TS2ACT: Few-Shot Human Activity Sensing with Cross-Modal Co-Learning
PROCEEDINGS OF THE ACM ON INTERACTIVE MOBILE WEARABLE AND UBIQUITOUS TECHNOLOGIES-IMWUT(2023)
摘要
Human Activity Recognition (HAR) based on embedded sensor data has become a popular research topic in ubiquitous computing, which has a wide range of practical applications in various fields such as human-computer interaction, healthcare, and motion tracking. Due to the difficulties of annotating sensing data, unsupervised and semi-supervised HAR methods are extensively studied, but their performance gap to the fully-supervised methods is notable. In this paper, we proposed a novel cross-modal co-learning approach called TS2ACT to achieve few-shot HAR. It introduces a cross-modal dataset augmentation method that uses the semantic-rich label text to search for human activity images to form an augmented dataset consisting of partially-labeled time series and fully-labeled images. Then it adopts a pre-trained CLIP image encoder to jointly train with a time series encoder using contrastive learning, where the time series and images are brought closer in feature space if they belong to the same activity class. For inference, the feature extracted from the input time series is compared with the embedding of a pre-trained CLIP text encoder using prompt learning, and the best match is output as the HAR classification results. We conducted extensive experiments on four public datasets to evaluate the performance of the proposed method. The numerical results show that TS2ACT significantly outperforms the state-of-the-art HAR methods, and it achieves performance close to or better than the fully supervised methods even using as few as 1% labeled data for model training. The source codes of TS2ACT are publicly available on GitHub(1).
更多查看译文
关键词
Human activity recognition,few-shot learning,cross-modal dataset augmentation,contrastive learning,prompt learning
AI 理解论文
溯源树
样例
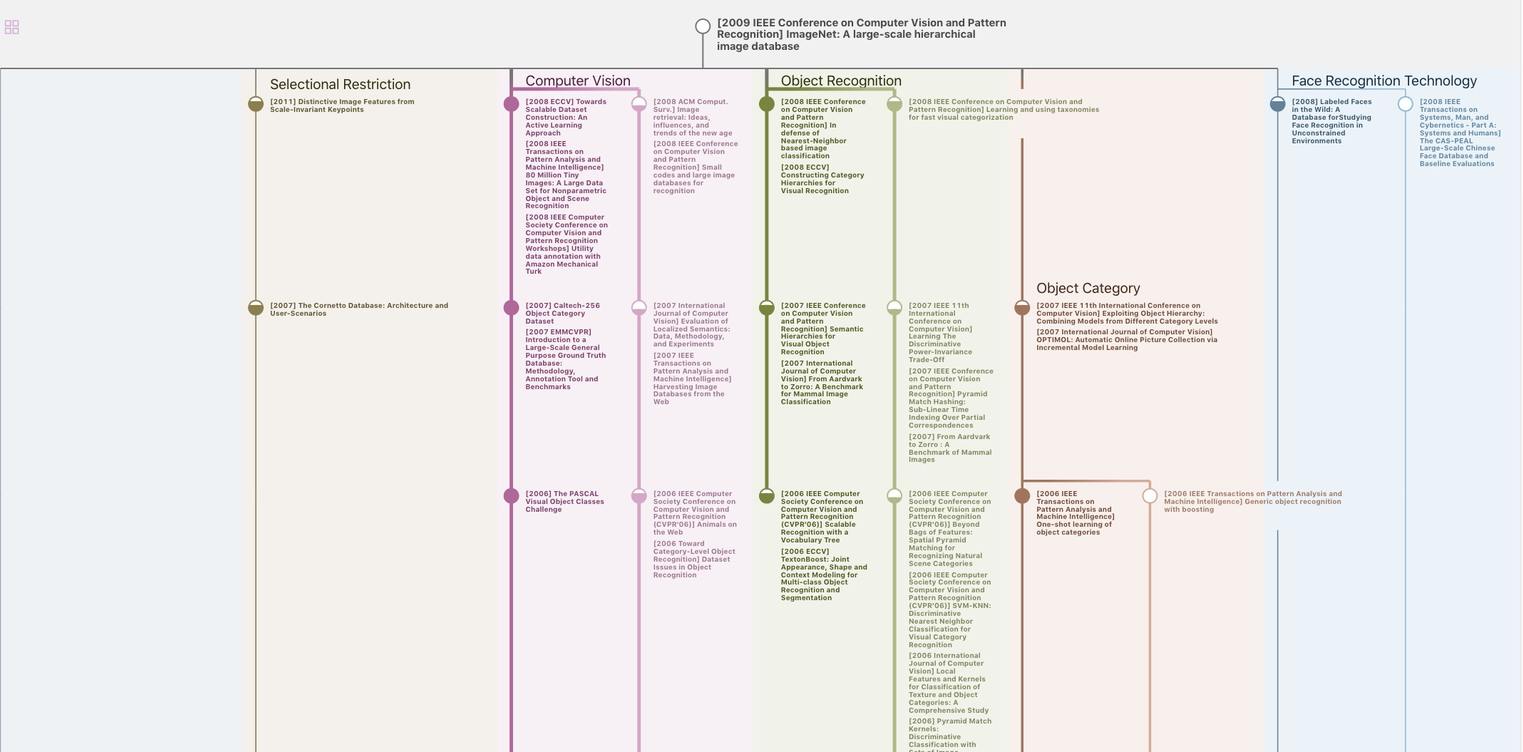
生成溯源树,研究论文发展脉络
Chat Paper
正在生成论文摘要