Denoising Sparker Seismic Data with Deep BiLSTM in Fractional Fourier Transform.
Computers & geosciences(2024)
摘要
Sparkers are widely-used sources of high-resolution images for shallow sub-bottom seismic monitoring. Sparker seismic data contain high random noise levels caused by the high voltage and electrical current required to power the sparker source. Random noise is distributed over the entire frequency and wavenumber, and is thereby difficult to suppress. Numerous studies have been conducted to develop random noise suppression by using convolutional neural networks (CNNs). However, because CNNs learn common characteristics of random noise without considering the sequence while training, learning the sequence relationship between useful signal and random noise in time-series data is difficult. As an alternative, we proposed a recurrent neural network (RNN)based denoising method that considers seismic data sequences. Effective denoising of random noise using RNNs requires features that distinguish between useful signals and random noise during network training. We proposed a denoising method for sparker seismic data that uses bidirectional long short-term memory RNN in fractional Fourier transform (FrFT). The time-frequency distribution in the FrFT domain was utilized for network training, and useful signals were effectively separated from random noise. The proposed method was applied to multichannel sparker seismic data acquired in Yeongil, Pohang (offshore eastern Korea) by the Korea Maritime & Ocean University. Numerical results confirmed that the proposed method effectively suppressed random noise while maintaining a useful signal in sparker seismic data.
更多查看译文
关键词
Denoising,Deep BiLSTM,Fractional Fourier transform,Sparker seismic data
AI 理解论文
溯源树
样例
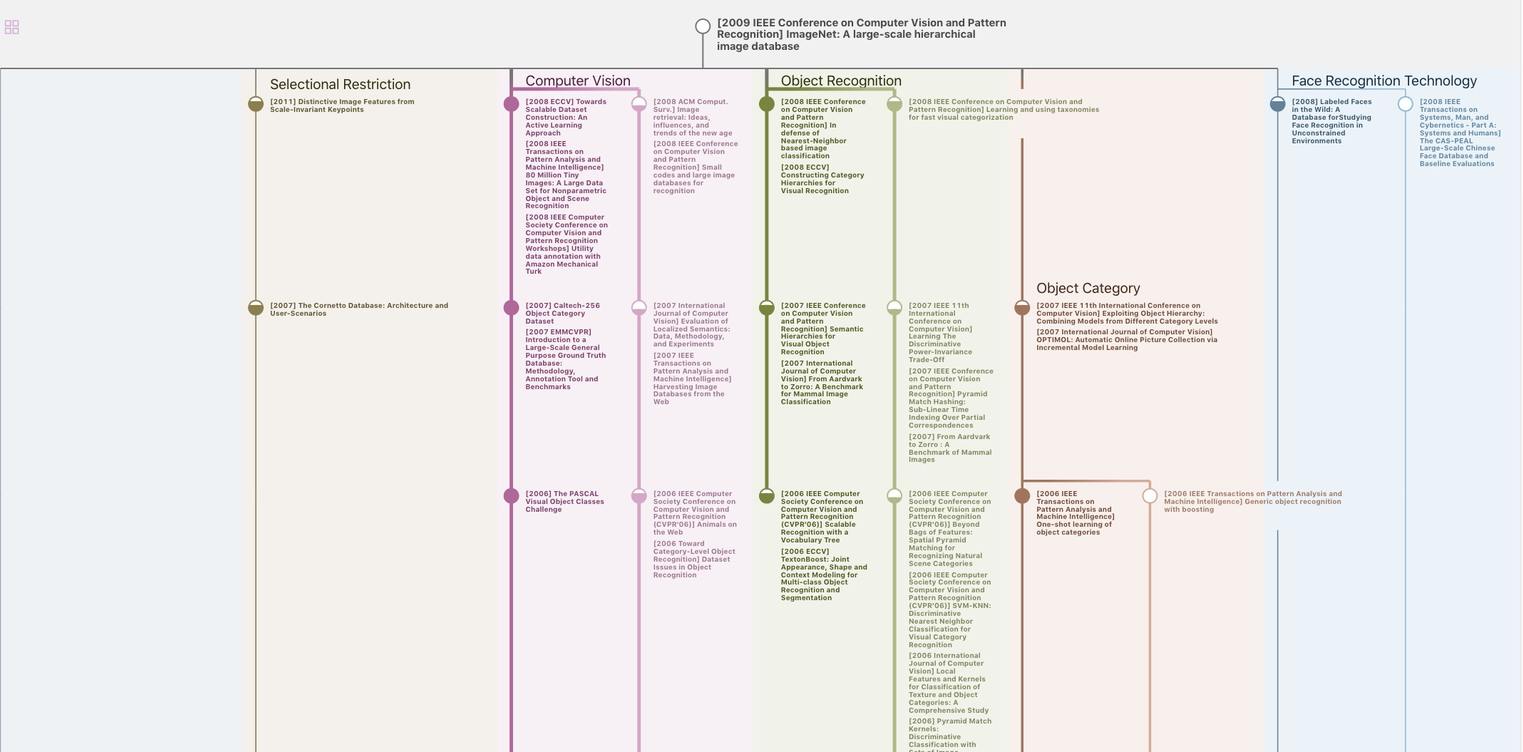
生成溯源树,研究论文发展脉络
Chat Paper
正在生成论文摘要