Optimisation of quantitative brain diffusion-relaxation MRI acquisition protocols with physics-informed machine learning
MEDICAL IMAGE ANALYSIS(2024)
摘要
Diffusion -relaxation MRI aims to extract quantitative measures that characterise microstructural tissue properties such as orientation, size, and shape, but long acquisition times are typically required. This work proposes a physics -informed learning framework to extract an optimal subset of diffusion -relaxation MRI measurements for enabling shorter acquisition times, predict non -measured signals, and estimate quantitative parameters. In vivo and synthetic brain 5D -Diffusion -T1 -T2* -weighted MRI data obtained from five healthy subjects were used for training and validation, and from a sixth participant for testing. One fully data -driven and two physicsinformed machine learning methods were implemented and compared to two manual selection procedures and Cram & eacute;r-Rao lower bound optimisation. The physics -informed approaches could identify measurement -subsets that yielded more consistently accurate parameter estimates in simulations than other approaches, with similar signal prediction error. Fivefold shorter protocols yielded error distributions of estimated quantitative parameters with very small effect sizes compared to estimates from the full protocol. Selected subsets commonly included a denser sampling of the shortest and longest inversion time, lowest echo time, and high b -value. The proposed framework combining machine learning and MRI physics offers a promising approach to develop shorter imaging protocols without compromising the quality of parameter estimates and signal predictions.
更多查看译文
关键词
Quantitative MRI,Machine learning,Brain,Diffusion-relaxation
AI 理解论文
溯源树
样例
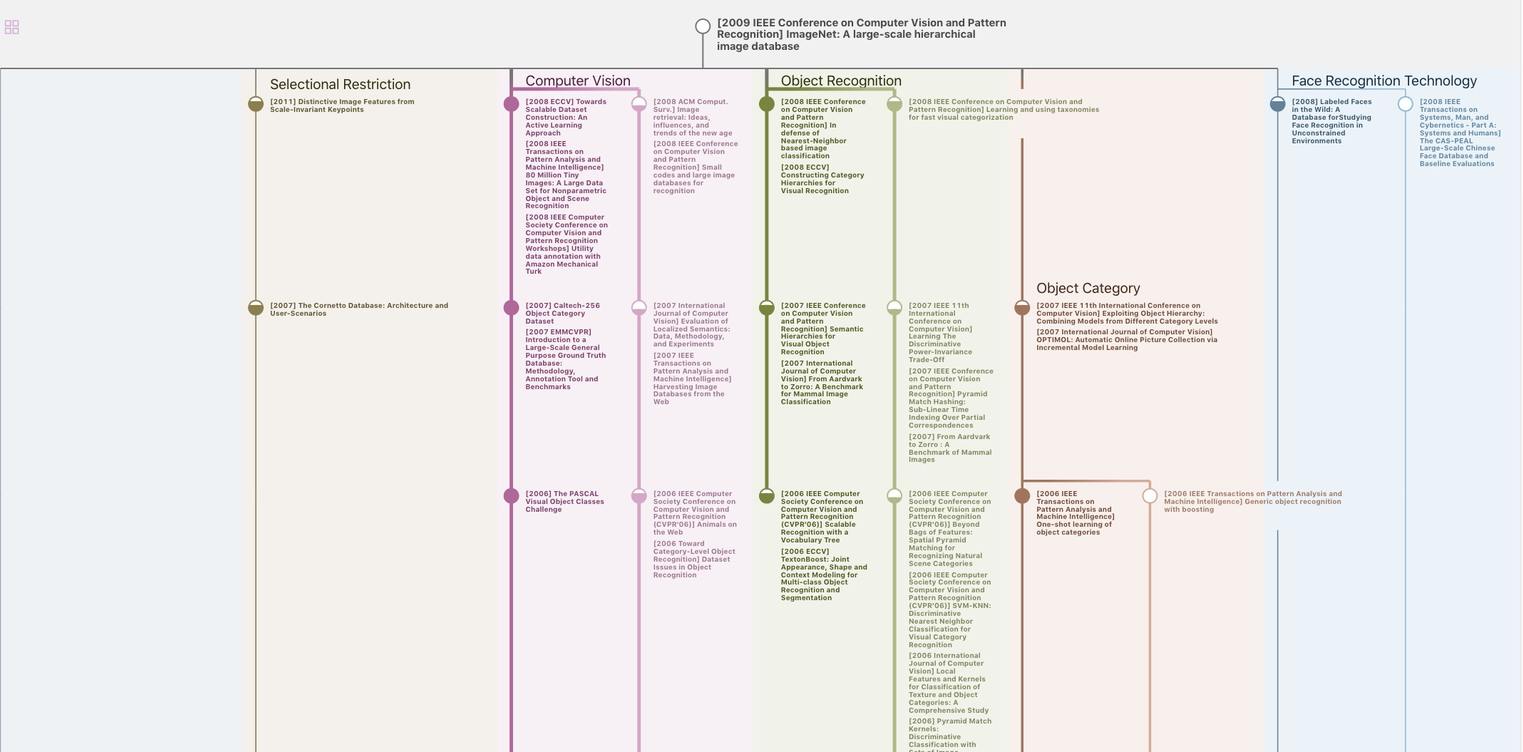
生成溯源树,研究论文发展脉络
Chat Paper
正在生成论文摘要