Domain-control prompt-driven zero-shot relational triplet extraction
NEUROCOMPUTING(2024)
摘要
Zero -shot relational triplet extraction is a vital solution to the problem of fact extracted from unstructured text without labeled training data. In the task, the data is divided into seen and unseen relations for training and prediction, respectively. A strategy that trains a generative model based on seen data first and generates training samples for unseen data has been shown to be effective in solving this task. However, this strategy is severely limited by error propagation caused by generated noisy data. To address this issue, prompts may provide a feasible route since they have been widely utilized in cross -domain tasks. In this paper, three preliminary experiments reveal the effectiveness of prompts for the task of triplet extraction and its internal mechanism. Specifically, the method using prompts can control the domain.1 Further, we propose a simple but effective model for zero -shot relational triplet extraction, which leverages zero -shot text classification to first determine the prompts of unseen relations aiming to optimize both its domain and length, and then extracts triplets via prompt -driven strategy. Extensive experiments are conducted on two public datasets, demonstrating that the proposed model achieves a better performance than baselines.
更多查看译文
关键词
Relational triplet extraction,Zero-shot learning,Prompt,Pre-trained language models
AI 理解论文
溯源树
样例
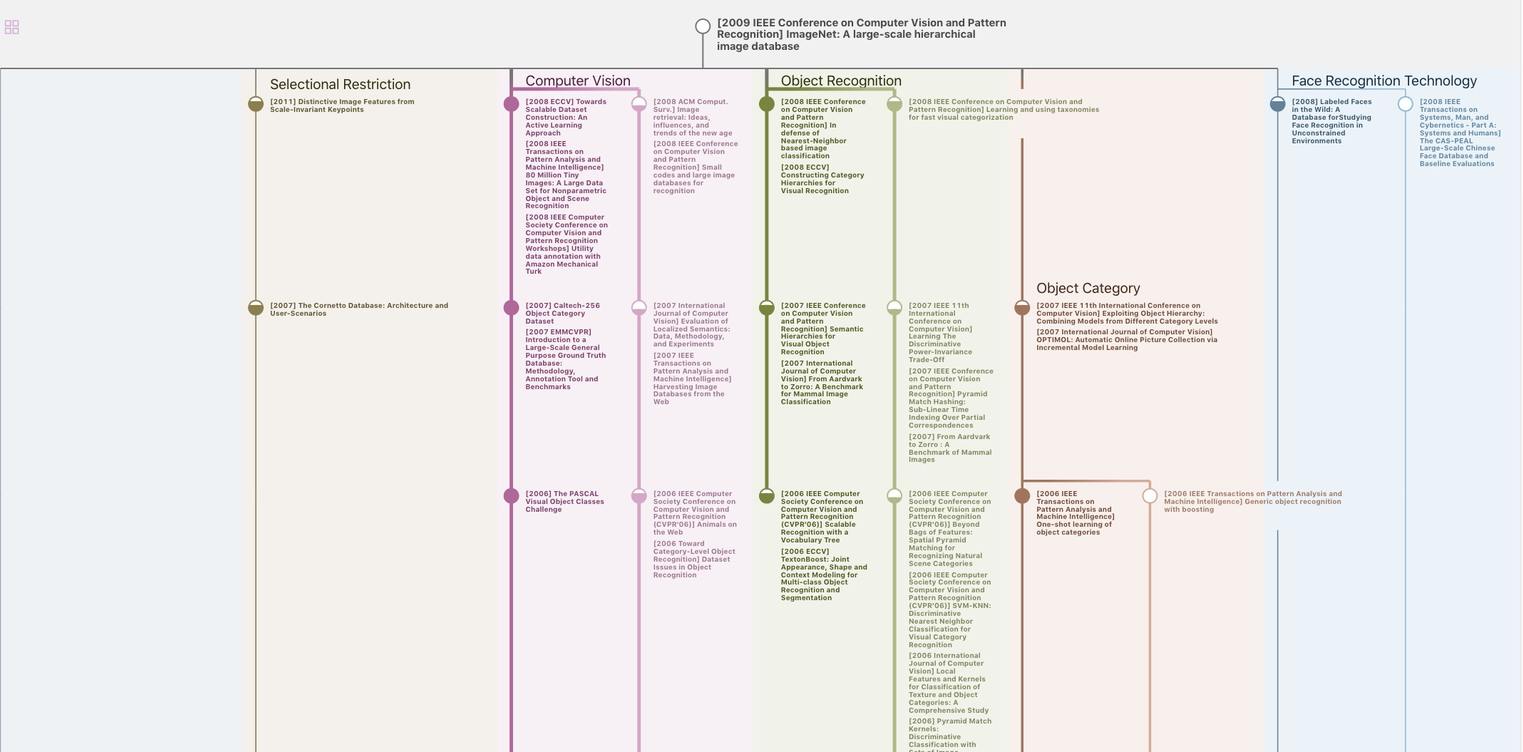
生成溯源树,研究论文发展脉络
Chat Paper
正在生成论文摘要