Spiking Autoencoder for Nonlinear Industrial Process Fault Detection
Information sciences(2024)
摘要
In recent years, artificial neural networks have been found successful applications in process monitoring within metallurgy, chemical engineering and mechanical manufacturing owing to their superior ability to construct flexible models with varying degrees of nonlinearity and effectively handle large-scale data. However, the model's distinctiveness diminishes due to the high cost associated with neural network training and initializations, resulting in a more fluctuating fault detection performance. To alleviate this problem and inspired by the way biological neurons emit spikes to transmit information, the spiking neurons are employed in the construction of a spiking neural network, introducing a shift in parameter optimization from conventional global parameter tuning to two-stage layer-wise process. Based on this, a brain-like discrete model, spiking auto-encoder (SNNAE) is constructed. The SNNAE's training process is firstly compared with that of artificial neural networks with the same structure through modeling a numerical example, results show the superior efficiency and accuracy of SNNAE in modeling highly nonlinear data. To gauge its effectiveness in fault detection, SNNAE is then compared with the state-of-art methods through the same numerical example and a three-phase flow process, indicating its ability to significantly improve process monitoring performance in nonlinear processes while notably reducing its fluctuation.
更多查看译文
关键词
Artificial neural networks,Spiking neural network,Fault detection,Nonlinear process,Performance fluctuation
AI 理解论文
溯源树
样例
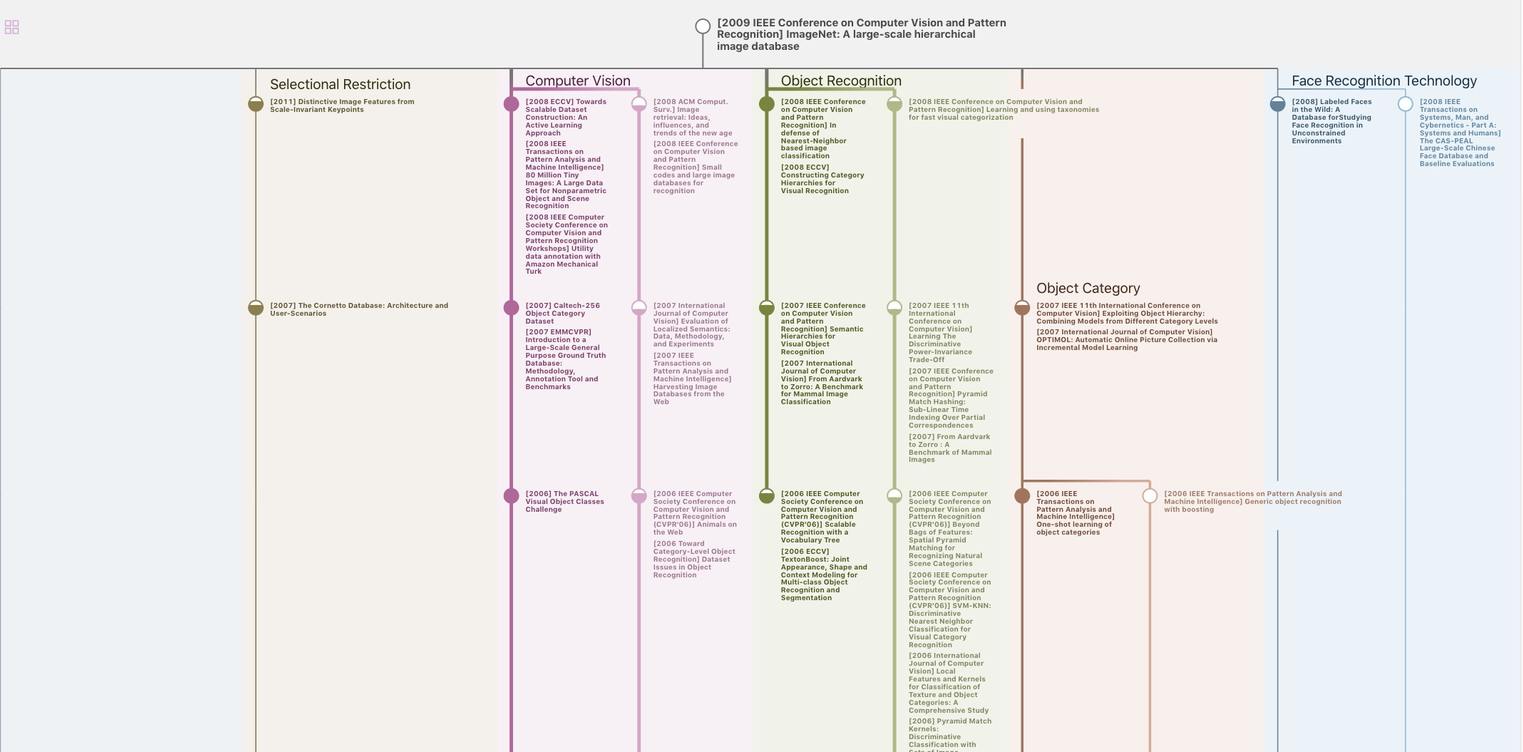
生成溯源树,研究论文发展脉络
Chat Paper
正在生成论文摘要