SDPDet: Learning Scale-Separated Dynamic Proposals for End-to-End Drone-View Detection.
IEEE Trans. Multim.(2024)
摘要
Detecting objects in large-scale drone-view images is notoriously challenging due to their uneven distribution and scale variation caused by photoing angles. Common approaches promote drone-view object detection by two-step detection (i.e., detecting sub-regions first) and multi-scale input. However, all these methods suffer from onerous computational costs since the high model complexity and input resolution. In this paper, we propose a novel one-step detector, called
SDPDet
, to enable effective object learning in drone-view images. In particular, a Scale-separated Activation Pyramid (SAP) serves to focus on the regions with objects aggregated at each scale, and a Scale-separated Learnable Proposals (SLP) mechanism learns proposal boxes and corresponding features on these regions. By such design, the quantity of learnable proposals allows dynamic adjustment at each scale separately, which facilitates the objects learning of various distributions and scales with less computational costs. Experiments demonstrate SDPDet can significantly outperform the state-of-the-art one-step detectors on three widely-used benchmarks. On the most challenging VisDrone dataset, SDPDet with ResNet50 gains
5.4%
AP and
6.9%
AP
$_{s}$
improvements while running
1.9×
faster than previous models.
更多查看译文
关键词
Drone-view image,Activation pyramid,Scale-separated learnable proposals,Object detection
AI 理解论文
溯源树
样例
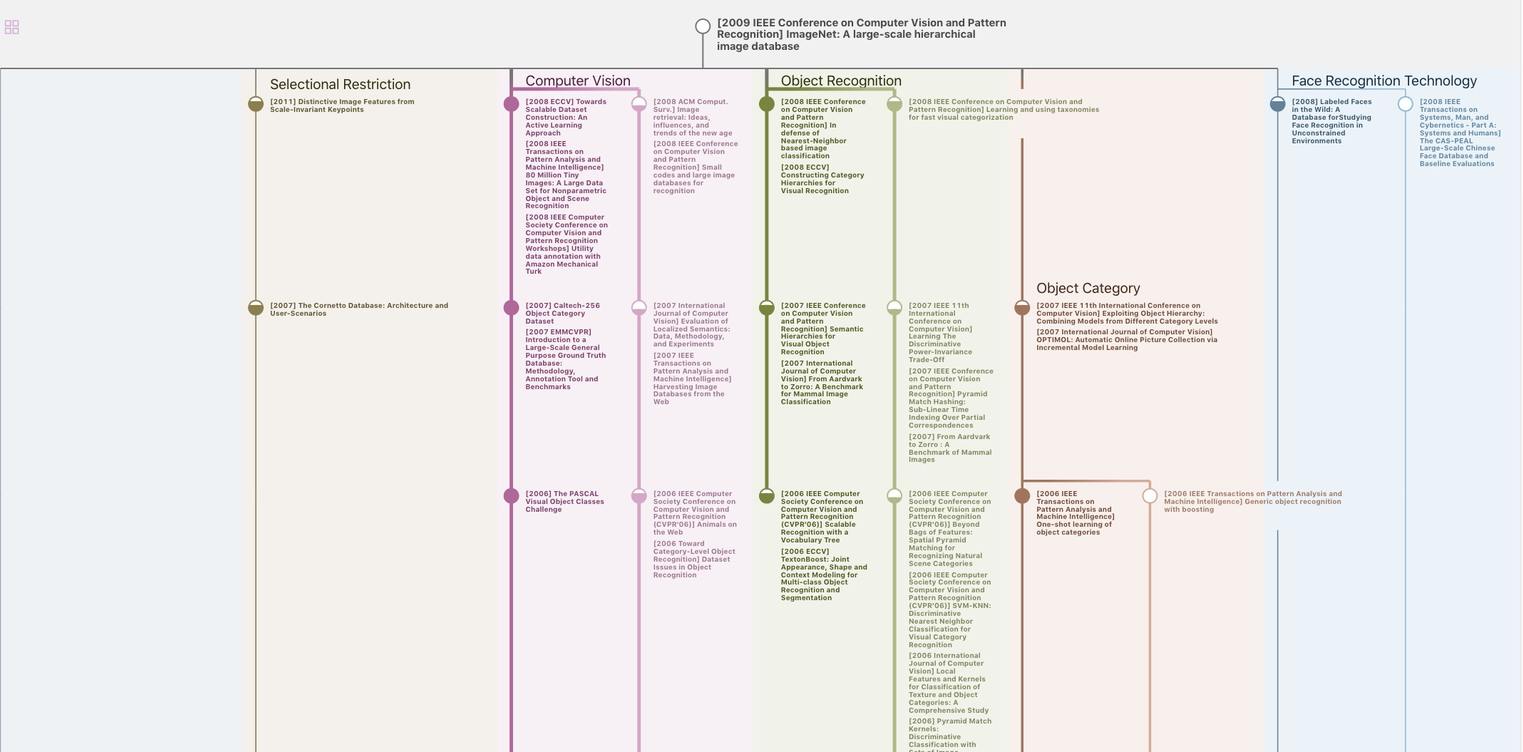
生成溯源树,研究论文发展脉络
Chat Paper
正在生成论文摘要