Comprehensive fault diagnosis in UAV-assisted sensor networks: A three-phase automated approach
Computer Communications(2024)
摘要
Ensuring operational safety in an automated working environment is a crucial requirement. In this article, we propose a technique for automated fault diagnosis in unmanned aerial vehicle-based sensor networks (UAV-sensor networks) aimed explicitly at the composite nature of different faults. Our technique consists of 3-stages: (1) UAVs collecting data from sensors and running a modified Z-score statistics to diagnose faulty readings; (2) the UAV cluster head running a multivariate analysis of variance (MANOVA) to identify data faults in cluster members; and (3) utilizing a probabilistic neural network with correlation centroids at the ground control station for further diagnosis of collected UAV data. This approach is intended for progressive improvement in fault diagnosis at different stages of data collection in a UAV-sensor network for critical applications. We conduct an extensive evaluation of our 3-stage approach on the data collected in real-time from the sensors in a physically created UAV-sensor network. The performance of the proposed modified Z-score test improves fault detection accuracy of ∼20.8%, false alarm rate of ∼54.3%, and false positive rate of ∼67.2% with the traditional and existing sensor fault detection approach. The performance of MANOVA to identify the permanent UAV data fault shows fault detection accuracy of ∼10.9%, false alarm rate of ∼44.3%, and false positive rate of ∼48.7% superior to the intermittent and transient faults. Our preliminary results suggest that the proposed approach can handle multiple composite faults and outperforms existing research results.
更多查看译文
关键词
UAV-assisted sensor networks,Automated fault diagnosis,Modified Z-score test,Multivariate analysis of variance (MANOVA) test,Probabilistic neural network (PNN)
AI 理解论文
溯源树
样例
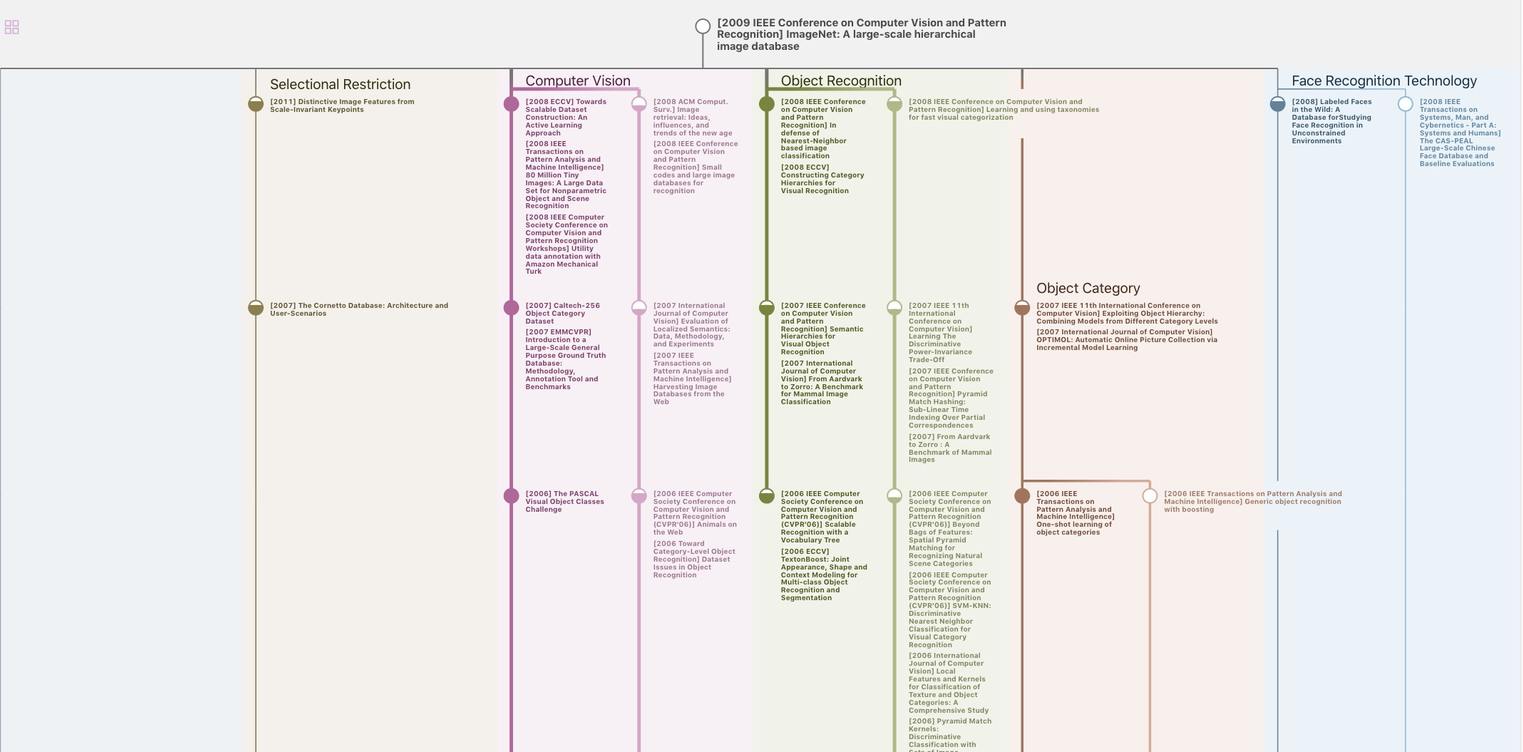
生成溯源树,研究论文发展脉络
Chat Paper
正在生成论文摘要