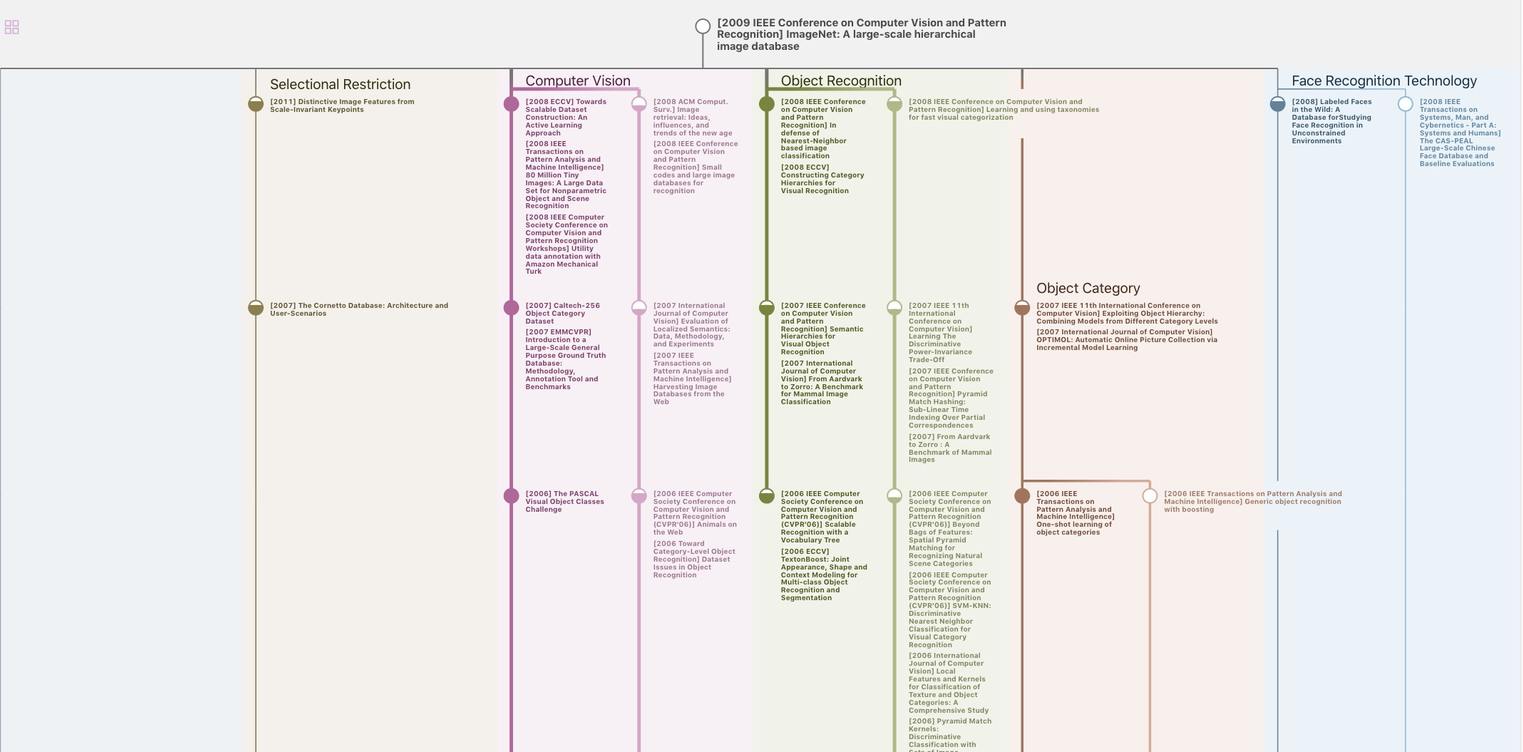
生成溯源树,研究论文发展脉络
Chat Paper
正在生成论文摘要