Artificial neural network for brain-machine interface consistently produces more naturalistic finger movements than linear methods.
bioRxiv : the preprint server for biology(2024)
摘要
Brain-machine interfaces (BMI) aim to restore function to persons living with spinal cord injuries by 'decoding' neural signals into behavior. Recently, nonlinear BMI decoders have outperformed previous state-of-the-art linear decoders, but few studies have investigated what specific improvements these nonlinear approaches provide. In this study, we compare how temporally convolved feedforward neural networks (tcFNNs) and linear approaches predict individuated finger movements in open and closed-loop settings. We show that nonlinear decoders generate more naturalistic movements, producing distributions of velocities 85.3% closer to true hand control than linear decoders. Addressing concerns that neural networks may come to inconsistent solutions, we find that regularization techniques improve the consistency of tcFNN convergence by 194.6%, along with improving average performance, and training speed. Finally, we show that tcFNN can leverage training data from multiple task variations to improve generalization. The results of this study show that nonlinear methods produce more naturalistic movements and show potential for generalizing over less constrained tasks.
Teaser:A neural network decoder produces consistent naturalistic movements and shows potential for real-world generalization through task variations.
更多查看译文
AI 理解论文
溯源树
样例
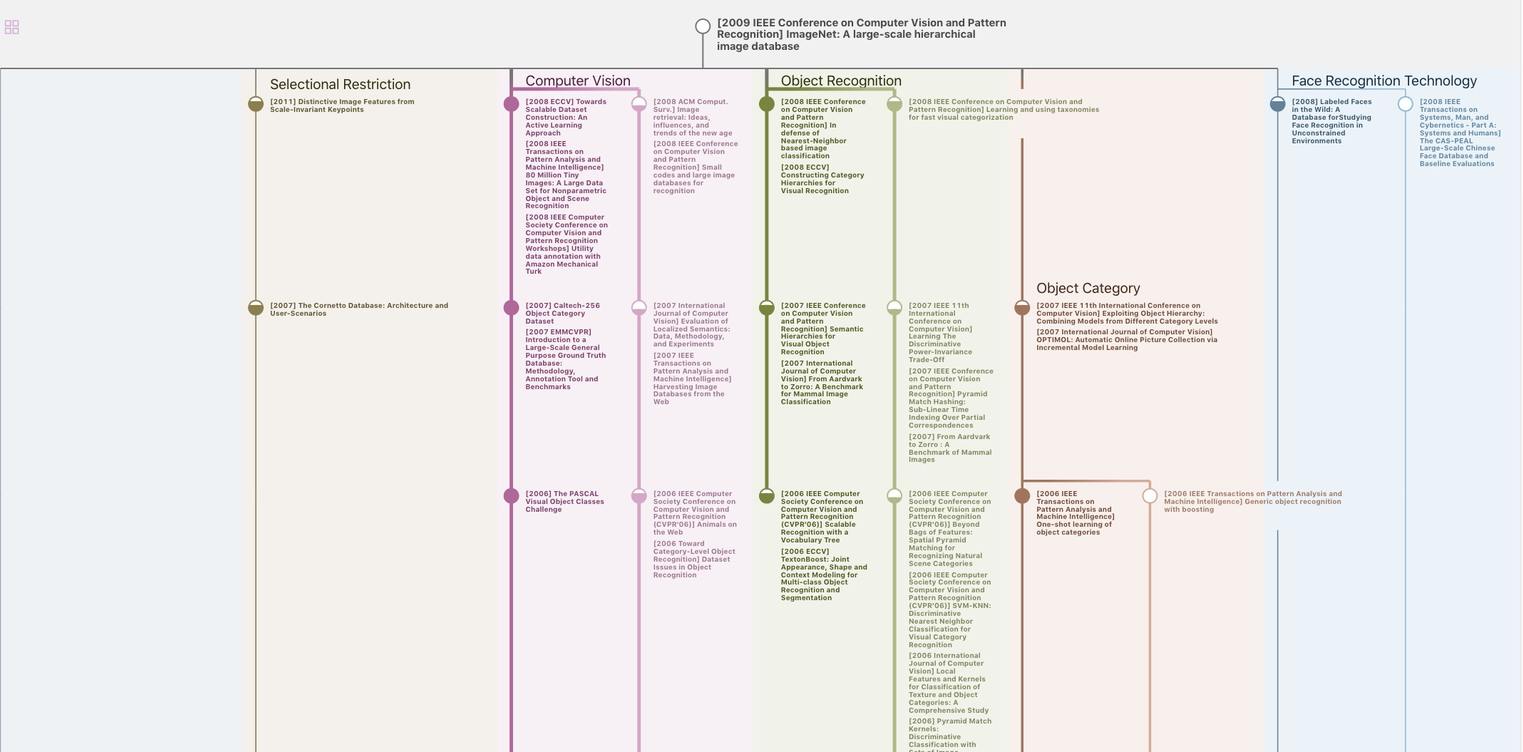
生成溯源树,研究论文发展脉络
Chat Paper
正在生成论文摘要