Privacy-preserving Multi-source Cross-domain Recommendation Based on Knowledge Graph
ACM TRANSACTIONS ON MULTIMEDIA COMPUTING COMMUNICATIONS AND APPLICATIONS(2024)
摘要
The cross-domain recommender systems aim to alleviate the data sparsity problem in the target domain by transferring knowledge from the auxiliary domain. However, existing works ignore the fact that the data sparsity problem may also exist in the single auxiliary domain, and sharing user behavior data is restricted by the privacy policy. In addition, their cross-domain models lack interpretability. To address these concerns, we propose a novel multi-source cross-domain model based on knowledge graph. Specifically, to avoid the insufficiency of single auxiliary domain, we construct a knowledge graph comprehensively leveraging items from multiple auxiliary domains. To avoid the leakage of user privacy when user information is transferred to multiple domains, we construct graph for information transfer between items to effectively avoid the propagation of users' private information between different domains. We implicitly integrate the user-item interaction by transferring the learned item embeddings. To improve the interpretability of cross-domain knowledge transfer, we propose a knowledge graph-based retrieval and fusion method to transfer knowledge derived from multiple auxiliary domains. An attention-based fusion network is designed to enhance the representation of the targeted user and items with the transferred item embedding. We perform extensive experiments on three real-world datasets, demonstrating that our model outperforms the states of the art.
更多查看译文
关键词
Cross-domain recommendation,collaborative filtering,privacy preserving,knowledge graph,implicit feedback
AI 理解论文
溯源树
样例
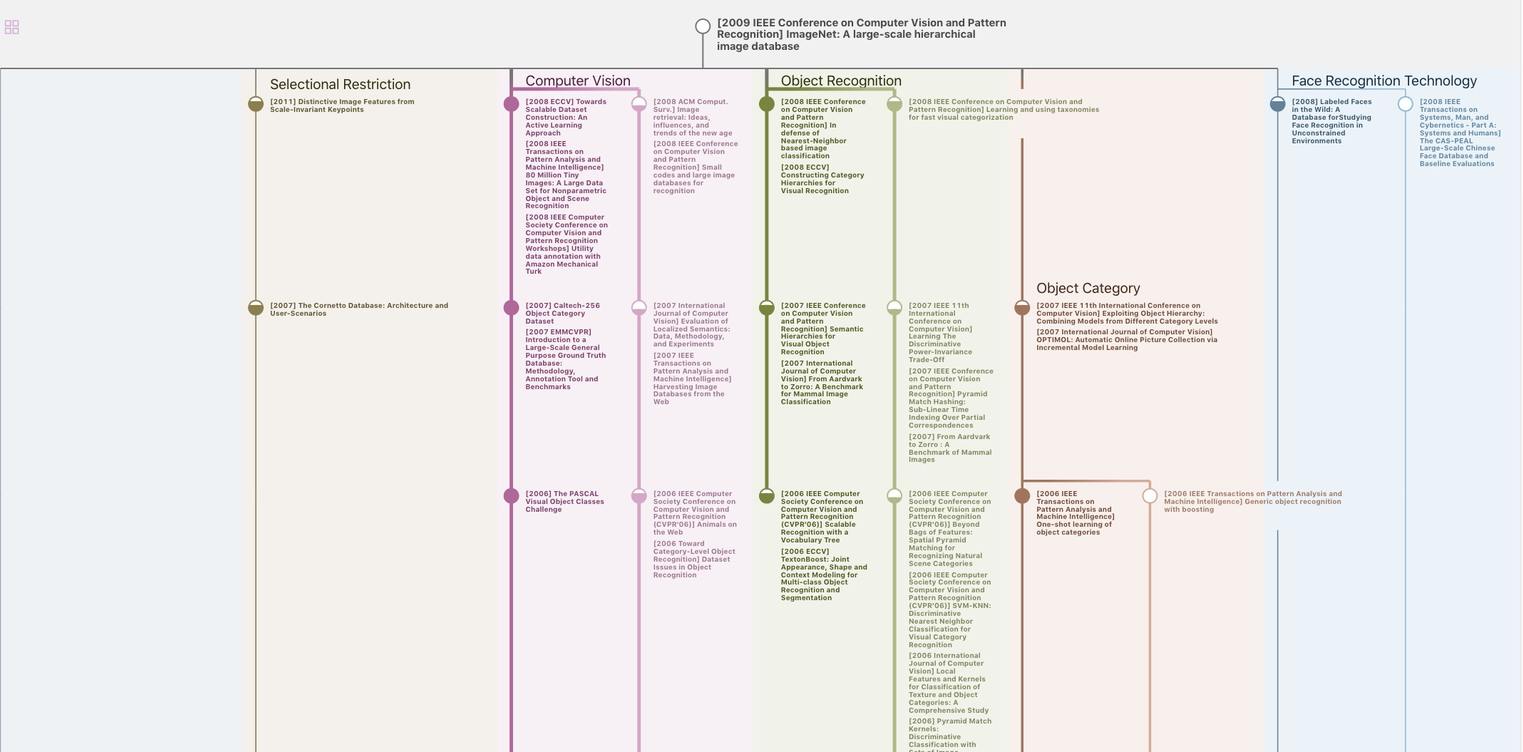
生成溯源树,研究论文发展脉络
Chat Paper
正在生成论文摘要