Weighted Knowledge Graph Embedding
PROCEEDINGS OF THE 46TH INTERNATIONAL ACM SIGIR CONFERENCE ON RESEARCH AND DEVELOPMENT IN INFORMATION RETRIEVAL, SIGIR 2023(2023)
摘要
Knowledge graph embedding (KGE) aims to project both entities and relations in a knowledge graph (KG) into low-dimensional vectors. Indeed, existing KGs suffer from the data imbalance issue, i.e., entities and relations conform to a long-tail distribution, only a small portion of entities and relations occur frequently, while the vast majority of entities and relations only have a few training samples. Existing KGE methods assign equal weights to each entity and relation during the training process. Under this setting, long-tail entities and relations are not fully trained during training, leading to unreliable representations. In this paper, we propose WeightE, which attends differentially to different entities and relations. Specifically, WeightE is able to endow lower weights to frequent entities and relations, and higher weights to infrequent ones. In such manner, WeightE is capable of increasing the weights of long-tail entities and relations, and learning better representations for them. In particular, WeightE tailors bilevel optimization for the KGE task, where the inner level aims to learn reliable entity and relation embeddings, and the outer level attempts to assign appropriate weights for each entity and relation. Moreover, it is worth noting that our technique of applying weights to different entities and relations is general and flexible, which can be applied to a number of existing KGE models. Finally, we extensively validate the superiority of WeightE against various state-of-the-art baselines.
更多查看译文
关键词
Knowledge Graph Embedding,Bilevel Optimization,Link Prediction
AI 理解论文
溯源树
样例
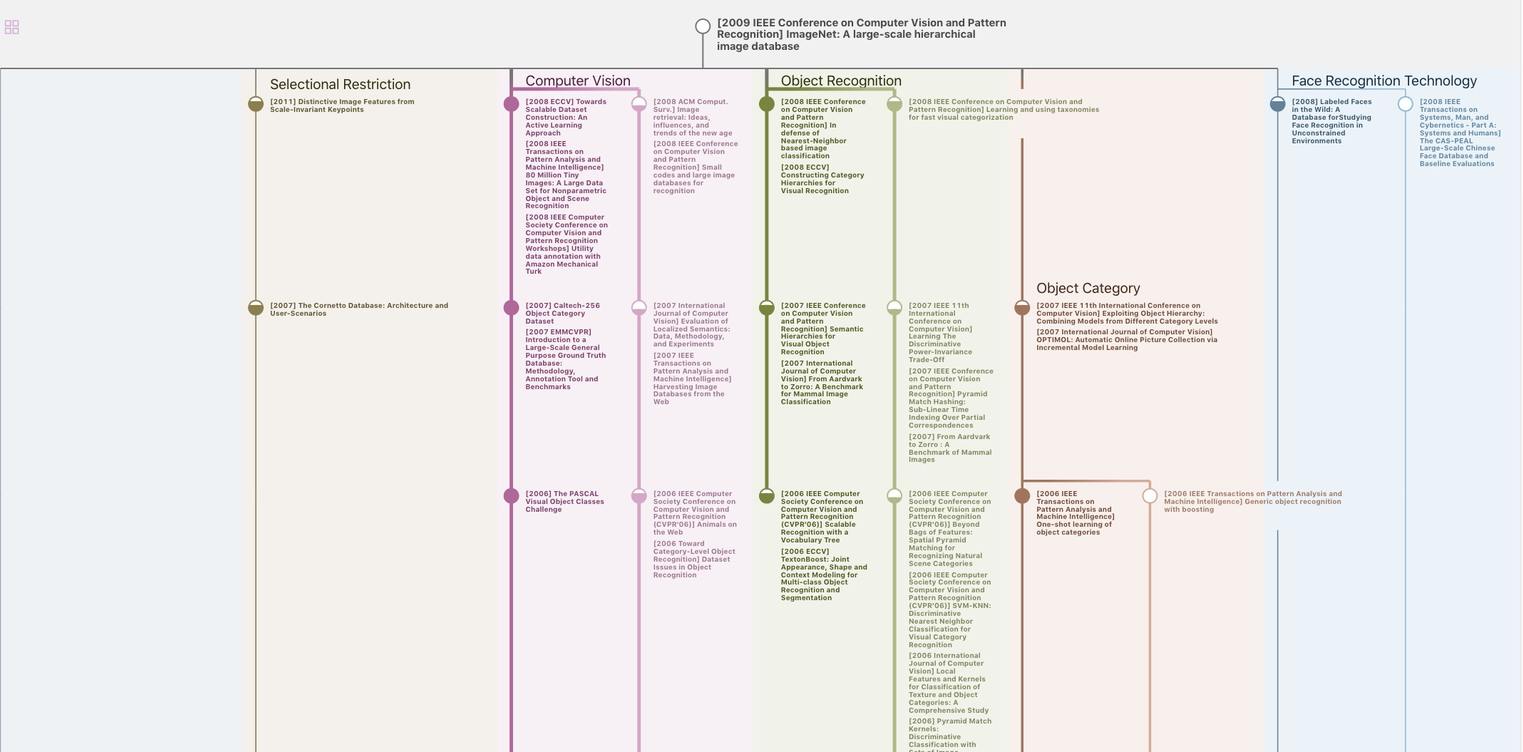
生成溯源树,研究论文发展脉络
Chat Paper
正在生成论文摘要