HierCat: Hierarchical Qery Categorization from Weakly Supervised Data at Facebook Marketplace
COMPANION OF THE WORLD WIDE WEB CONFERENCE, WWW 2023(2023)
摘要
Query categorization at customer-to-customer e-commerce platforms like Facebook Marketplace is challenging due to the vagueness of search intent, noise in real-world data, and imbalanced training data across languages. Its deployment also needs to consider challenges in scalability and downstream integration in order to translate modeling advances into better search result relevance. In this paper we present HierCat, the query categorization system at Facebook Marketplace. HierCat addresses these challenges by leveraging multi-task pre-training of dual-encoder architectures with a hierarchical inference step to efectively learn from weakly supervised training data mined from searcher engagement. We show that HierCat not only outperforms popular methods in ofine experiments, but also leads to 1.4% improvement in NDCG and 4.3% increase in searcher engagement at Facebook Marketplace Search in two weeks of online A/B testing.
更多查看译文
关键词
Query understanding,e-commerce,information retrieval
AI 理解论文
溯源树
样例
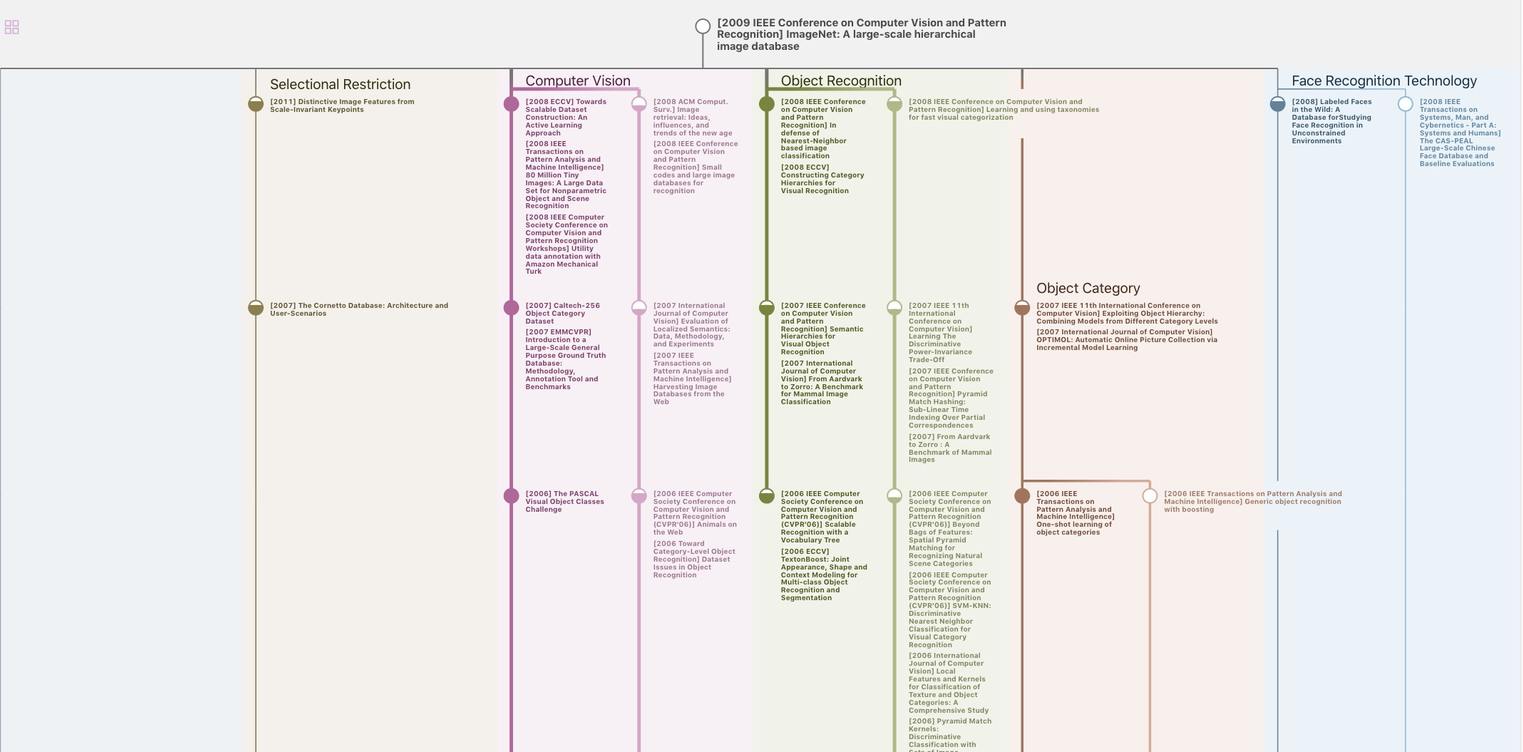
生成溯源树,研究论文发展脉络
Chat Paper
正在生成论文摘要