TerrainMesh: Metric-Semantic Terrain Reconstruction From Aerial Images Using Joint 2-D-3-D Learning
IEEE TRANSACTIONS ON ROBOTICS(2024)
摘要
This article considers outdoor terrain mapping using RGB images obtained from an aerial vehicle. While feature-based localization and mapping techniques deliver real-time vehicle odometry and sparse keypoint depth reconstruction, a dense model of the environment geometry and semantics (vegetation, buildings, etc.) is usually recovered offline with significant computation and storage. This article develops a joint 2-D-3-D learning approach to reconstruct a local metric-semantic mesh at each camera keyframe maintained by a visual odometry algorithm. Given the estimated camera trajectory, the local meshes can be assembled into a global environment model to capture the terrain topology and semantics during online operation. A local mesh is reconstructed using an initialization and refinement stage. In the initialization stage, we estimate the mesh vertex elevation by solving a least squares problem relating the vertex barycentric coordinates to the sparse keypoint depth measurements. In the refinement stage, we associate 2-D image and semantic features with the 3-D mesh vertices using camera projection and apply graph convolution to refine the mesh vertex spatial coordinates and semantic features based on joint 2-D and 3-D supervision. Quantitative and qualitative evaluation using real aerial images show the potential of our method to support environmental monitoring and surveillance applications.
更多查看译文
关键词
Image reconstruction,Semantics,Three-dimensional displays,Convolution,Solid modeling,Robots,Cameras,Aerial systems: Perception and autonomy,graph convolution for mesh reconstruction,mapping,semantic scene understanding
AI 理解论文
溯源树
样例
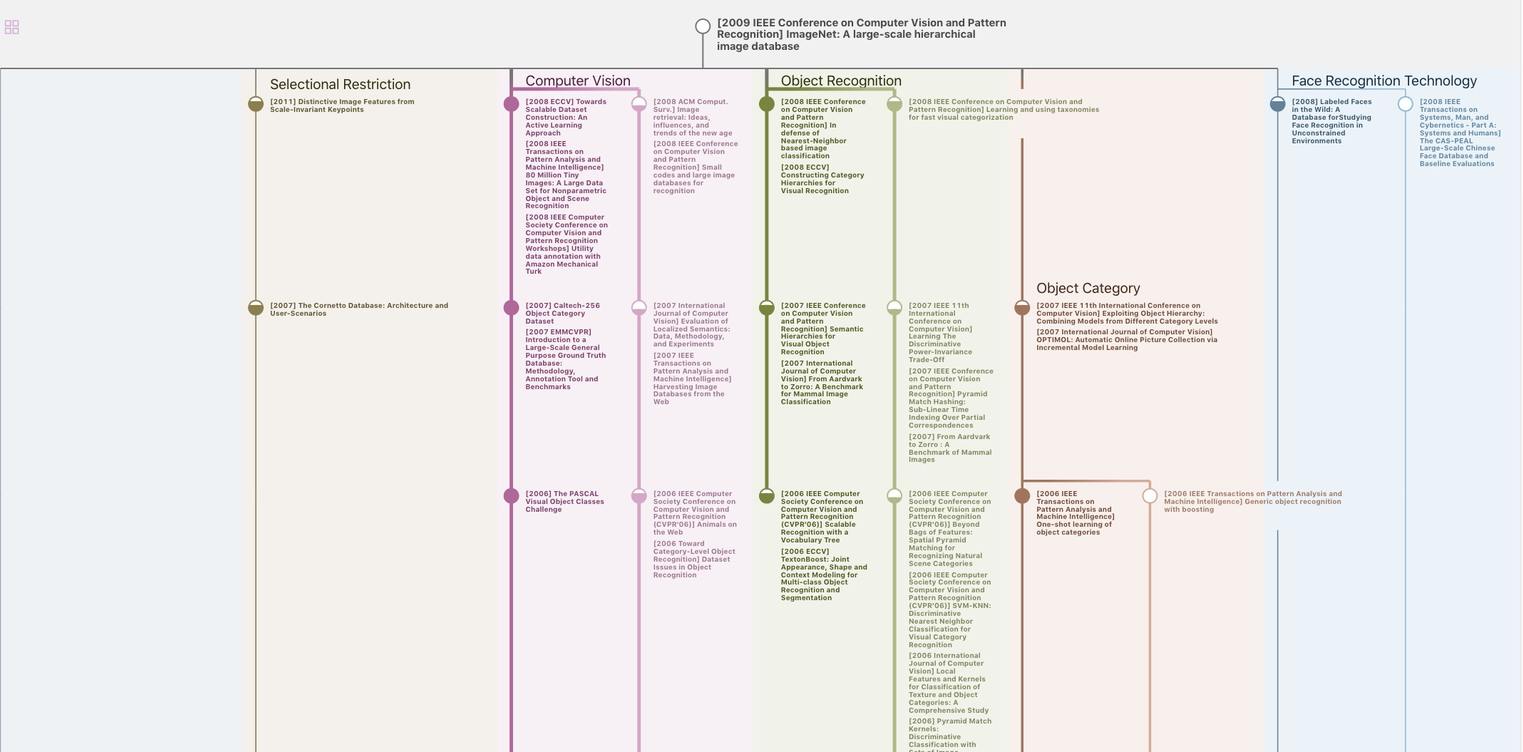
生成溯源树,研究论文发展脉络
Chat Paper
正在生成论文摘要