Learning from the Past Training Trajectories: Regularization by Validation
JOURNAL OF ADVANCED COMPUTATIONAL INTELLIGENCE AND INTELLIGENT INFORMATICS(2024)
摘要
Deep model optimization methods discard the training weights which contain information about the validation loss landscape that can guide further model optimization. In this paper, we first show that a supervisor neural network can be used to predict the validation losses or accuracy of another deep model (student) through its discarded training weights. Then based on this behavior, we propose a weight-loss (accuracy) pair-based training framework called regularization by validation to help decrease overfitting and increase the generalization performance of the student model by predicting the validation losses. We conduct our experiments on the MNIST, CIFAR-10, and CIFAR-100 datasets with the multilayer perceptron and ResNet-56 to show that we can improve the generalization performance with the past training trajectories.
更多查看译文
关键词
validation loss landscape,multilayer perceptron,overfitting,regularization,gradient descent
AI 理解论文
溯源树
样例
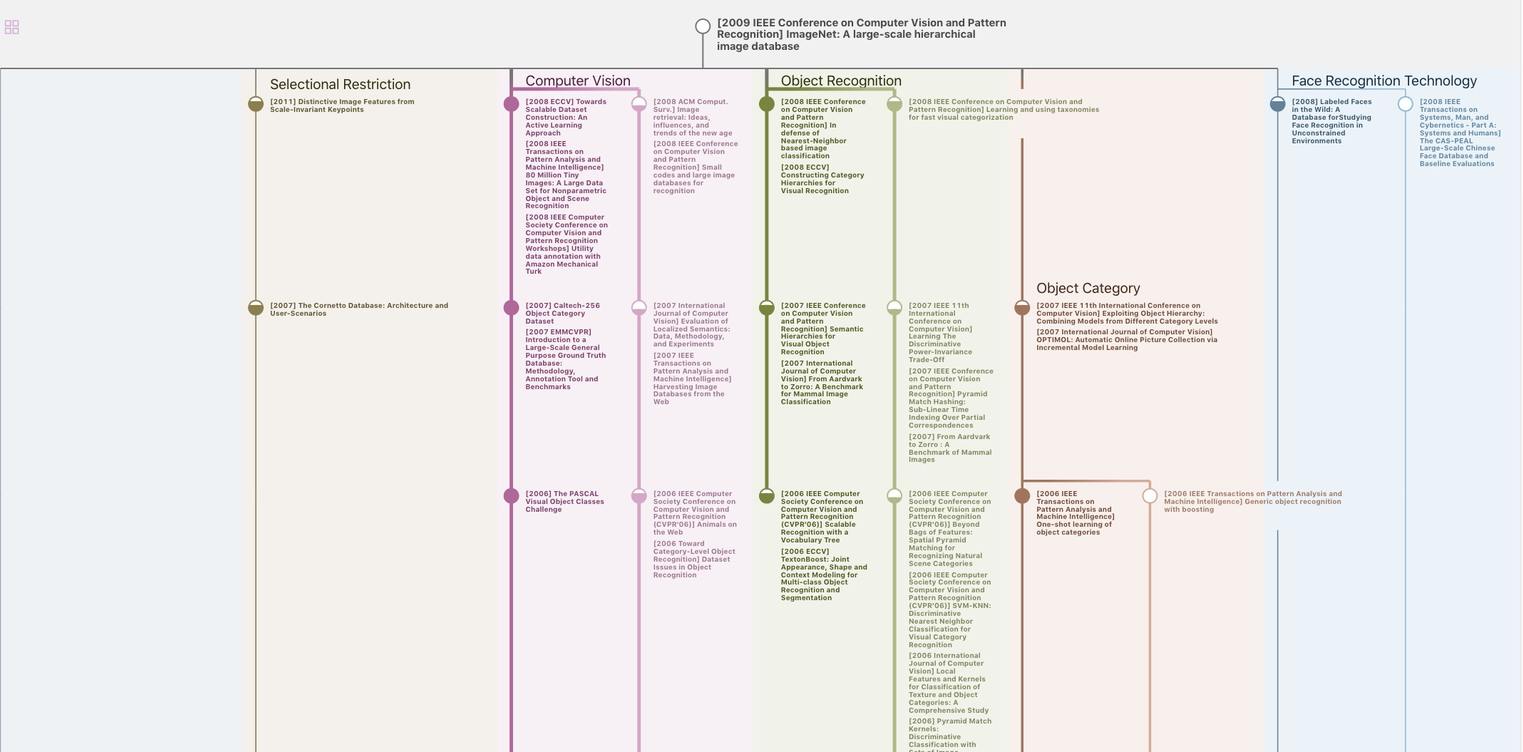
生成溯源树,研究论文发展脉络
Chat Paper
正在生成论文摘要