Similarity Learning for Land Use Scene-Level Change Detection
IEEE JOURNAL OF SELECTED TOPICS IN APPLIED EARTH OBSERVATIONS AND REMOTE SENSING(2024)
摘要
Scene-level change detection (SLCD) can provide semantic change information at image level, thus it is of great significance for monitoring land use changes. Supervised SLCD approaches tend to outperform unsupervised ones. However, the existing supervised methods rely on postclassification, which often results in unsatisfactory performance due to classification error accumulation. We therefore formulate SLCD as a similarity learning task, and propose a scene similarity learning network (SSLN) for land use SLCD. To be specific, SSLN is a two-branch network with ResNet as the backbone for feature extraction, where the global feature difference and the multiscale local feature fusion modules are considered in order to better mine the temporal correlation between bitemporal scenes. Then, the trained SSLN is further exploited to obtain the similarity of scene pairs for determining the similarity threshold via threshold traversal algorithm. Finally, the land use scenes are categorized into changed or unchanged by comparing scene similarity with the threshold. Experimental results on the publicly available MtS-WH dataset and our newly released land use scene change detection dataset show that the proposed approach achieves better performance than comparison methods, indicating that our approach is a simple yet effective solution to land use SLCD.
更多查看译文
关键词
Feature extraction,Urban areas,Training,Remote sensing,Task analysis,Semantics,Benchmark testing,Land use,scene change detection,scene similarity,similarity learning
AI 理解论文
溯源树
样例
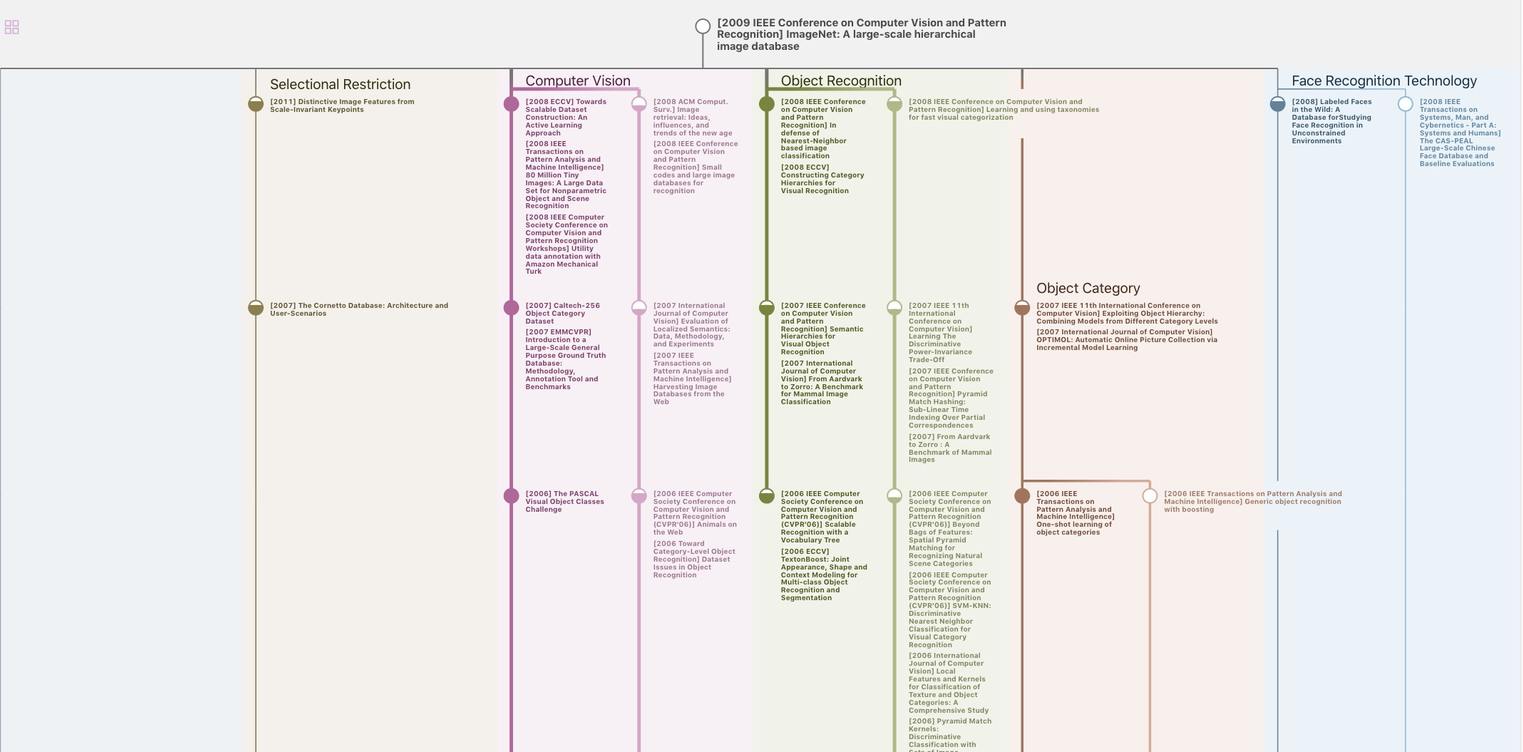
生成溯源树,研究论文发展脉络
Chat Paper
正在生成论文摘要