Unified Physical-Layer Learning Framework Toward VLC-Enabled 6G Indoor Flying Networks
IEEE INTERNET OF THINGS JOURNAL(2024)
摘要
The practical deployment of visible light communication (VLC)-enabled flying networks could be accelerated via the simultaneous services offered by mixed carrier communication (MCC). The MCC is a unified physical-layer waveform that uniquely integrates various communication streams for data/control and signaling to support localization and dimming. For such a composite waveform, the receiver must conduct successive decoding operations of the constituent streams; thus, optimum decoding of the individual streams is essential. Existing publications rely on a conventional way to design the MCC communication chain using a block-based standalone approach that provides suboptimal performance. However, a machine learning (ML) framework has the potential for better optimization of the overall MCC system's performance. This article proposes a novel end-to-end learning framework toward a unified physical-layer waveform for VLC-enabled indoor flying networks. The obtained symbol error rate (SER) performance results confirm that the proposed learning framework outperforms the existing work by ensuring perfect decoding of the control stream and detecting signals for localization. A 12 to 15-dB gain in signal-to-noise ratio (SNR) is demonstrated at a target of 10(-3) SER. The results also indicate that a wide range of dimming is supported without significantly altering SER performance. The topics of convergence, spectral efficiency, and complexity analysis of the proposed framework are discussed in detail.
更多查看译文
关键词
Channel auto-encoders,mixed-carrier communication (MCC),unmanned aerial vehicles (UAVs),visible light communication (VLC)
AI 理解论文
溯源树
样例
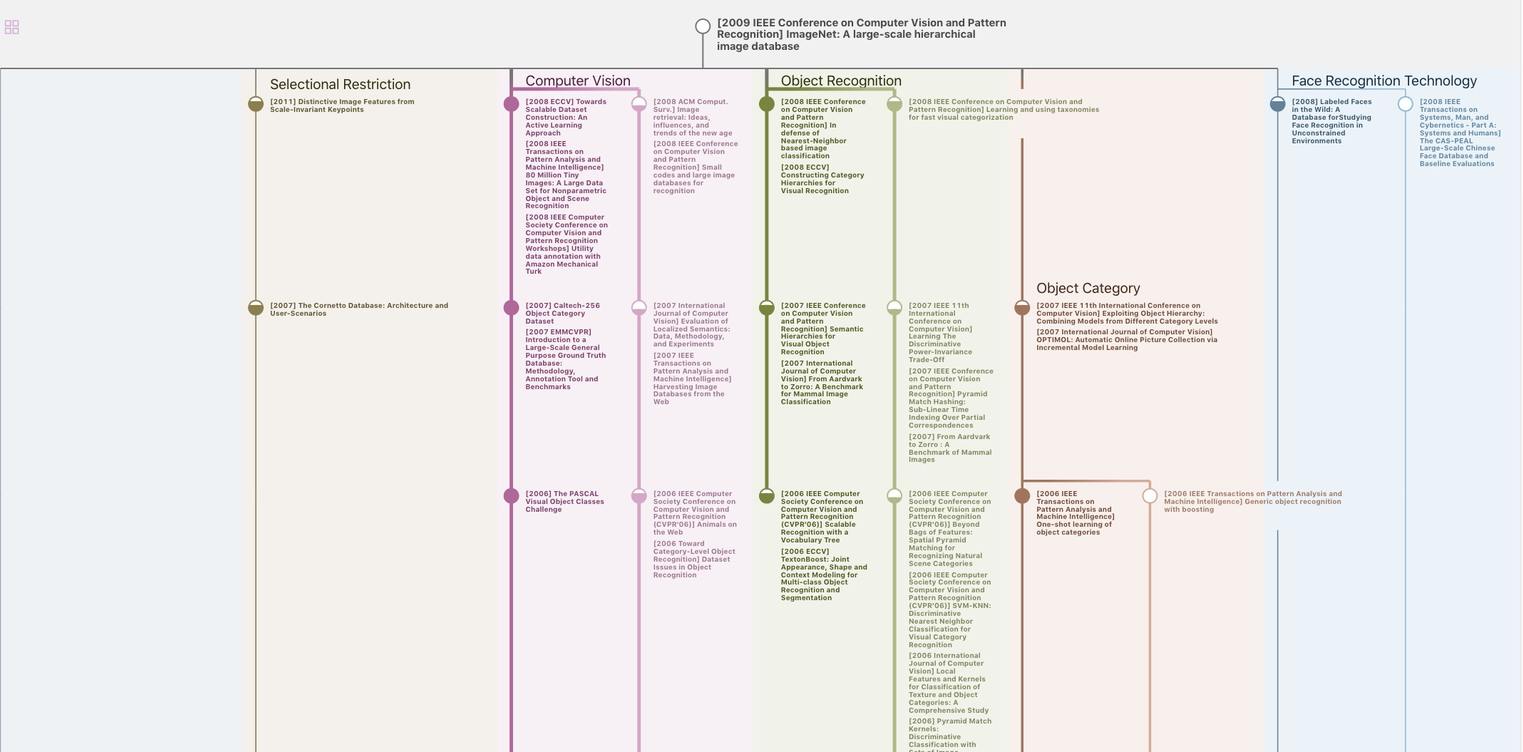
生成溯源树,研究论文发展脉络
Chat Paper
正在生成论文摘要